Typical lake area is accurately predicted and assessed based on deep learning algorithms and associated physical mechanisms
Earth Science Informatics(2024)
摘要
Accurate quantification of lake areas is pivotal for understanding regional hydrological cycles and conserving wetlands. Existing methodologies, including remote sensing interpretations and conventional deep learning approaches, face inherent limitations. Addressing this, the present study introduces an innovative deep learning model for lake area prediction, incorporating physical mechanisms. Initially, the study extracts the monthly areas of East Dongting Lake over 2000 to 2020, utilizing the Google Earth Engine (GEE) platform. Subsequently, it integrates water level data from Chenglingji, developing a response relationship between area and water level. This process enhances the loss function of the traditional Long Short-Term Memory (LSTM) model, leading to the creation of a physically informed Area and Water Level-LSTM model (AWL-LSTM). This model is then assessed in comparison to its traditional LSTM counterpart.The findings reveal a pronounced shrinking trend in the water area of East Dongting Lake over the past two decades, characterized by significant seasonal fluctuations. In periods of rising, high, and receding water levels, the lake's area exhibits a robust correlation with the Chenglingji water levels. However, this correlation weakens during low water periods. The AWL-LSTM model outperforms the conventional LSTM in several aspects, including extensibility, precision, interpretability, and stability. It demonstrates superior accuracy in peak data series fitting, evidenced by a 34
更多查看译文
关键词
Deep learning,Physical mechanism,Lake forecast,East Dongting Lake
AI 理解论文
溯源树
样例
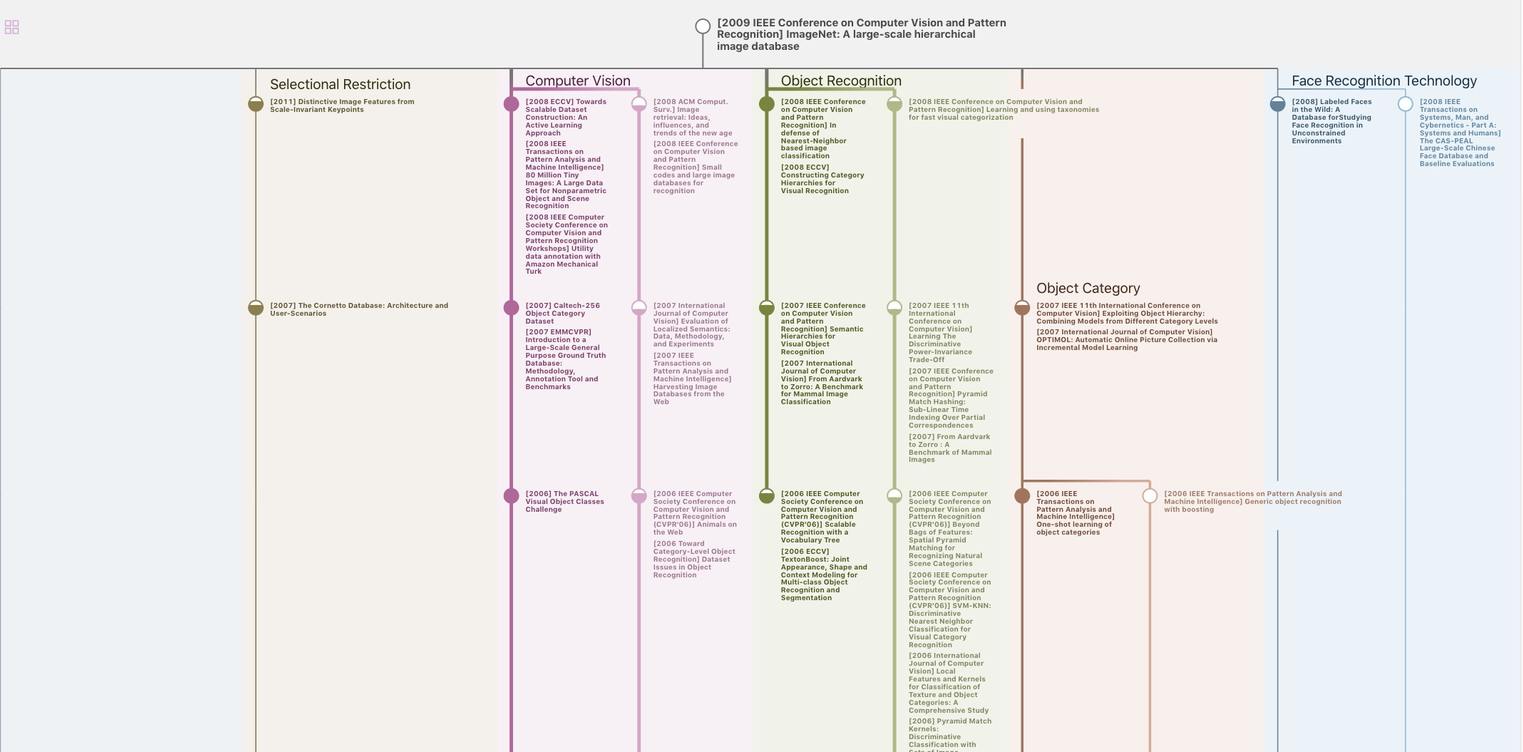
生成溯源树,研究论文发展脉络
Chat Paper
正在生成论文摘要