A novel framework for quantitative attribution of particulate matter pollution mitigation to natural and socioeconomic drivers
Science of The Total Environment(2024)
摘要
Quantifying drivers contributing to air quality improvements is crucial for pollution prevention and optimizing local policies. Despite advances in machine learning for air quality analysis, their limited interpretability hinders attribution on global and local scales, vital for informed city management. Our study introduces an innovative framework quantifying socioeconomic and natural impacts on mitigation of particulate matter pollution in 31 Chinese major cities from 2014 to 2021. Two indices, formulated based on the additivity of Shapley additive explanations, are proposed to measure driver contributions globally and locally. Our analysis explores the self-contained and interactive effects of these drivers on particulate levels, pinpointing critical threshold values where these drivers trigger shifts in particulate matter levels. It is revealed that SO2, NOx, and dust emission reductions collectively account for 51.58 % and 51.96 % of PM2.5 and PM10 decreases at the global level. Moreover, our findings unveil a significant heterogeneity in driver contributions to pollutant mitigation across distinct cities, which can be instrumental in crafting location-specific policy recommendations.
更多查看译文
关键词
Quantitative attribution,Particulate matter pollution,Interpretable machine learning,Natural and socioeconomic drivers,Chinese major cities
AI 理解论文
溯源树
样例
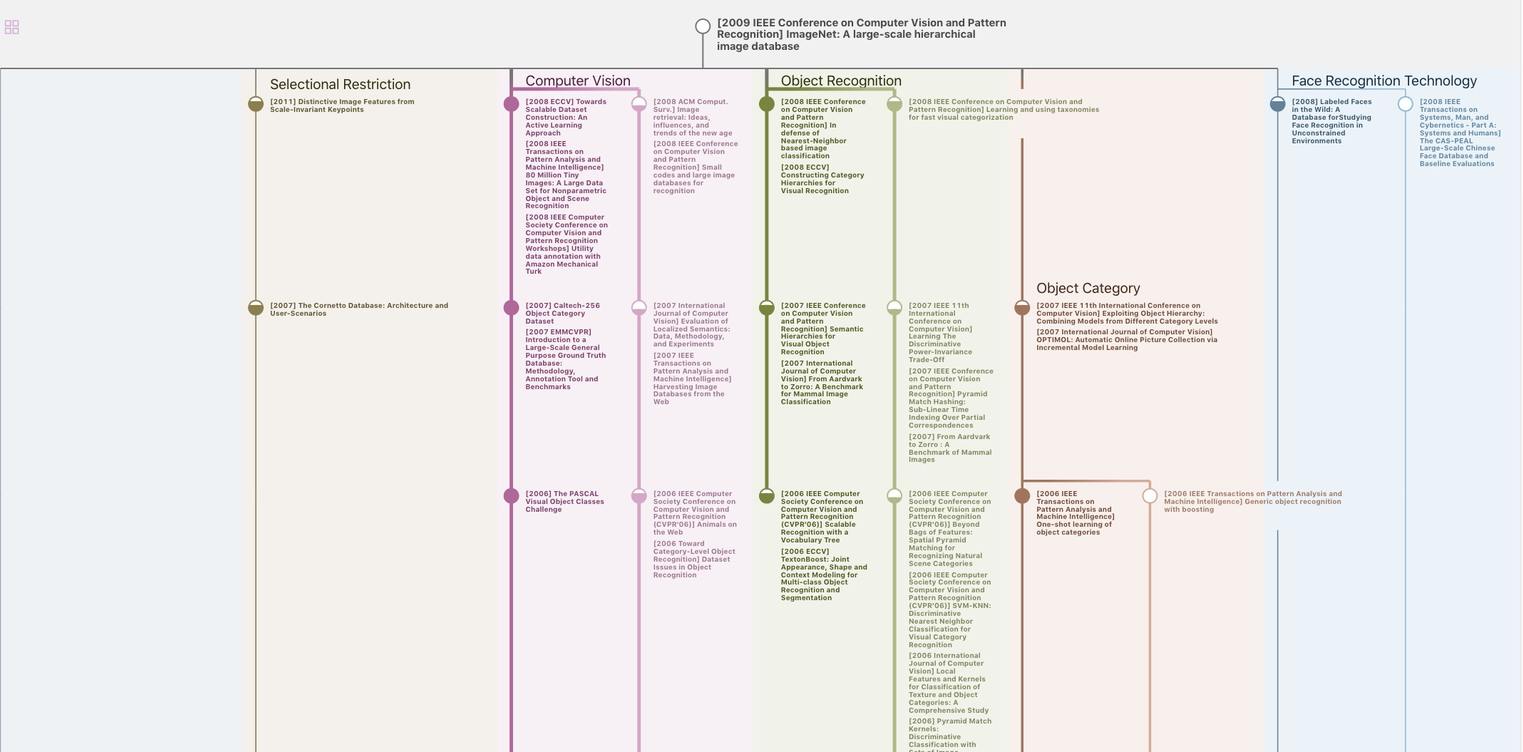
生成溯源树,研究论文发展脉络
Chat Paper
正在生成论文摘要