Early Detection of Extreme Storm Tide Events Using Multimodal Data Processing
AAAI 2024(2024)
摘要
Sea-level rise is a well-known consequence of climate change. Several studies have estimated the social and economic impact of the increase in extreme flooding. An efficient way to mitigate its consequences is the development of a flood alert and prediction system, based on high-resolution numerical models and robust sensing networks. However, current models use various simplifying assumptions that compromise accuracy to ensure solvability within a reasonable timeframe, hindering more regular and cost-effective forecasts for various locations along the shoreline. To address these issues, this work proposes a hybrid model for multimodal data processing that combines physics-based numerical simulations, data obtained from a network of sensors, and satellite images to provide refined wave and sea-surface height forecasts, with real results obtained in a critical location within the Port of Santos (the largest port in Latin America). Our approach exhibits faster convergence than data-driven models while achieving more accurate predictions. Moreover, the model handles irregularly sampled time series and missing data without the need for complex preprocessing mechanisms or data imputation while keeping low computational costs through a combination of time encoding, recurrent and graph neural networks. Enabling raw sensor data to be easily combined with existing physics-based models opens up new possibilities for accurate extreme storm tide events forecast systems that enhance community safety and aid policymakers in their decision-making processes.
更多查看译文
关键词
General
AI 理解论文
溯源树
样例
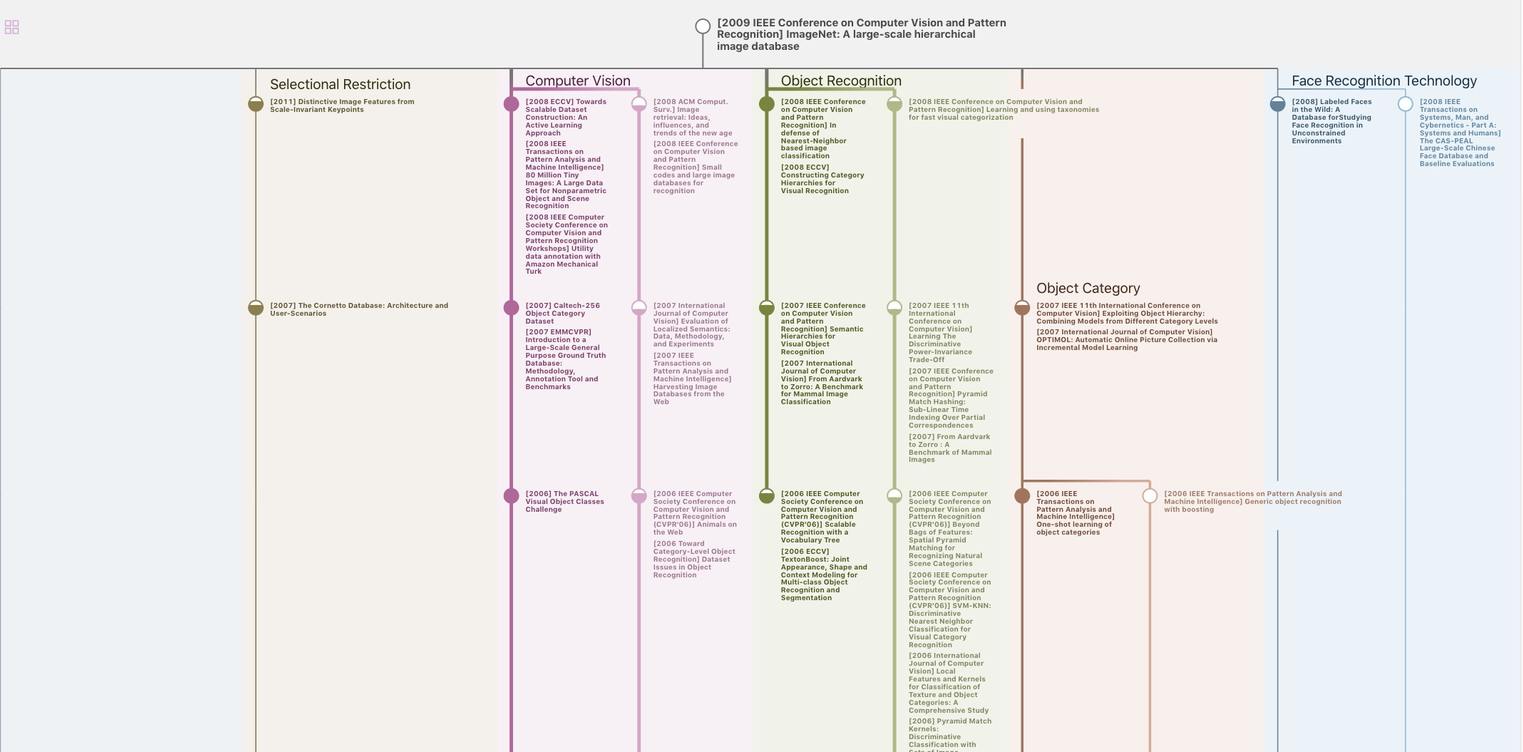
生成溯源树,研究论文发展脉络
Chat Paper
正在生成论文摘要