Abstract 6467: Causal personalized treatment estimation framework using real-world electronic health record (EHR) data to inform cancer care decisions
Cancer Research(2024)
摘要
Abstract Background: The average treatment effects (ATEs) from randomized controlled trials often do not generalize across populations, or they are not sufficiently personalized to capture the benefits and risks to individual patients. Real-world data aids in validating and extending trial results, but can introduce biases that invalidate the causal interpretation. Here, we propose a robust statistical framework, which uses real-world EHR data to evaluate the efficacy of cancer treatments at both population and individual levels. We demonstrate the ability of our framework to deliver personalized insights in metastatic prostate cancer treatments. Methods: We assembled a retrospective cohort of metastatic prostate cancer patients from a large academic medical center. We gathered demographic, comorbidity, cancer treatment, and outcome data from the EHR or natural language processing of clinician narratives, and social determinants of health from Census data. We used doubly robust machine learning with bootstrap standard errors to compute the ATE and personalized treatment estimates (PTEs), comparing the impact of ADT (androgen depravation therapy) + chemotherapy (ACT) and ADT + combination anti-androgen therapy (ACAT) on percentage reduction in PSA levels in metastatic prostate cancer. Results: The baseline characteristics of our cohort are summarized in Table 1a. Our approach found that ACAT reduces PSA levels significantly more than ACT, and captured the heterogeneity in treatment effects across individuals (Table 1b). Through simulation studies, we demonstrated that any reasonable bias from unobserved confounding or missing information was small compared to the estimated treatment effects and their standard errors. Conclusion: We presented a doubly robust framework and demonstrated its utility in analyzing real-world data, enabling us to uncover the diversity in treatment responses among individuals with metastatic prostate cancer. Results summary a. Baseline demographic characteristics. ADT + chemotherapy N = 302 ADT + combination therapy N = 114 Treatments Any ADT + Docetaxel or Cabazitaxel Any ADT + Abiraterone or apalutamide or Enzalutamide Age Mean (SD) 69.3 (8.76) 66.7 (8.17) Median [Min, Max] 69.0 [47.0, 94.0] 66.5 [46.0, 86.0] Race Asian 8 (2.6%) 2 (1.8%) Black or African American 48 (15.9%) 7 (6.1%) White or Caucasian 224 (74.2%) 99 (86.8%) Other 22 (7.3%) 6 (5.3%) Max PSA treatment start (ng/mL) Mean (SD) 34.9 (98.5) 83.3 (448) b. Treatment effects estimation ATE (95% CI) 32.0% [24.6%, 40.8%] PTEs: 25th and 75th percentiles 29.0%, 34.3% Citation Format: Sumana Srivatsa, David Walsh, Mayada Aljehani, Jason Weinreb, Nathan Becker, Benjamin H. Ellis, Renee George, Xingyao Chen, Naim Matasci, Reva Basho, Mitchell E. Gross, Christine L. Swisher. Causal personalized treatment estimation framework using real-world electronic health record (EHR) data to inform cancer care decisions [abstract]. In: Proceedings of the American Association for Cancer Research Annual Meeting 2024; Part 1 (Regular Abstracts); 2024 Apr 5-10; San Diego, CA. Philadelphia (PA): AACR; Cancer Res 2024;84(6_Suppl):Abstract nr 6467.
更多查看译文
AI 理解论文
溯源树
样例
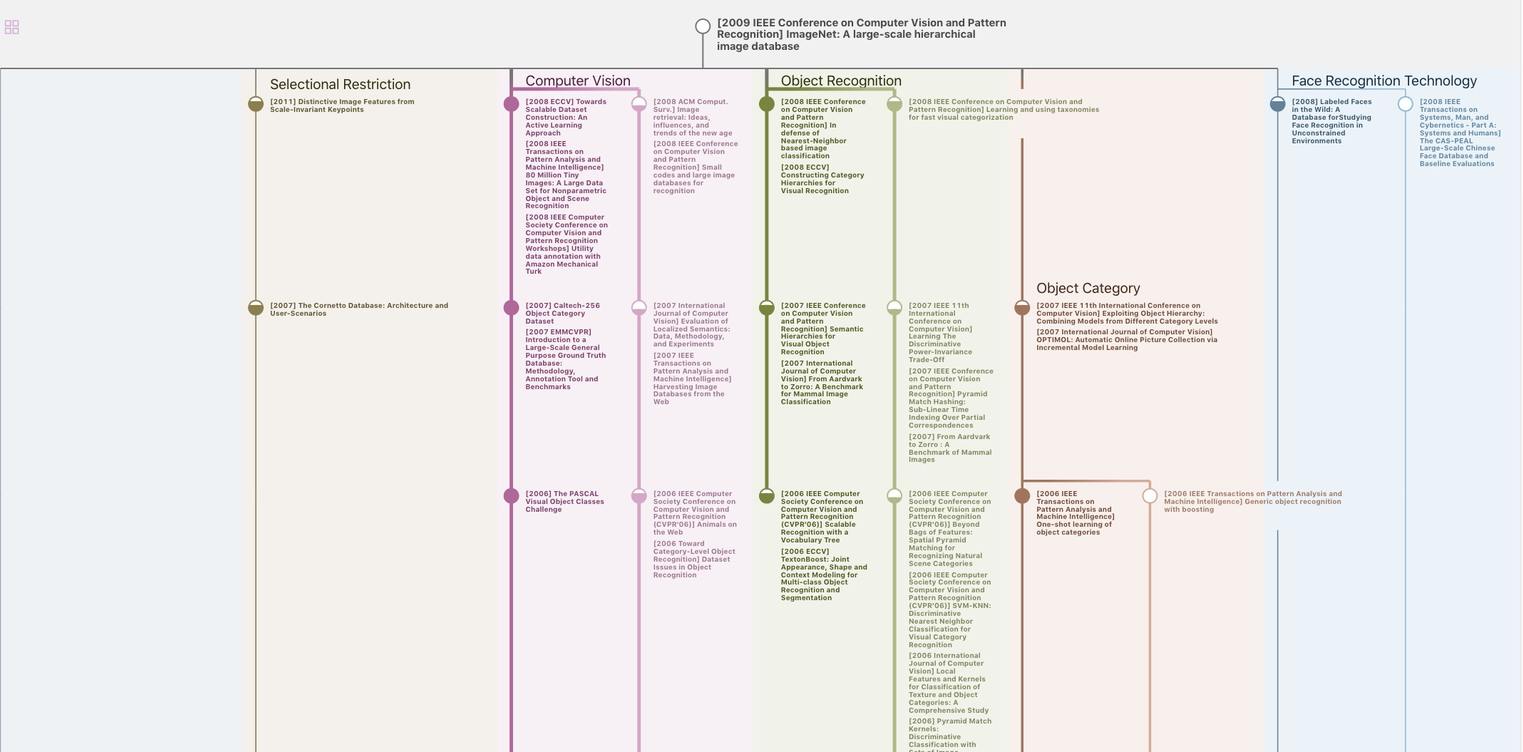
生成溯源树,研究论文发展脉络
Chat Paper
正在生成论文摘要