Bi-objective Optimization in Role Mining
arxiv(2024)
摘要
Role mining is a technique used to derive a role-based authorization policy
from an existing policy. Given a set of users U, a set of permissions P and
a user-permission authorization relation UPA⊆ U× P, a
role mining algorithm seeks to compute a set of roles R, a user-role
authorization relation 𝑈𝐴⊆ U× R and a permission-role
authorization relation 𝑃𝐴⊆ R× P, such that the
composition of 𝑈𝐴 and 𝑃𝐴 is close (in some appropriate
sense) to 𝑈𝑃𝐴.
In this paper, we first introduce the Generalized Noise Role Mining problem
(GNRM) – a generalization of the MinNoise Role Mining problem – which we
believe has considerable practical relevance. Extending work of Fomin et al.,
we show that GNRM is fixed parameter tractable, with parameter r + k, where
r is the number of roles in the solution and k is the number of
discrepancies between 𝑈𝑃𝐴 and the relation defined by the
composition of 𝑈𝐴 and 𝑃𝐴. We further introduce a
bi-objective optimization variant of GNRM, where we wish to minimize both r
and k subject to upper bounds r≤r̅ and k≤k̅, where
r̅ and k̅ are constants. We show that the Pareto front of this
bi-objective optimization problem (BO-GNRM) can be computed in fixed-parameter
tractable time with parameter r̅+k̅.
We then report the results of our experimental work using the integer
programming solver Gurobi to solve instances of BO-GNRM. Our key findings are
that (a) we obtained strong support that Gurobi's performance is
fixed-parameter tractable, (b) our results suggest that our techniques may be
useful for role mining in practice, based on our experiments in the context of
three well-known real-world authorization policies.
更多查看译文
AI 理解论文
溯源树
样例
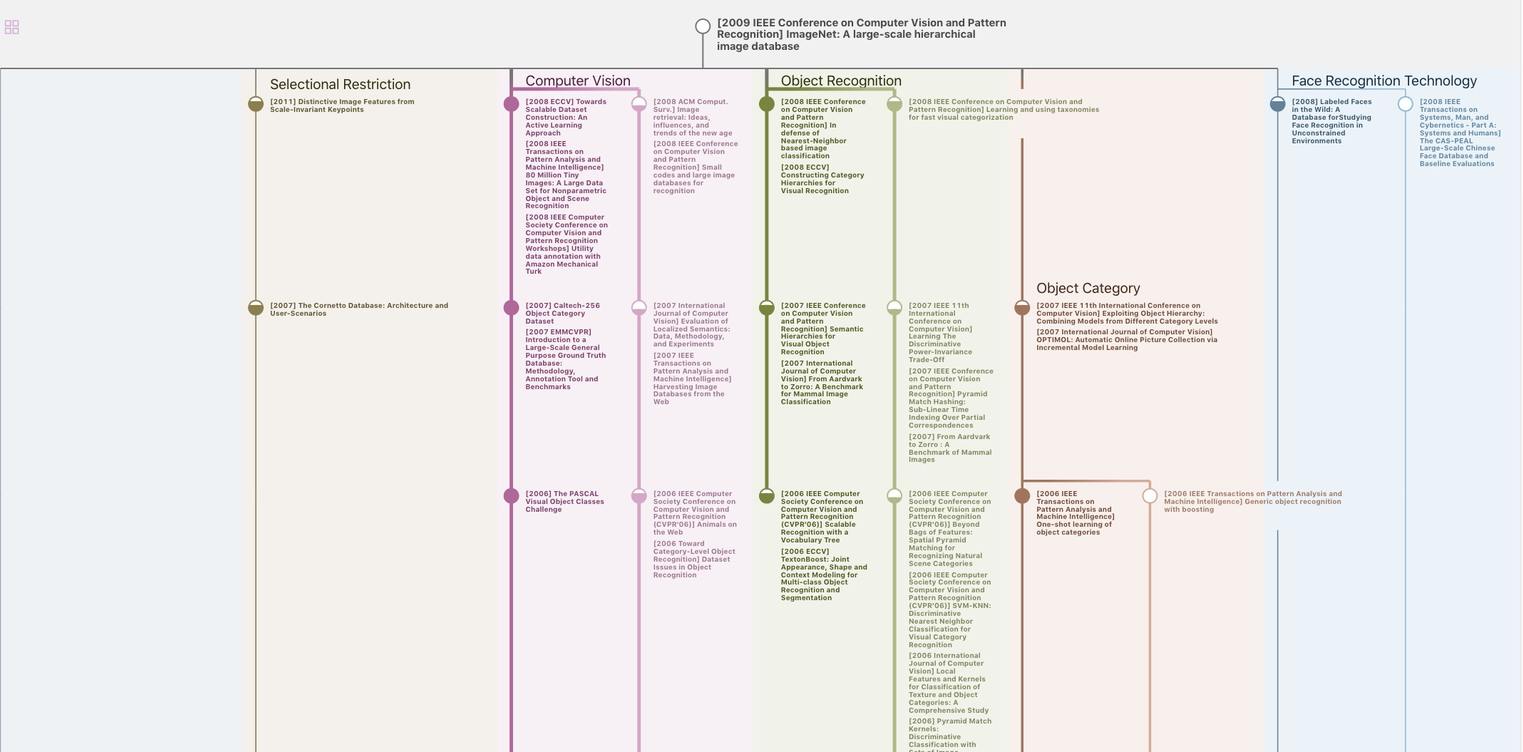
生成溯源树,研究论文发展脉络
Chat Paper
正在生成论文摘要