Self-Interpretable Graph Learning with Sufficient and Necessary Explanations
AAAI 2024(2024)
摘要
Self-interpretable graph learning methods provide insights to unveil the black-box nature of GNNs by providing predictions with built-in explanations. However, current works suffer from performance degradation compared to GNNs trained without built-in explanations. We argue the main reason is that they fail to generate explanations satisfying both sufficiency and necessity, and the biased explanations further hurt GNNs' performance. In this work, we propose a novel framework for generating SUfficient aNd NecessarY explanations (SUNNY-GNN for short) that benefit GNNs' predictions. The key idea is to conduct augmentations by structurally perturbing given explanations and employ a contrastive loss to guide the learning of explanations toward sufficiency and necessity directions. SUNNY-GNN introduces two coefficients to generate hard and reliable contrastive samples. We further extend SUNNY-GNN to heterogeneous graphs. Empirical results on various GNNs and real-world graphs show that SUNNY-GNN yields accurate predictions and faithful explanations, outperforming the state-of-the-art methods by improving 3.5% prediction accuracy and 13.1% explainability fidelity on average. Our code and data are available at https://github.com/SJTU-Quant/SUNNY-GNN.
更多查看译文
关键词
ML: Transparent, Interpretable, Explainable ML,ML: Graph-based Machine Learning
AI 理解论文
溯源树
样例
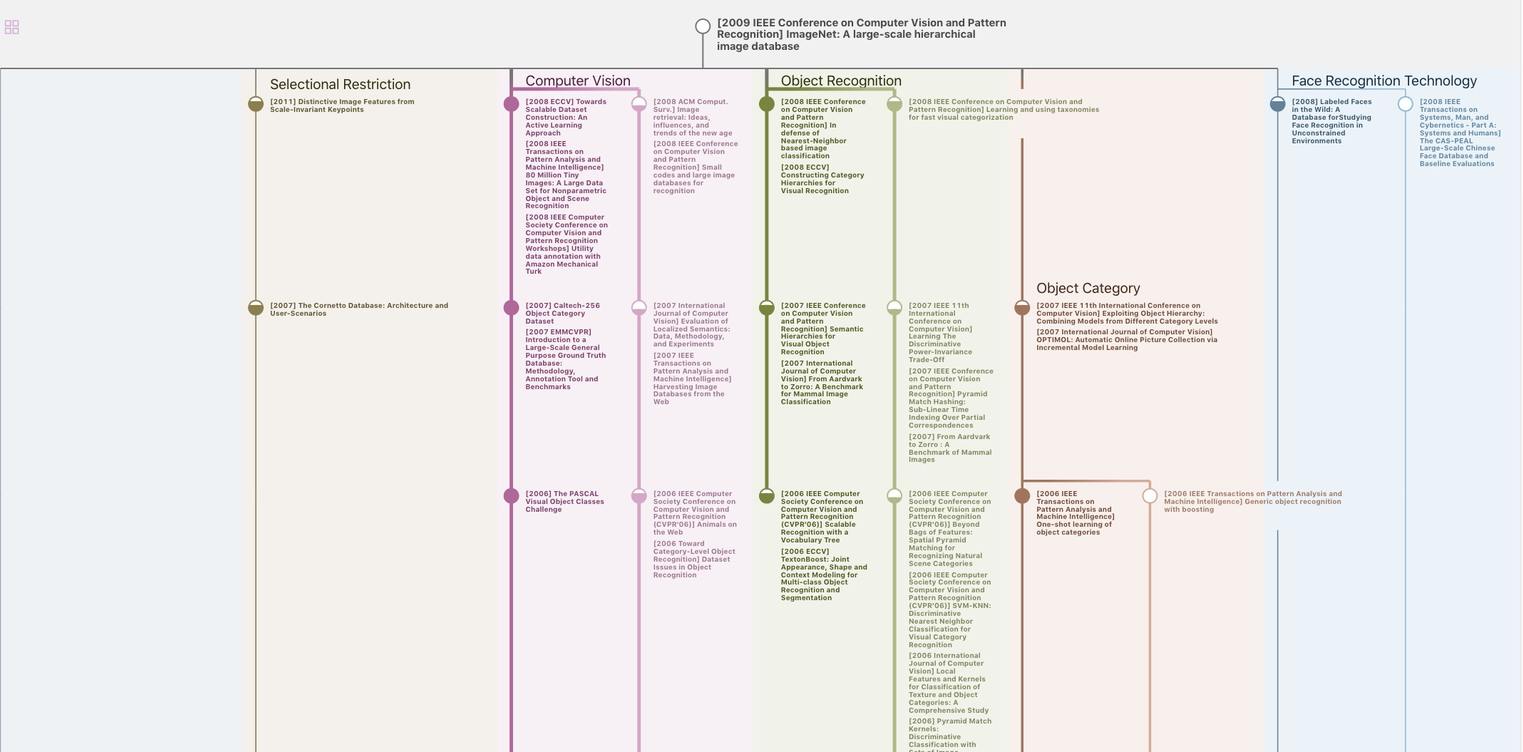
生成溯源树,研究论文发展脉络
Chat Paper
正在生成论文摘要