On the Robustness of Neural-Enhanced Video Streaming against Adversarial Attacks
AAAI 2024(2024)
摘要
The explosive growth of video traffic on today's Internet promotes the rise of Neural-enhanced Video Streaming (NeVS), which effectively improves the rate-distortion trade-off by employing a cheap neural super-resolution model for quality enhancement on the receiver side. Missing by existing work, we reveal that the NeVS pipeline may suffer from a practical threat, where the crucial codec component (i.e., encoder for compression and decoder for restoration) can trigger adversarial attacks in a man-in-the-middle manner to significantly destroy video recovery performance and finally incurs the malfunction of downstream video perception tasks. In this paper, we are the first attempt to inspect the vulnerability of NeVS and discover a novel adversarial attack, called codec hijacking, where the injected invisible perturbation conspires with the malicious encoding matrix by reorganizing the spatial-temporal bit allocation within the bitstream size budget. Such a zero-day vulnerability makes our attack hard to defend because there is no visual distortion on the recovered videos until the attack happens. More seriously, this attack can be extended to diverse enhancement models, thus exposing a wide range of video perception tasks under threat. Evaluation based on state-of-the-art video codec benchmark illustrates that our attack significantly degrades the recovery performance of NeVS over previous attack methods. The damaged video quality finally leads to obvious malfunction of downstream tasks with over 75% success rate. We hope to arouse public attention on codec hijacking and its defence.
更多查看译文
关键词
General,ML: Applications,ML: Deep Learning Algorithms,ML: Evaluation and Analysis,ML: Feature Construction/Reformulation,ML: Adversarial Learning & Robustness
AI 理解论文
溯源树
样例
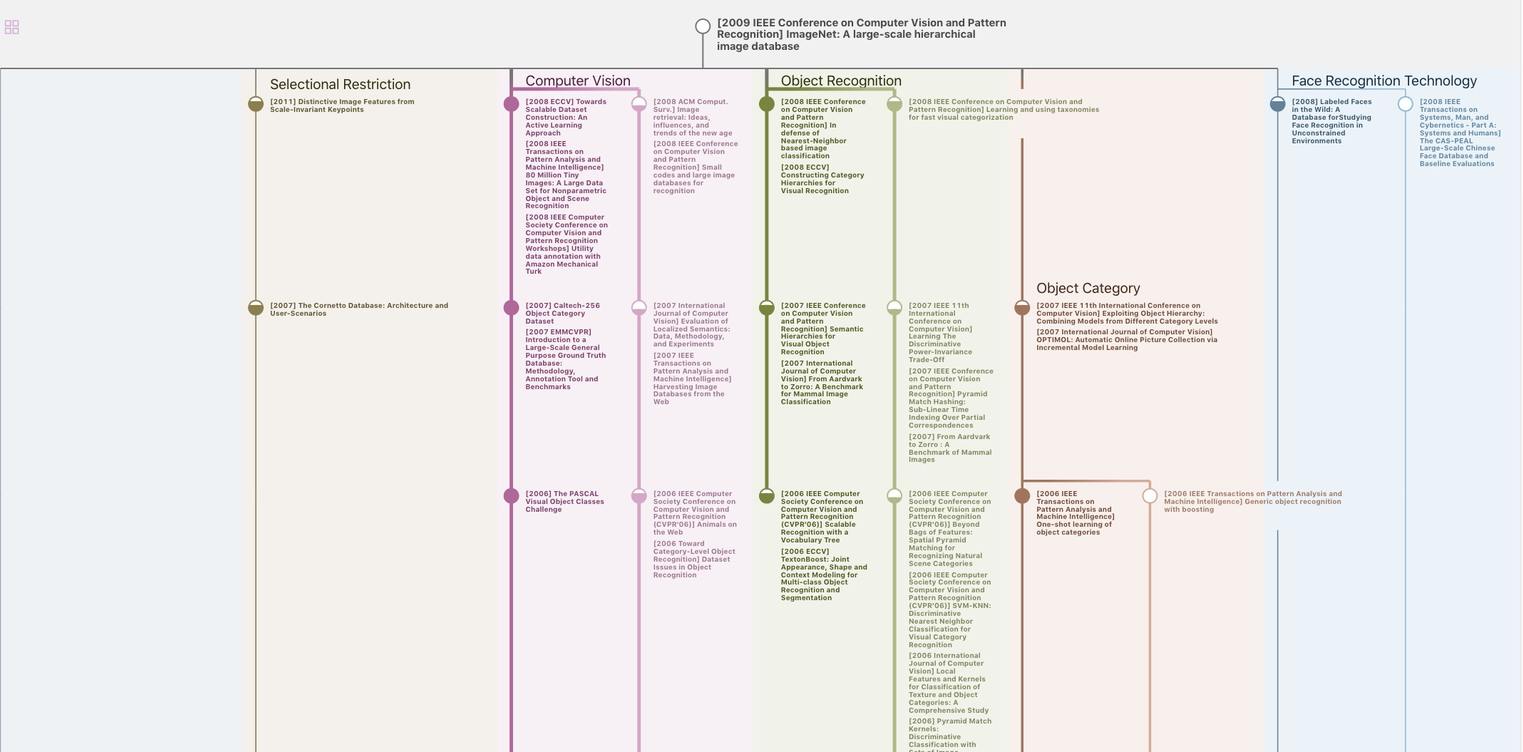
生成溯源树,研究论文发展脉络
Chat Paper
正在生成论文摘要