ConvFIFO: A Crossbar Memory PIM Architecture for ConvNets Featuring First-In-First-Out Dataflow
2024 29th Asia and South Pacific Design Automation Conference (ASP-DAC)(2024)
摘要
Process-in-memory (PIM) architectures based on emerging non-volatile memories (NVMs) have been widely studied for more efficient computation of convolutional neural networks (ConvNets). However, conventional NVM-based PIM suffered from various non-idealities including IR drop, sneak-path currents, analog-to-digital converter (ADC) overhead, device variations and mismatch. In this work, we propose ConvFIFO, a crossbar memory PIM architecture for ConvNets featuring a novel first-in-first-out (FIFO) dataflow. Through the design of FIFO-type input/output buffers, ConvFIFO can maximize the reuse rates of inputs and partial sums to achieve a more balanced trade-off among throughput, accuracy and area/energy consumption. By using SRAM-based FIFO, ConvFIFO further achieves a systolic architecture without the need to move weight data, bypassing the limitation of NVM endurance. Compared to classical NVM-based PIM architectures like ISAAC, ConvFIFO exhibits significant performance improvement in terms of energy consumption $(1.66-3.56 \times)$, latency $(1.69-1.74 \times)$, Ops/W ($4.23-10.17 \times)$ and $\mathrm{Ops}/\mathrm{s} \times \mathrm{mm}^{2} (1.59-1.74 \times)$, benchmarked against a number of common ConvNet models.
更多查看译文
关键词
Convolutional Neural Network,Crossbar Memory,Neural Network,Energy Consumption,Voltage Drop,Analog-to-digital Converter,Non-volatile Memory,Partial Sums,Balanced Trade-off,Energy Efficiency,Receptive Field,Convolution Kernel,Load Data,AlexNet,Output Feature Map,Buffer Size,Design Philosophy,Memory Elements,Crossbar Array,Memory Wall
AI 理解论文
溯源树
样例
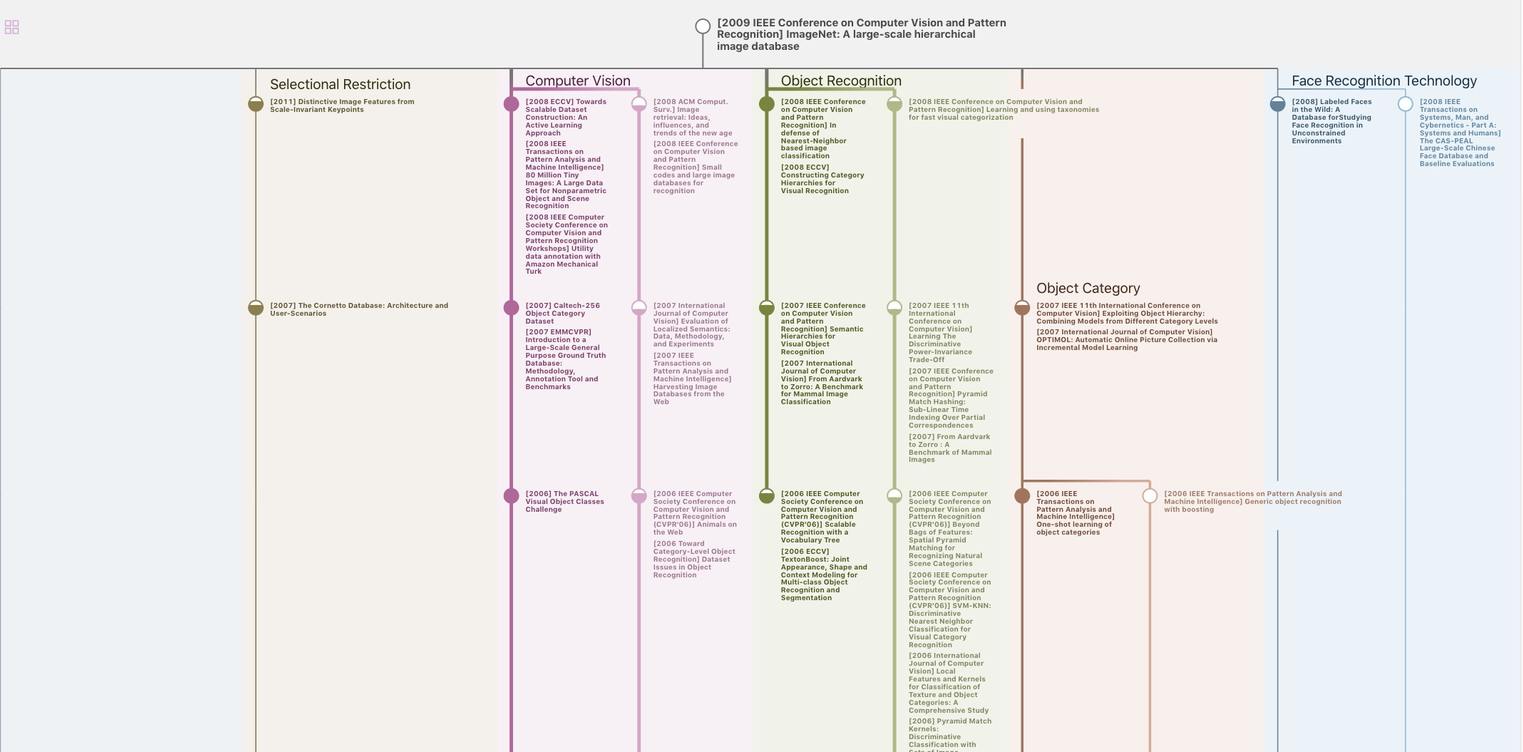
生成溯源树,研究论文发展脉络
Chat Paper
正在生成论文摘要