Abstract 4911: PAIRWISE: Deep learning-powered personalized medicine approach identifies actionable drug combinations in B cell lymphoma
Cancer Research(2024)
摘要
Abstract Combination drug therapies offer promise for improving cancer treatment. However, identifying actionable drug combinations for specific cancer subtypes and individual patients remains challenging due to the vast number of possibilities. Existing approaches like high-throughput screening (HTS) and rule-based drug additions have limitations in their ability to predict clinically translatable synergistic combinations. Therefore, advanced machine learning focused on patient-centric modelling have the potential to optimize combination therapies. PAIRWISE is a patient-centric deep learning-powered drug combination recommendation platform. Given gene expression along with chemical structures and targets from a set of two drugs being applied, the output is the binary combination outcome. PAIRWISE introduces a new approach of representing tumor genomics using the unsupervised pretraining design on 11K TCGA tumors. The power of PAIRWISE is derived from knowledge-guided transfer learning by learning from in vitro experiments with drug-drug-cell interactions and cellular heterogeneity. PAIRWISE can accurately predict combination synergy with area under the curve (AUC) > 0.85 across 10 tissue types in a large dataset of 200K in vitro drug combinations and significantly outperform all state-of-the-art methods. Diffuse large B cell lymphoma (DLBCL) is the most common B cell non-Hodgkin lymphoma and remains incurable in around 40% of patients. Finding optimal combination therapy for each DLBCL patient is a critical unmet need. As independent validation, we applied PAIRWISE to an external HTS study of Bruton Tyrosine Kinase inhibitor (BTKi) combinations (Griner et al., 2014), confirming the reproducibility and accuracy of high-confidence predictions (AUC = 0.72). PAIRWISE was also applied to transcriptomic data of 29 DLBCL cell lines (Bal et al., 2022) and predicted BTKi-containing combinations across ABC subtypes with higher synergy scores than GCB (P = 0.02). This is consistent with expected better overall efficacy of BTK inhibitors in ABC subtype (Younes et al., 2019; Davis et al., 2010; Wilson et al., 2015). To predict opportunities in DLBCL, PAIRWISE was used to identify BTKi partners for an additional 211 anti-cancer drugs using 562 published ex vivo DLBCL samples (Schmitz et al., 2018). PAIRWISE effectively identified novel DLBCL sub-population with significantly higher response scores for predicted BTKi combinations and favorable survival. Notably, patients predicted as responsive had significantly better outcomes upon first-line chemotherapy treatments than non-responsive ones (P < 0.0001). Collectively, we demonstrate that PAIRWISE is a highly efficient and generalizable framework that enables confident predictions of pairwise drug combinations at disease subtype level, with the potential of informing treatment options and clinical trial design. Citation Format: Chengqi Xu, Jake Cohen-Setton, Zhouyang Shen, Marta Milo, Ilkay US, Ben Sidders, Jude Fitzgibbon, Heng Pan, Olivier Elemento, Krishna Bulusu. PAIRWISE: Deep learning-powered personalized medicine approach identifies actionable drug combinations in B cell lymphoma [abstract]. In: Proceedings of the American Association for Cancer Research Annual Meeting 2024; Part 1 (Regular Abstracts); 2024 Apr 5-10; San Diego, CA. Philadelphia (PA): AACR; Cancer Res 2024;84(6_Suppl):Abstract nr 4911.
更多查看译文
AI 理解论文
溯源树
样例
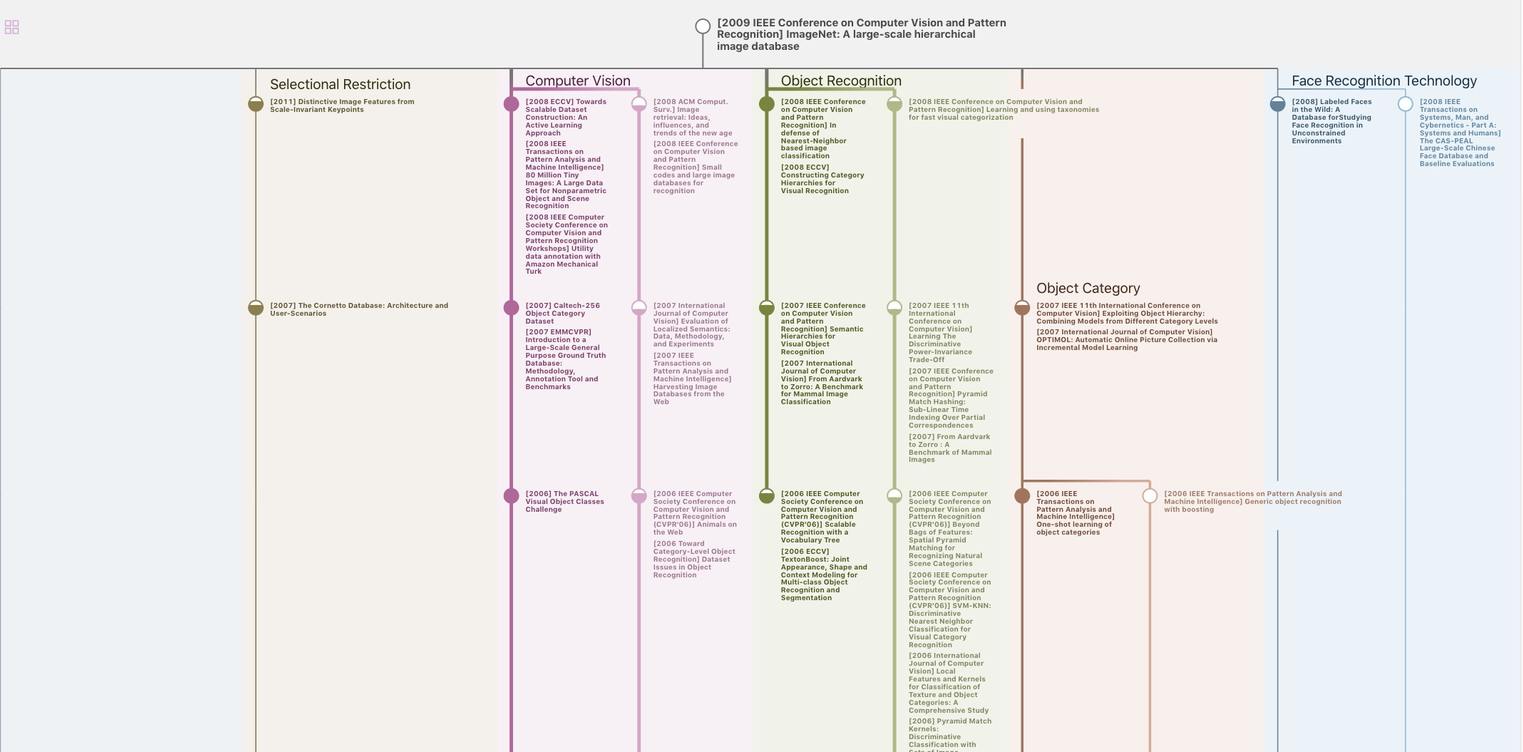
生成溯源树,研究论文发展脉络
Chat Paper
正在生成论文摘要