Designing Social Good Semantic Computing Architectures for the Long Tail: Case Studies, Evaluation, and Challenges.
IEEE International Conference on Semantic Computing(2024)
摘要
Many real-world systems, especially systems characterized by high social activity (such as the Web), tend to obey power law distributions and thereby have a significant ‘long tail’. We argue that researching, developing and designing semantic computing systems for the long tail, especially dependent on inductive AI, constitutes an important class of problems, not least because the long tail is challenging both technically and socially. By its very nature, the long tail is irregular, testing the generalization capabilities of the state-of-the-art, especially in architectures and interfaces that are built on some form of machine learning or statistical inference (including large language models). As machine learning and generative AI continues to be integrated into more front facing systems, the issue of the long tail cannot be ignored by either the systems engineering or the AI communities. We present two case studies with important social consequences (fighting human trafficking online, and managing information effectively and in real-time during humanitarian crises) where semantic computing and AI platforms specifically designed to handle long-tail challenges find critical application, and sometimes with drastically different design choices compared to designing only for the short tail (with the main goal of maximizing average accuracy).
更多查看译文
关键词
Long tail,social good,crisis response,human trafficking
AI 理解论文
溯源树
样例
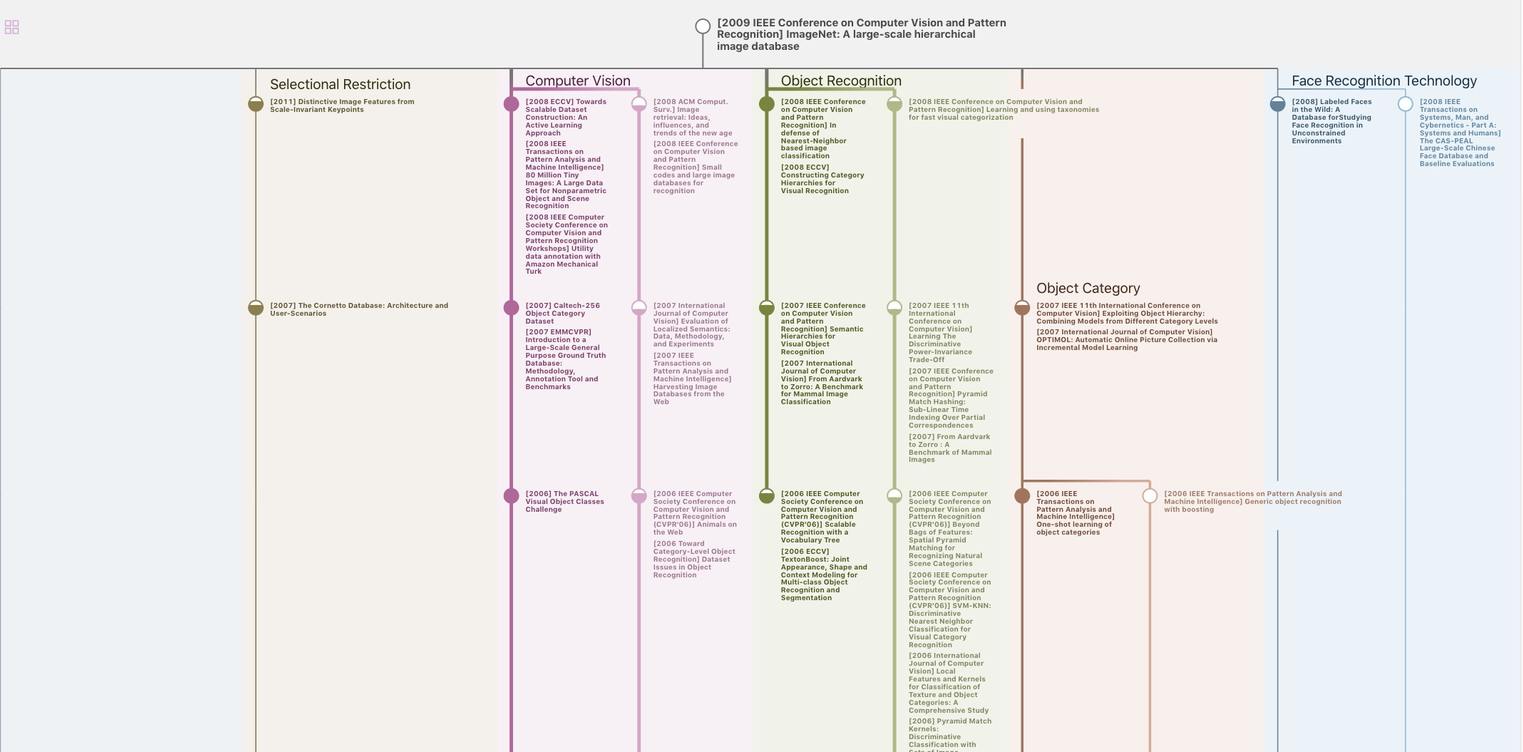
生成溯源树,研究论文发展脉络
Chat Paper
正在生成论文摘要