Modified Flower Pollination Algorithm for Energy Forecasting and Demand Management Coupled with Improved Battery Life for Smart Building Micro-Grid
2024 IEEE Texas Power and Energy Conference (TPEC)(2024)
摘要
This paper presents the Modified Flower Pollination Algorithm-based Multi-Layer Perceptron Neural Network (MFPA-MLPNN) as an optimization technique for efficient power flow management in a Smart Building Microgrid (SBMG) in-tegrated with solar and wind generation, and Electric Vehicle Batteries (EVBs) within grid connected structure while concurrently reducing optimization processing time. To achieve both technical and economic superiority, two optimization objectives are addressed. Firstly, a Demand Response (DR) framework is harnessed to accommodate the stochastic behavior and forecasting errors associated with intermittent sources. Secondly, the degradation of EVBs is considered, ensuring an economically viable power flow proposed strategy for both EV owners and mi-crogrid (MG) authorities. Power generation of Variable Renew-able Energy Sources (VRES) has been forecasted using MLPNN. Battery degradation and system stability under the action of the proposed topology have been evaluated using a simulation-based environment. Results show a significant decrease in battery degradation and processing time using the proposed MFPA-MLPNN optimization architecture.
更多查看译文
关键词
Smart Building Microgrid,Renewable energy,Electric vehicle batteries,Energy management,Demand response,Flower pollination algorithm
AI 理解论文
溯源树
样例
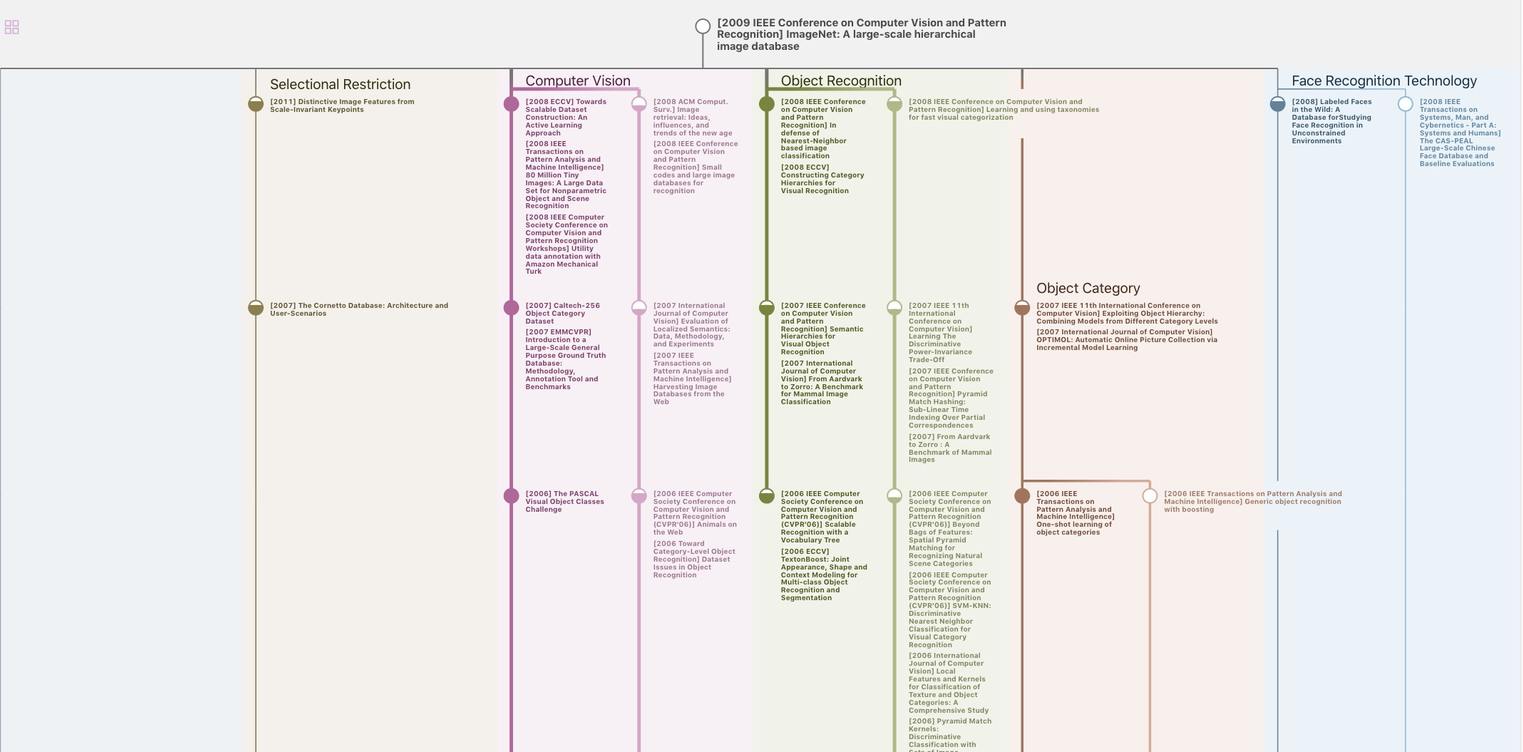
生成溯源树,研究论文发展脉络
Chat Paper
正在生成论文摘要