PVALane: Prior-Guided 3D Lane Detection with View-Agnostic Feature Alignment
AAAI 2024(2024)
摘要
Monocular 3D lane detection is essential for a reliable autonomous driving system and has recently been rapidly developing. Existing popular methods mainly employ a predefined 3D anchor for lane detection based on front-viewed (FV) space, aiming to mitigate the effects of view transformations. However, the perspective geometric distortion between FV and 3D space in this FV-based approach introduces extremely dense anchor designs, which ultimately leads to confusing lane representations. In this paper, we introduce a novel prior-guided perspective on lane detection and propose an end-to-end framework named PVALane, which utilizes 2D prior knowledge to achieve precise and efficient 3D lane detection. Since 2D lane predictions can provide strong priors for lane existence, PVALane exploits FV features to generate sparse prior anchors with potential lanes in 2D space. These dynamic prior anchors help PVALane to achieve distinct lane representations and effectively improve the precision of PVALane due to the reduced lane search space. Additionally, by leveraging these prior anchors and representing lanes in both FV and bird-eye-viewed (BEV) spaces, we effectively align and merge semantic and geometric information from FV and BEV features. Extensive experiments conducted on the OpenLane and ONCE-3DLanes datasets demonstrate the superior performance of our method compared to existing state-of-the-art approaches and exhibit excellent robustness.
更多查看译文
关键词
CV: Vision for Robotics & Autonomous Driving
AI 理解论文
溯源树
样例
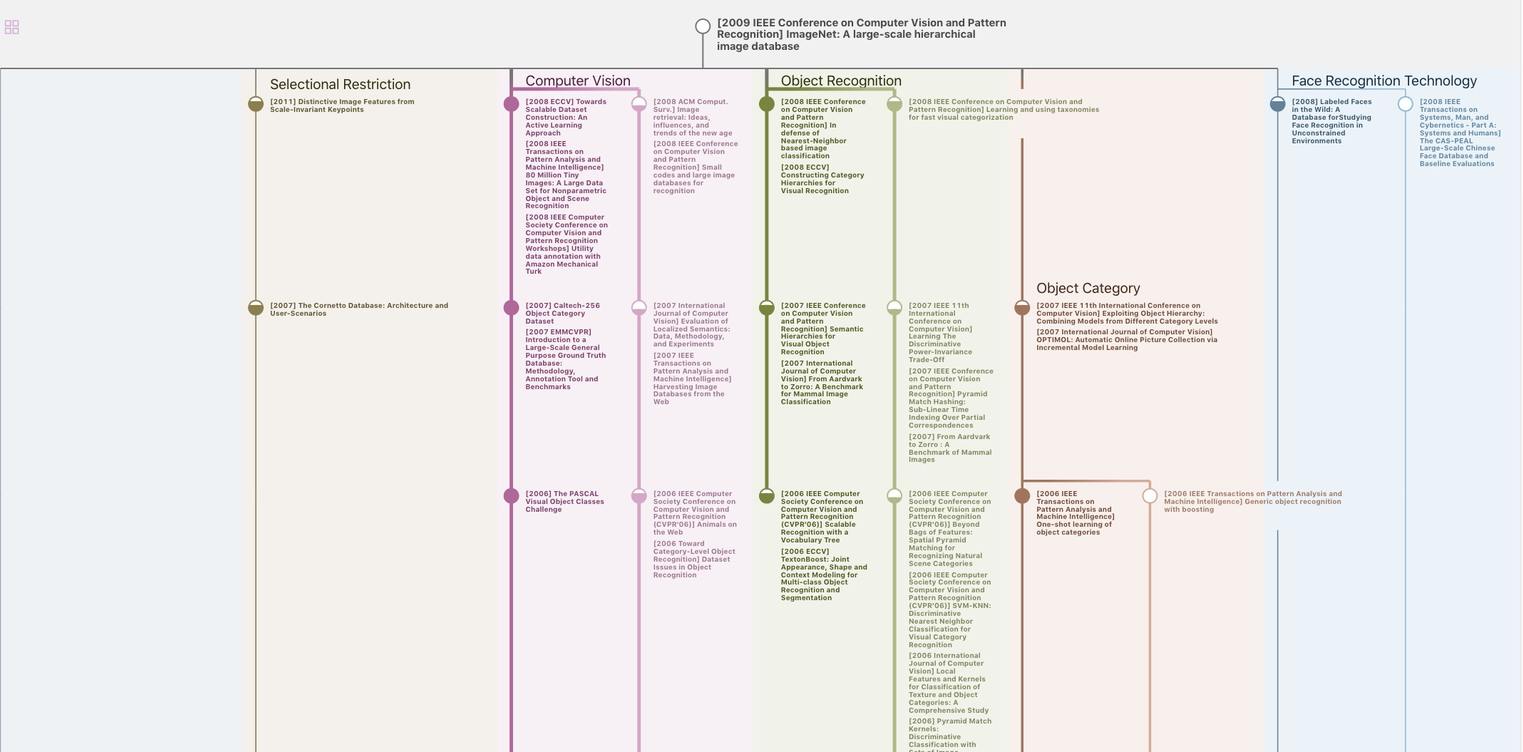
生成溯源树,研究论文发展脉络
Chat Paper
正在生成论文摘要