Partial Multi-View Clustering via Self-Supervised Network
AAAI 2024(2024)
摘要
Partial multi-view clustering is a challenging and practical research problem for data analysis in real-world applications, due to the potential data missing issue in different views. However, most existing methods have not fully explored the correlation information among various incomplete views. In addition, these existing clustering methods always ignore discovering discriminative features inside the data itself in this unsupervised task. To tackle these challenges, we propose Partial Multi-View Clustering via Self-Supervised \textbf{N}etwork (PVC-SSN) in this paper.
Specifically, we employ contrastive learning to obtain a more discriminative and consistent subspace representation, which is guided by a self-supervised module. Self-supervised learning can exploit effective cluster information through the data itself to guide the learning process of clustering tasks. Thus, it can pull together embedding features from the same cluster and push apart these from different clusters. Extensive experiments on several benchmark datasets show that the proposed PVC-SCN method outperforms several state-of-the-art clustering methods.
更多查看译文
关键词
ML: Multi-instance/Multi-view Learning,ML: Clustering,ML: Deep Learning Algorithms,ML: Deep Learning Theory,ML: Multi-class/Multi-label Learning & Extreme Classification,ML: Multimodal Learning,ML: Representation Learning
AI 理解论文
溯源树
样例
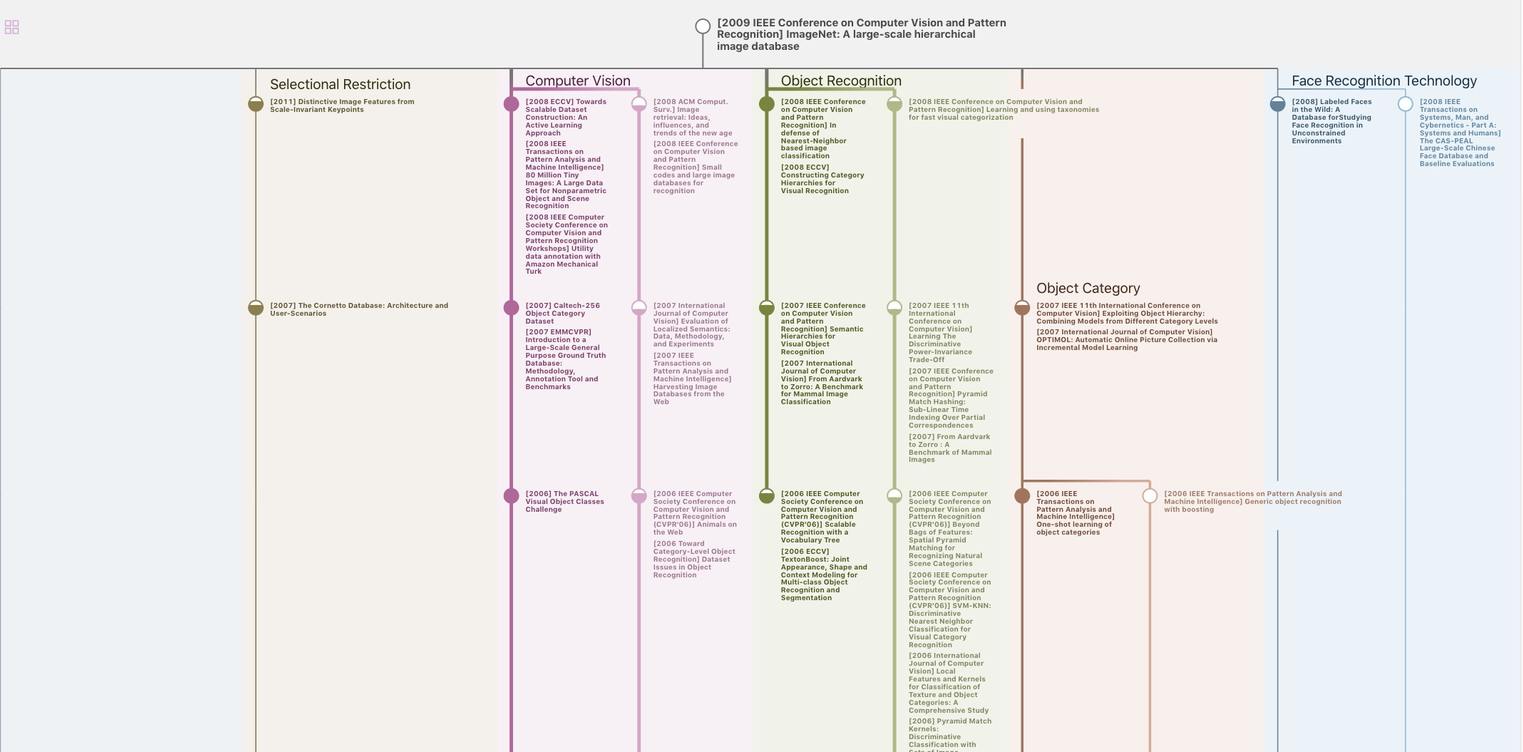
生成溯源树,研究论文发展脉络
Chat Paper
正在生成论文摘要