Abstract 4888: Multi-modal knowledge graphs enhance patient stratification & biomarker discovery
Cancer Research(2024)
摘要
Abstract Background: Multi-modal analysis is crucial for deeper understanding of disease subtypes and more meaningful patient selection. We developed a flexible Knowledge Graph (KG) framework that enables deep multi-omic analysis. It can be used to uncover the interrelationships between the layers of data in a population to inform patient selection or biomarker discovery. We present an application of our framework to non-small cell lung cancer (NSCLC) to identify and separate communities of patients based on their survival and identify the associated biomarkers. We identified potentially mislabeled patients that do not share all the characteristics of the cancer subtype to which they are assigned: either lung adenocarcinoma (LUAD) or lung squamous cell carcinoma (LUSC). Crucially, the community-based biomarkers for poor or long survivors were validated on the whole population. Methods: Our KG framework was leveraged by applying supervised community detection to NSCLC data from TCGA, specifically RNA expression and DNA methylation, with overall survival (OS) as the endpoint (n=999 subjects). Biomarkers associated to each community were ranked based on their prevalence inside against their prevalence outside the community. Results: We obtained 3 communities (391 + 229 + 379 patients) that are all significantly separated by their OS (p<0.05). While KG-derived communities largely overlapped with histology-labelled LUAD or LUSC (concordant LUAD (n=330)/concordant LUSC (n=342)), a small number of patients did not (discordant LUAD (n=37)/discordant LUSC (n=61)). Discordant LUAD patients had significantly lower OS than concordant LUAD (p=0.0198), despite being both labeled as LUAD. Many of the discordant LUSC lacked the 3q26 amplification commonly seen in LUSC and other squamous cell carcinomas. The results from our KG framework highlight its increased sensitivity in relation to existing tools (Cline, Sci Rep 3, 2652 (2013)) as we identified a discordant LUAD group in addition to a discordant LUSC group. Moreover, our tool can select the biomarkers most prevalent in each community, and these significantly separated long from poor survivors on the whole population (p=3.53e-5). Some of these KG-identified biomarkers are known regulators of progression and survival in NSCLC, whereas others are still not extensively studied. This highlights another advantage of our approach in prospective target discovery. Conclusions: Our KG framework allowed the observation of potential misclassifications of tumor subtypes in NSCLC (TCGA). This approach is a proof-of-concept of the value KGs have in identifying signals in muti-omic data that may improve patient stratification and uncover associated biomarker signatures. Our flexible end-to-end framework can take any type of ‘omic data and can be applied to any tumor type for such findings, which represents an alternative to pre-defined KG architectures with defined relationships. Citation Format: Miguel Goncalves, Jake Cohen-Setton, Ioannis Kagiampakis, Ben Sidders, Krishna Bulusu. Multi-modal knowledge graphs enhance patient stratification & biomarker discovery [abstract]. In: Proceedings of the American Association for Cancer Research Annual Meeting 2024; Part 1 (Regular Abstracts); 2024 Apr 5-10; San Diego, CA. Philadelphia (PA): AACR; Cancer Res 2024;84(6_Suppl):Abstract nr 4888.
更多查看译文
AI 理解论文
溯源树
样例
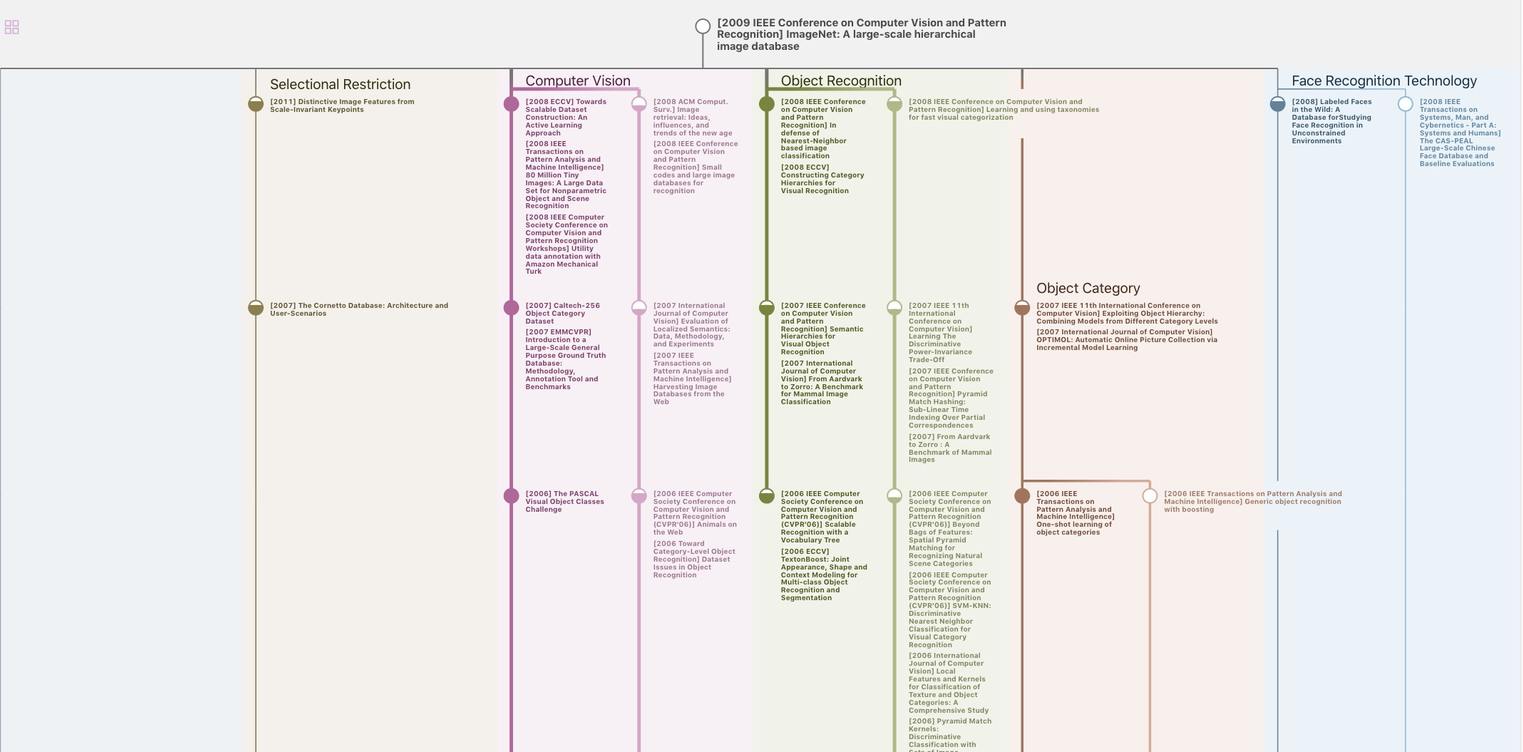
生成溯源树,研究论文发展脉络
Chat Paper
正在生成论文摘要