Reversible jump attack to textual classifiers with modification reduction
Machine Learning(2024)
摘要
Recent studies on adversarial examples expose vulnerabilities of natural language processing models. Existing techniques for generating adversarial examples are typically driven by deterministic hierarchical rules that are agnostic to the optimal adversarial examples, a strategy that often results in adversarial samples with a suboptimal balance between magnitudes of changes and attack successes. To this end, in this research we propose two algorithms, Reversible Jump Attack (RJA) and Metropolis–Hasting Modification Reduction (MMR), to generate highly effective adversarial examples and to improve the imperceptibility of the examples, respectively. RJA utilizes a novel randomization mechanism to enlarge the search space and efficiently adapts to a number of perturbed words for adversarial examples. With these generated adversarial examples, MMR applies the Metropolis–Hasting sampler to enhance the imperceptibility of adversarial examples. Extensive experiments demonstrate that RJA-MMR outperforms current state-of-the-art methods in attack performance, imperceptibility, fluency and grammar correctness.
更多查看译文
关键词
Textual attack,Adversarial learning,Natural language processing
AI 理解论文
溯源树
样例
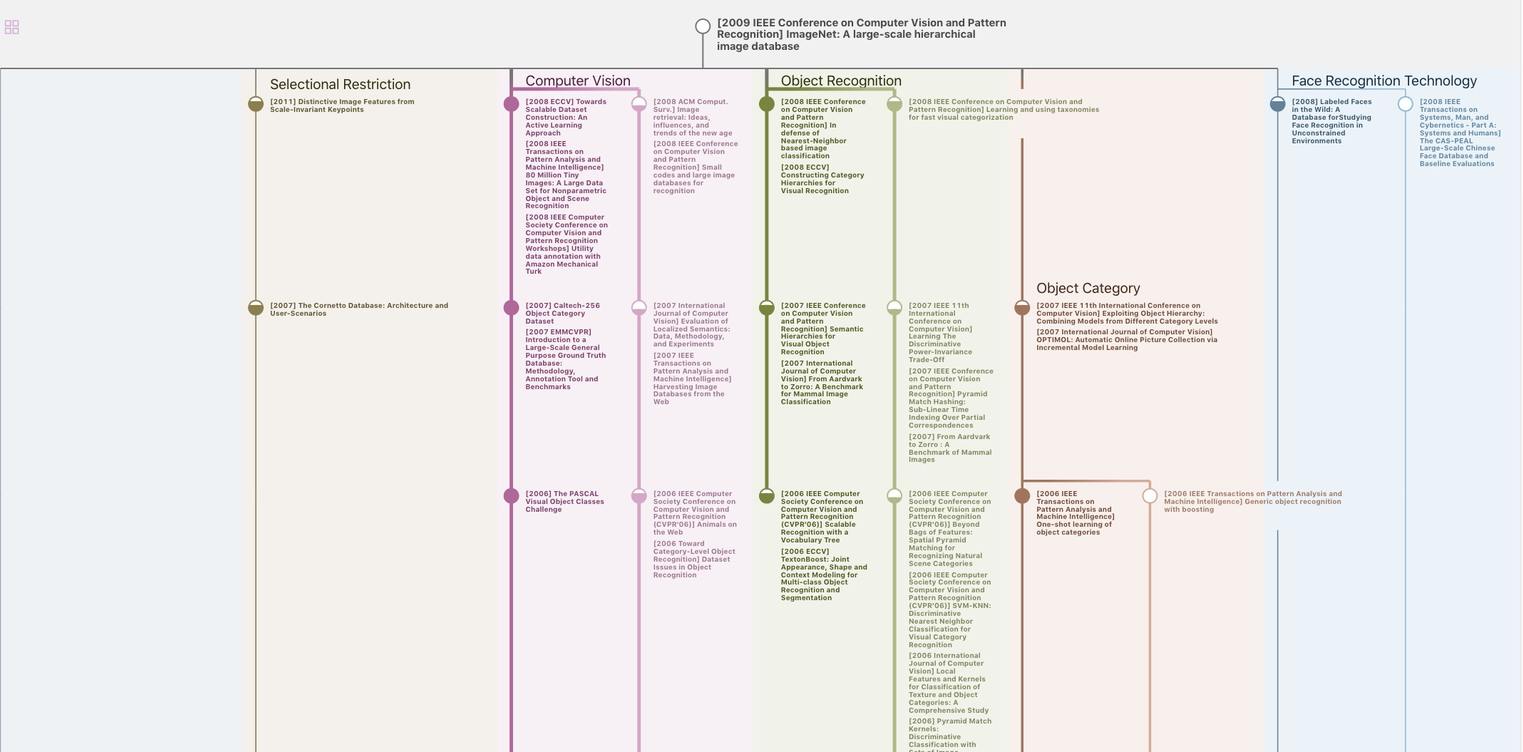
生成溯源树,研究论文发展脉络
Chat Paper
正在生成论文摘要