DCL-depth: monocular depth estimation network based on iam and depth consistency loss
Multimedia Tools and Applications(2024)
摘要
The self-supervised monocular depth estimation algorithm obtains excellent results in outdoor environments. However, traditional self-supervised depth estimation methods often suffer from edge blurring in complex textured regions and the loss of depth information in pixels within weakly-textured areas. To enhance the perception ability of the deep network for complex textured areas and the accuracy of depth estimation in weakly-textured regions, the following methods are proposed in this paper. First of all, the image activity measure (IAM) is used to segment the image features. Based on the multi-directional distribution of image contours, the network's perception ability has been improved, resulting in effective enhancement of depth estimation in complex regions. Furthermore, a new loss function called depth consistency loss (DCL) is proposed, which is based on recursive recurrent networks. The DCL aims to measure the similarity between the output images of the first-order network and the second-order network, and the network's constraint on weak-texture regions has been strengthened. By employing this approach, the accuracy of estimating depth information in weakly-textured regions can be enhanced. Through adequate experimentation on the public indoor datasets, the results show that our network outperforms the compared algorithms in terms of accuracy and visualization of predicted depth.
更多查看译文
关键词
Depth estimation,Self-Supervised learning,Image activity measure,Depth consistency loss
AI 理解论文
溯源树
样例
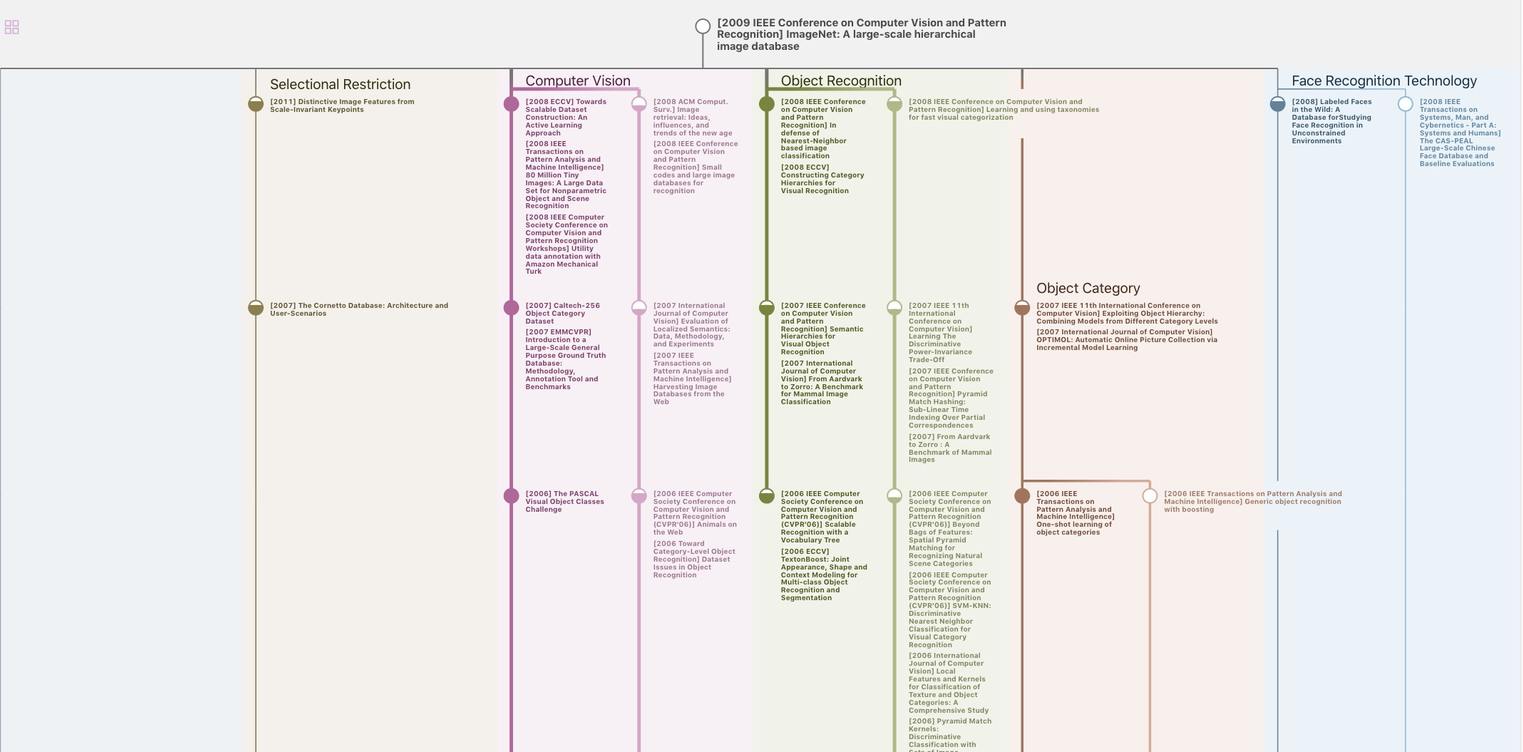
生成溯源树,研究论文发展脉络
Chat Paper
正在生成论文摘要