Droplet shape representation using Fourier series and autoencoders
arxiv(2024)
摘要
The shape of liquid droplets in air plays an important role in aerodynamic
behavior and combustion dynamics of miniaturized propulsion systems such as
microsatellites and small drones. Their precise manipulation can yield optimal
efficiency in such systems. It is desired to have a minimal representation of
droplet shapes using as few parameters to automate shape manipulation using
self-learning algorithms, such as reinforcement learning. In this paper, we use
a neural compression algorithm to represent, with only two parameters,
elliptical and bullet-shaped droplets initially represented with 200 points
(400 real numbers) at the droplet boundary. The mapping of many to two points
is achieved in two stages. Initially, a Fourier series is formulated to
approximate the contour of the droplet. Subsequently, the coefficients of this
Fourier series are condensed to lower dimensions utilizing a neural network
with a bottleneck architecture. Finally, 5000 synthetically generated droplet
shapes were used to train the neural network. With a two real numbers
representation, the recovered droplet shapes had excellent overlap with the
original ones, with a mean square error 10^-3. Hence, this method compresses
the droplet contour to merely two numerical parameters via a fully reversible
process, a crucial feature for rendering learning algorithms computationally
tractable.
更多查看译文
AI 理解论文
溯源树
样例
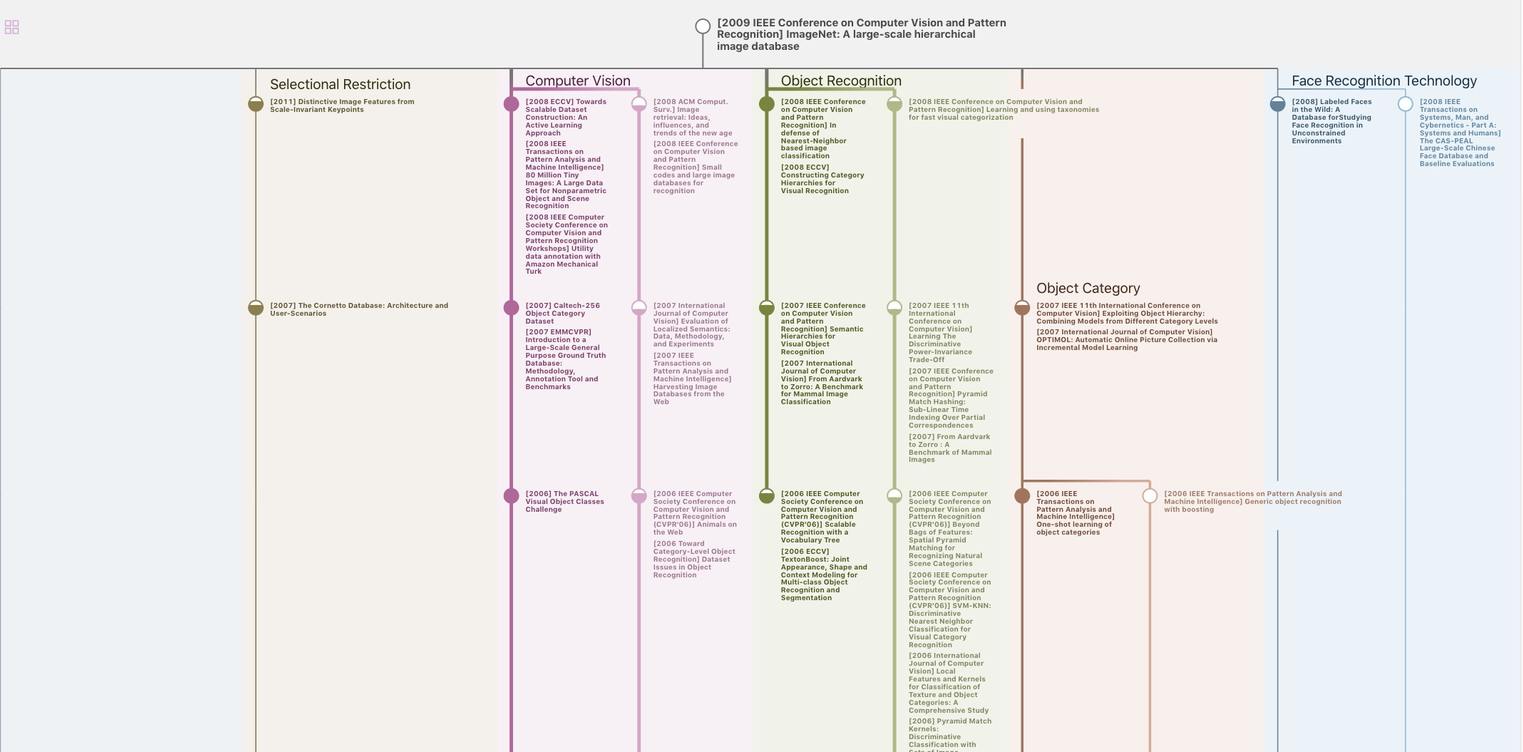
生成溯源树,研究论文发展脉络
Chat Paper
正在生成论文摘要