Towards Reliable Learning in the Wild: Generalization and Adaptation
AAAI 2024(2024)
摘要
The real-world deployment of machine learning algorithms often poses challenges due to shifts in data distributions and tasks. These shifts can lead to a degradation in model performance, as the model may not have encountered such changes during training. Additionally, they can make it difficult for the model to generalize to new scenarios and can result in poor performance in real-world applications. In this talk, I will present our research on building machine learning models that are highly generalizable and easily adaptable to different shifts. Specifically, I will first discuss our approach to improving out-of-distribution robustness and mitigating spurious correlations by training environment-invariant models through selective augmentation and post-hoc rectification. Second, I will present our techniques for continuous and rapid adaptation of models to new tasks and environments. This includes methods to facilitate compositional generalization and adaptation by extracting relationships from historical observations and to enhance reliable adaptation even in the face of imperfect observations. Additionally, I will showcase our successful practices for addressing shifts in real-world applications, such as in the healthcare, e-commerce, and transportation industries. The talk will also touch upon the remaining challenges and outline future research directions in this area.
更多查看译文
关键词
Reliable Machine Learning,Distribution Shift,Generalization
AI 理解论文
溯源树
样例
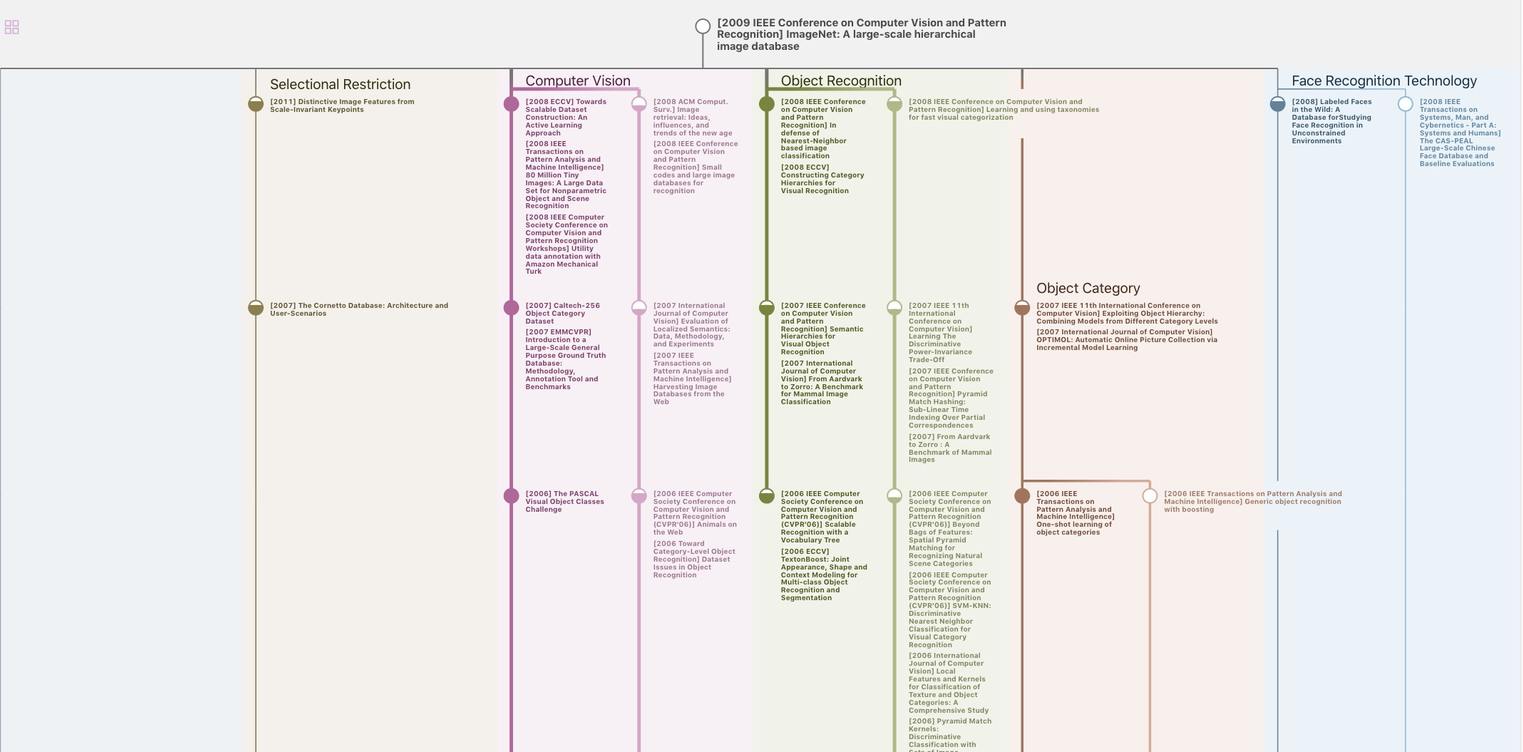
生成溯源树,研究论文发展脉络
Chat Paper
正在生成论文摘要