Personalized Trajectory Prediction for Driving Behavior Modeling in Ramp-Merging Scenarios.
International Conference on Robotic Computing(2023)
摘要
Despite numerous studies on trajectory prediction, existing approaches often fail to adequately capture the multifaceted and individual nature of driving behavior. In recognition of this gap and based on DenseTNT, an end-to-end and goal-based trajectory prediction method, our study developed a new version of DenseTNT that incorporates personalized nodes within the graph neural network in VectorNet as context encoder. Throughout the neural network computations, these nodes represent individual driver labels, allowing a more granular understanding of diverse driving behaviors to be gained. Based on comparative analysis, our model has a 11.4% reduction in minADE when compared to baseline models that do not have personalized labels.
更多查看译文
关键词
connected and automated vehicles (CAV),trajectory prediction,personalized driving behavior,ramp merging
AI 理解论文
溯源树
样例
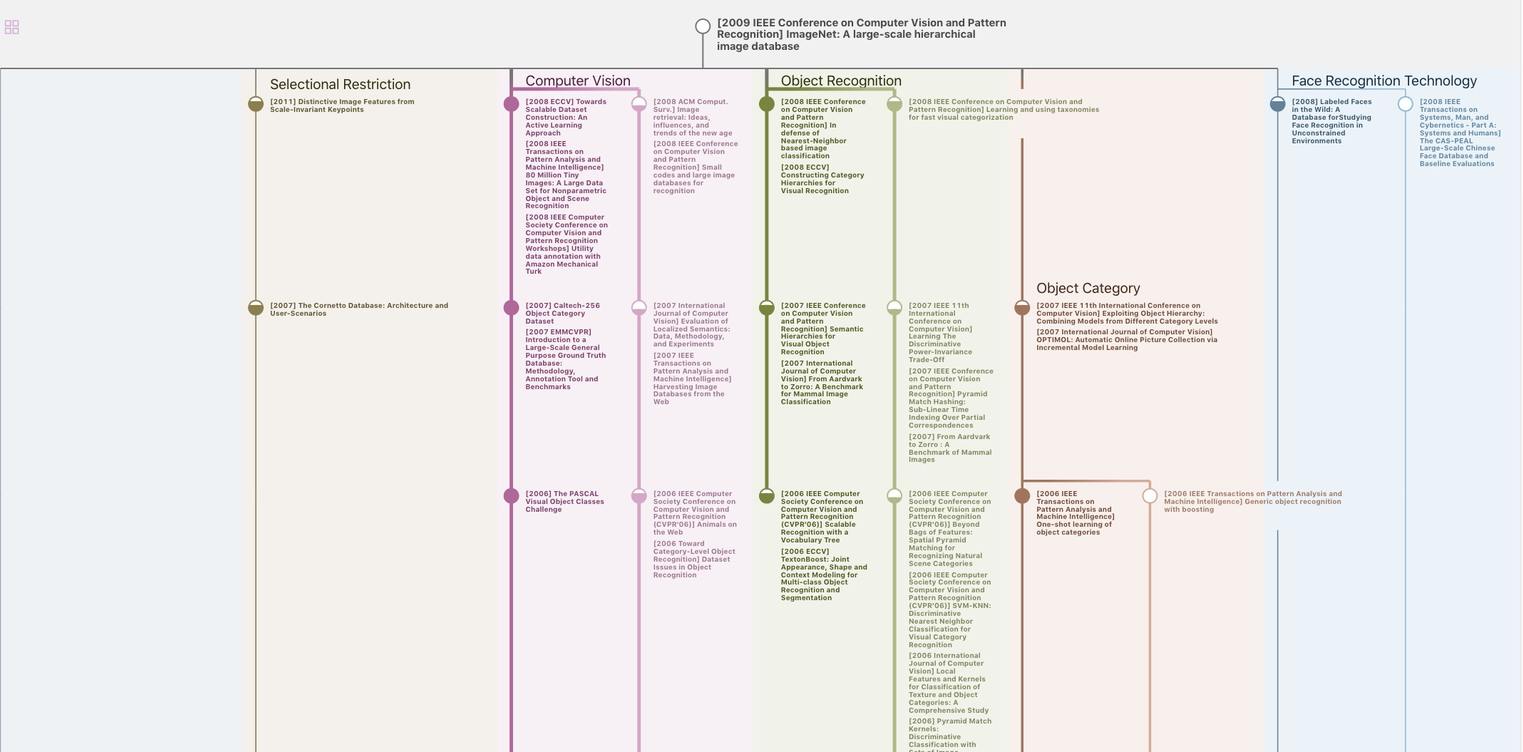
生成溯源树,研究论文发展脉络
Chat Paper
正在生成论文摘要