Model Explainable AI Method for Fault Detection in Inverter-Based Distribution Systems
2024 IEEE Texas Power and Energy Conference (TPEC)(2024)
摘要
This paper proposes a model-based explainable artificial intelligence (XAI) architecture for the detection of short circuit faults in inverter-based distribution systems. The architecture consists of two artificial neural networks - one for fault type and the other for fault location classification, which work in tandem for the overall fault detection. These neural networks are trained on synthetic fault data produced in simulation using a test distribution network with inverter-based resource generation. Once a high training accuracy is achieved for each model, Shapley values are employed to provide explanations of the mechanism into how each black-box model - i.e., the neural network- on average, uses each input feature leading to the output response.
更多查看译文
关键词
Explainable AI,IBDER,intelligent fault detection,Shapley value,XAI
AI 理解论文
溯源树
样例
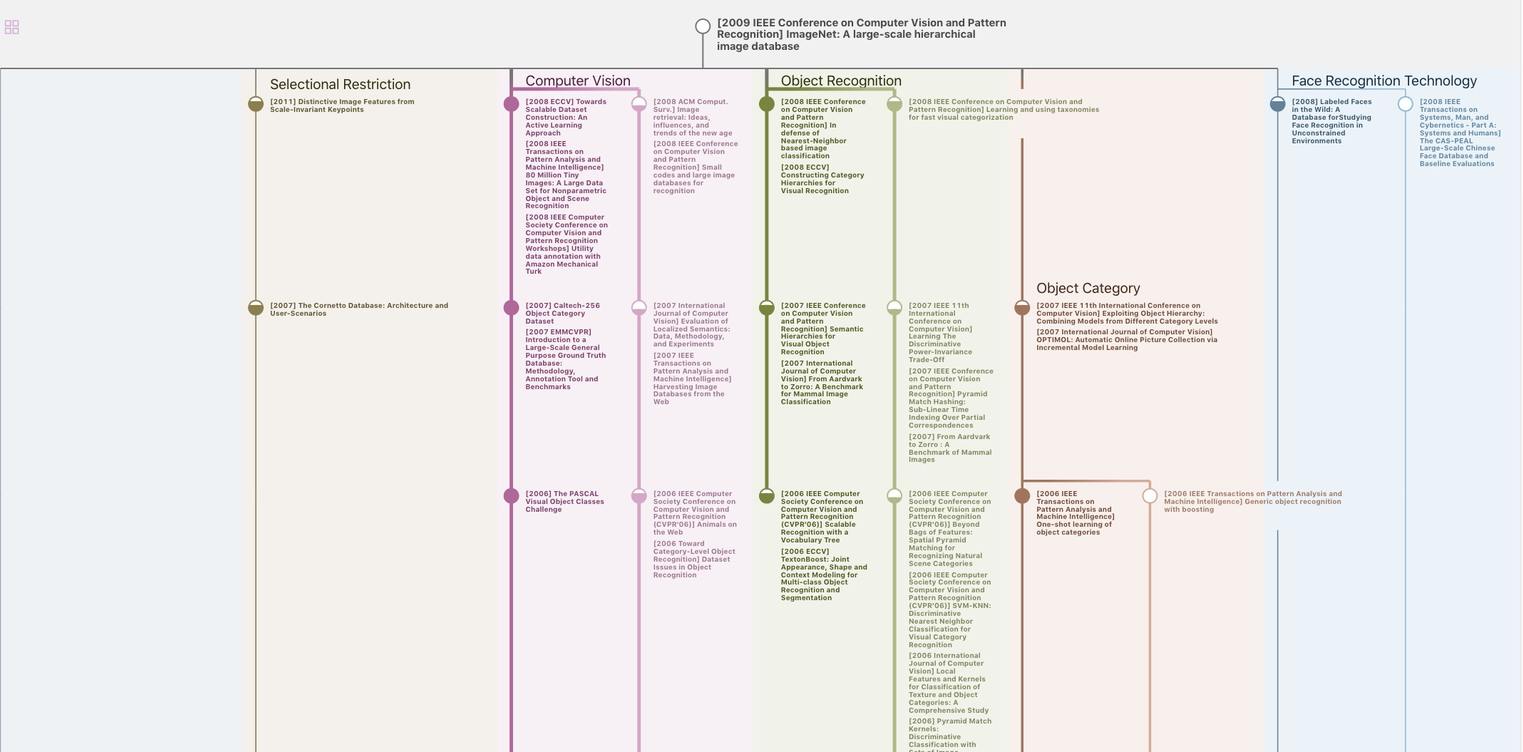
生成溯源树,研究论文发展脉络
Chat Paper
正在生成论文摘要