A Multimodal Multi-Objective Evolutionary Algorithm for Filter Feature Selection in Multi-Label Classification
IEEE Transactions on Artificial Intelligence(2024)
摘要
Multi-label learning is an emergent topic that addresses the challenge of associating multiple labels with a single instance simultaneously. Multi-label datasets often exhibit high dimensionality with noisy, irrelevant, and redundant features. In recent years, multi-label feature selection (MLFS) has gained prominence as a crucial and emerging machine learning task due to its ability to handle such data effectively. However, existing approaches for MLFS often prioritize top-ranked features based on intrinsic data criteria, disregarding relationships within the feature subset. Additionally, compared with conventional feature selection, multi-objective evolutionary algorithms (MOEAs) have not been widely explored in the context of MLFS. This study aims to address these gaps by proposing a multimodal multi-objective evolutionary algorithm (MMOEA) called MMDE_SICD which incorporates a pre-elimination scheme, an improved initialization scheme, an exploration scheme inspired by genetic operations and a statistically inspired crowding distance scheme. The results show that the proposed MMDE_SICD algorithm can outperform a variety of MOEAs and MMOEAs as well as conventional MLFS algorithms. Notably, this study is the first of its kind to consider MLFS as a multimodal multi-objective problem.
更多查看译文
关键词
Multi-Label Feature Selection,Multimodal Multi-Objective Optimization,Differential Evolution,Multi-Label Classification
AI 理解论文
溯源树
样例
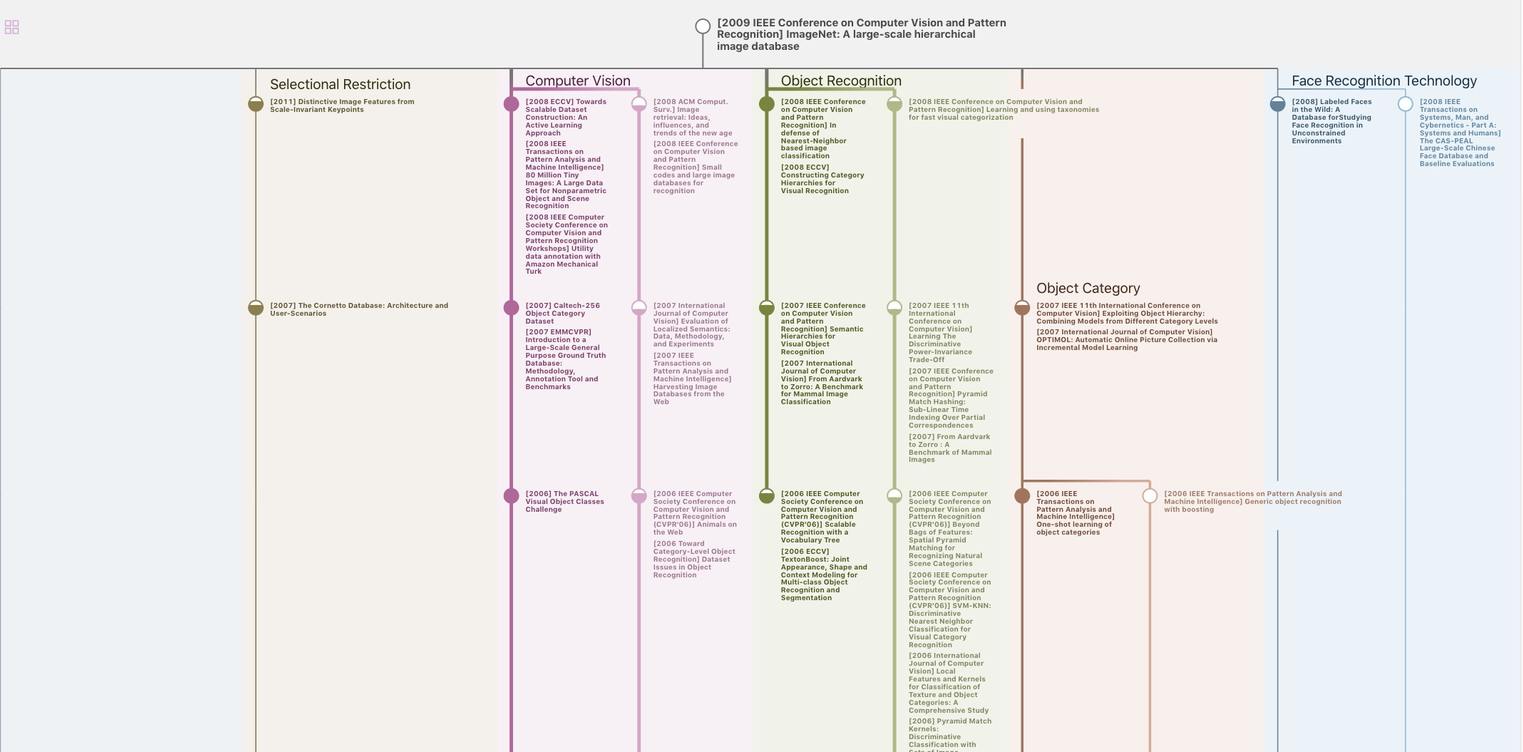
生成溯源树,研究论文发展脉络
Chat Paper
正在生成论文摘要