Abstract 2268: CREDAC: Copy number-based reference-free expression deconvolution analysis of cancers
Cancer Research(2024)
摘要
Abstract Introduction: Analyses of bulk RNA sequencing data are central to most large-scale tumor sequencing studies. Bulk expression data represent population averages and its interpretation is confounded by both normal cell contamination and somatic copy number alterations. Several computational methods to deconvolve have been developed recently. However, these methods often rely on a set of cell-type-specific reference signatures and ignore the effect of copy number changes. Methods: To address these issues, we have developed a method that formalizes the relationship between allele-specific copy number, expression and sample purity to deconvolve the expression profiles of tumor and normal cells from bulk RNA-seq in an unbiased manner. Our method was applied to sequencing data produced by the TRACERx consortium, a longitudinal study with multi-region whole-exome and RNA-seq of non-small-cell lung cancers. A total of 414 primary tumor regions and 140 adjacent normal tissue samples from 140 patients with matched DNA and RNA-seq data were processed. Results: We were able to directly deconvolve a median of ~2,000 genes per sample and indirectly infer tumor and normal expression profiles of ~10,000 genes using an scaled and weighted mean approach. The accuracy of the deconvolution was validated using (1) in-silico mixtures of patient-derived tumor and normal cells, (2) pseudo-bulk scRNAseq and (3) in regions with loss of heterogeneity (LOH) directly on the bulk sequencing data, where the total fraction of expression attributed to tumor cells can be computed directly using somatic mutations. CREDAC allows for single sample bulk-level tumor-normal differential expression analysis and revealed a strong and constitutive genome-wide overexpression in cancer compared to admixed normal cells, with a more pronounced overexpression in lung squamous cell carcinoma than lung adenocarcinoma (p<0.001). CREDAC adjusts for variations in copy number, thus facilitating the investigation of cancer-specific dosage compensatory responses triggered by aneuploidy. We revealed that a majority of genes (~65%) exhibit proportionality to copy number alterations, while a substantial proportion (~35%) shows anti-scaling or dosage compensation strategies. We show an enrichment of genes involved in cell cycle and expression processes within the dosage compensated group and revealed methylation as a key mechanism driving this compensatory process. Conclusion: Overall, our results suggest that CREDAC is able to accurately disentangle the expression of tumor and normal cells from bulk RNA-seq without any previous knowledge. It has potential applications in many studies that include matched RNA-seq and copy number data and can provide new insights functional characterization, the taxonomy of cancer, and tumor evolution. Citation Format: Carla Castignani, Jonas Demeulemeester, Oriol Pich, Tom Lesluyes, Robert E. Hynds, David R. Pearce, Elizabeth Larose Cadieux, Stefan C. Dentro, TRACERx Consortium, Nnenna Kanu, Charles Swanton, Peter Van Loo. CREDAC: Copy number-based reference-free expression deconvolution analysis of cancers [abstract]. In: Proceedings of the American Association for Cancer Research Annual Meeting 2024; Part 1 (Regular Abstracts); 2024 Apr 5-10; San Diego, CA. Philadelphia (PA): AACR; Cancer Res 2024;84(6_Suppl):Abstract nr 2268.
更多查看译文
AI 理解论文
溯源树
样例
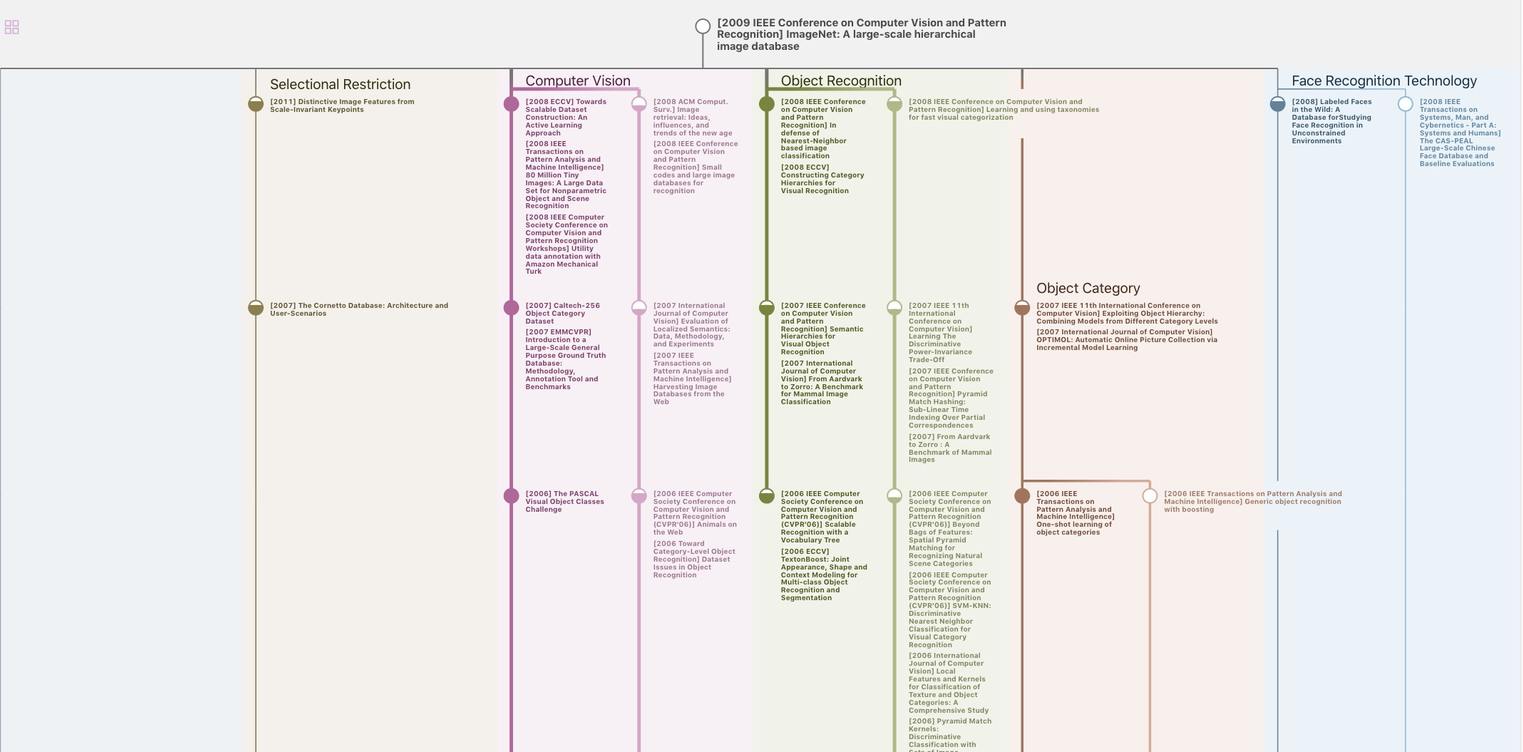
生成溯源树,研究论文发展脉络
Chat Paper
正在生成论文摘要