Long-Tailed Partial Label Learning by Head Classifier and Tail Classifier Cooperation
AAAI 2024(2024)
摘要
In partial label learning (PLL), each instance is associated with a set of candidate labels, among which only one is correct. The traditional PLL almost all implicitly assume that the distribution of the classes is balanced. However, in real-world applications, the distribution of the classes is imbalanced or long-tailed, leading to the long-tailed partial label learning problem. The previous methods solve this problem mainly by ameliorating the ability to learn in the tail classes, which will sacrifice the performance of the head classes. While keeping the performance of the head classes may degrade the performance of the tail classes. Therefore, in this paper, we construct two classifiers, i.e., a head classifier for keeping the performance of dominant classes and a tail classifier for improving the performance of the tail classes. Then, we propose a classifier weight estimation module to automatically estimate the shot belongingness (head class or tail class) of the samples and allocate the weights for the head classifier and tail classifier when making prediction. This cooperation improves the prediction ability for both the head classes and the tail classes. The experiments on the benchmarks demonstrate the proposed approach improves the accuracy of the SOTA methods by a substantial margin. Code and data are available at: https://github.com/pruirui/HTC-LTPLL.
更多查看译文
关键词
ML: Multi-class/Multi-label Learning & Extreme Classification,ML: Semi-Supervised Learning
AI 理解论文
溯源树
样例
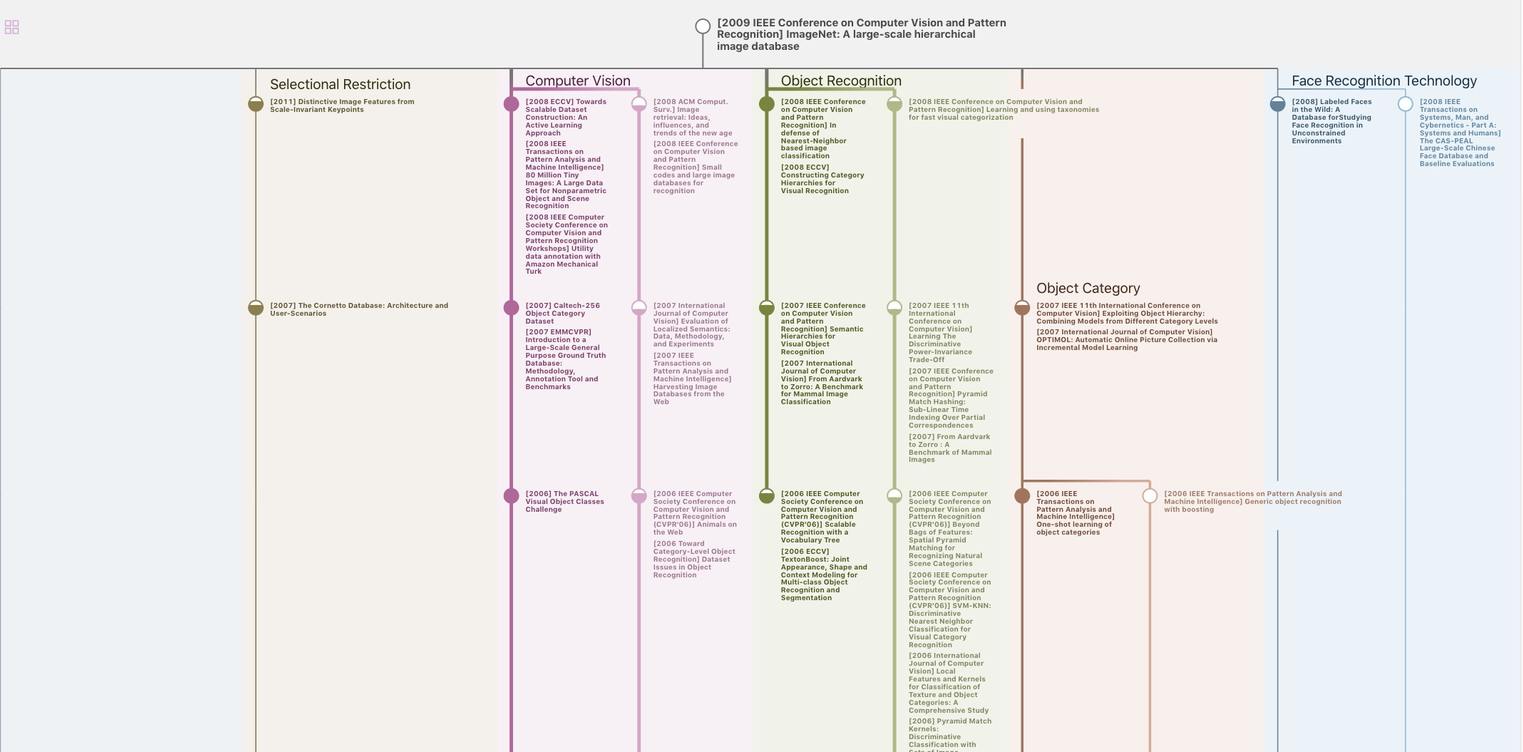
生成溯源树,研究论文发展脉络
Chat Paper
正在生成论文摘要