RG-GAN: Dynamic Regenerative Pruning for Data-Efficient Generative Adversarial Networks
AAAI 2024(2024)
摘要
Training Generative Adversarial Networks (GAN) to generate high-quality images typically requires large datasets. Network pruning during training has recently emerged as a significant advancement for data-efficient GAN. However, simple and straightforward pruning can lead to the risk of losing key information, resulting in suboptimal results due to GAN’s competitive dynamics between generator (G) and discriminator (D). Addressing this, we present RG-GAN, a novel approach that marks the first incorporation of dynamic weight regeneration and pruning in GAN training to improve the quality of the generated samples, even with limited data. Specifically, RG-GAN initiates layer-wise dynamic pruning by removing less important weights to the quality of the generated images. While pruning enhances efficiency, excessive sparsity within layers can pose a risk of model collapse. To mitigate this issue, RG-GAN applies a dynamic regeneration method to reintroduce specific weights when they become important, ensuring a balance between sparsity and image quality. Though effective, the sparse network achieved through this process might eliminate some weights important to the combined G and D performance, a crucial aspect for achieving stable and effective GAN training. RG-GAN addresses this loss of weights by integrating learned sparse network weights back into the dense network at the previous stage during a follow-up regeneration step. Our results consistently demonstrate RG-GAN’s robust performance across a variety of scenarios, including different GAN architectures, datasets, and degrees of data scarcity, reinforcing its value as a generic training methodology. Results also show that data augmentation exhibits improved performance in conjunction with RG-GAN. Furthermore, RG-GAN can achieve fewer parameters without compromising, and even enhancing, the quality of the generated samples. Code can be found at this link: https://github.com/IntellicentAI-Lab/RG-GAN
更多查看译文
关键词
CV: Learning & Optimization for CV,CV: Computational Photography, Image & Video Synthesis
AI 理解论文
溯源树
样例
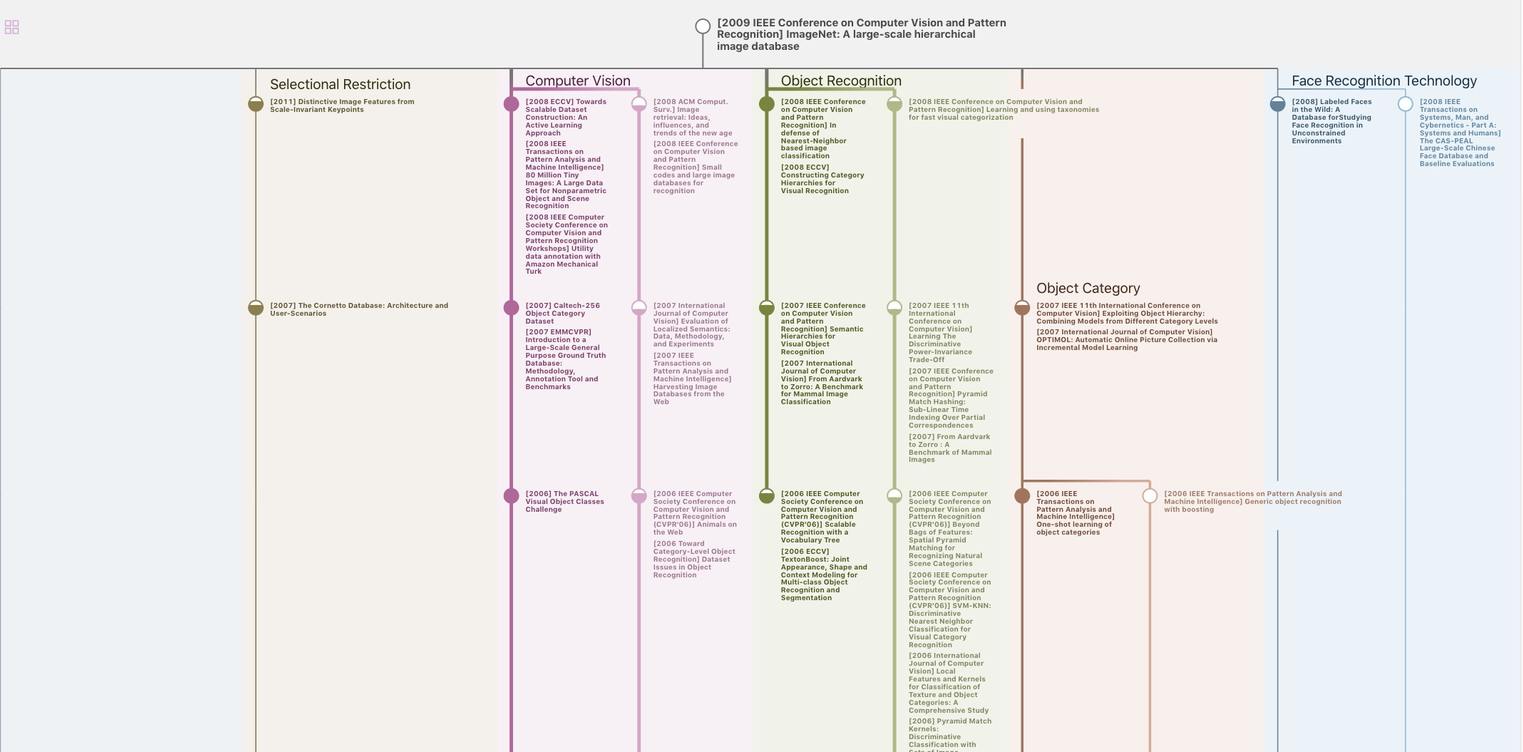
生成溯源树,研究论文发展脉络
Chat Paper
正在生成论文摘要