Fairness with Censorship: Bridging the Gap between Fairness Research and Real-World Deployment
AAAI 2024(2024)
摘要
Recent works in artificial intelligence fairness attempt to mitigate discrimination by proposing constrained optimization programs that achieve parity for some fairness statistics. Most assume the availability of class label which is impractical in many real-world applications such as precision medicine, actuarial analysis and recidivism prediction. To this end, this talk revisits fairness and reveals idiosyncrasies of existing fairness literature assuming the availability of class label that limits their real-world utility. The primary artifacts are formulating fairness with censorship to account for scenarios where the class label is not guaranteed, and a suite of corresponding new fairness notions, algorithms, and theoretical constructs to bridge the gap between the design of a ``fair'' model in the lab and its deployment in the real-world.
更多查看译文
关键词
Fairness,Censorship,Real-world Deployment
AI 理解论文
溯源树
样例
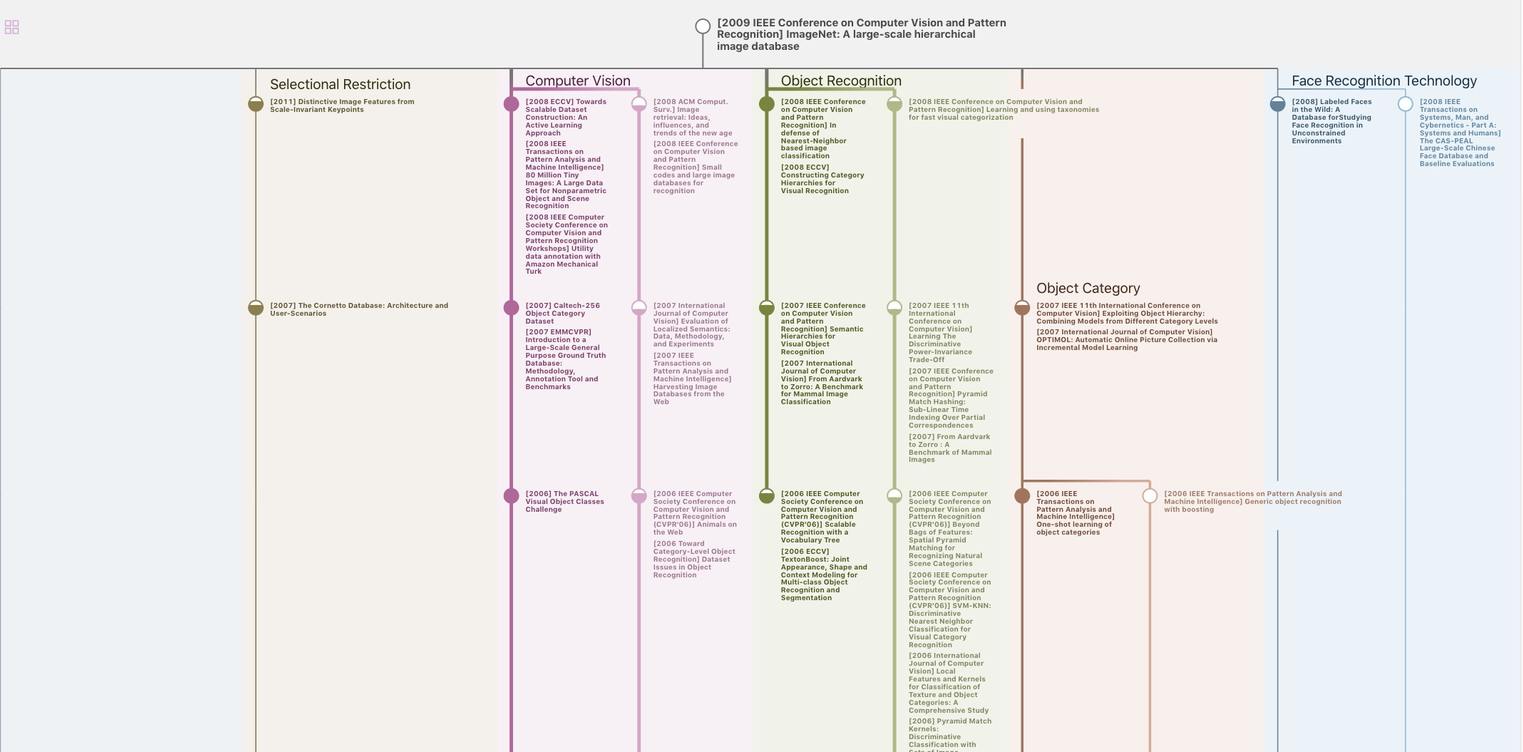
生成溯源树,研究论文发展脉络
Chat Paper
正在生成论文摘要