A Self-consistent Data-driven Model for Determining Stellar Parameters from Optical and Near-infrared Spectra
The Astronomical Journal(2024)
摘要
Data-driven models, which apply machine learning to infer physical properties from large quantities of data, have become increasingly important for extracting stellar properties from spectra. In general, these methods have been applied to data in one wavelength regime or another. For example, APOGEE Net has been applied to near-IR spectra from the Sloan Digital Sky Survey (SDSS)–V APOGEE survey to predict stellar parameters ( T _eff , log g , and [Fe/H]) for all stars with T _eff from 3000 to 50,000 K, including pre-main-sequence stars, OB stars, main-sequence dwarfs, and red giants. The increasing number of large surveys across multiple wavelength regimes provides the opportunity to improve data-driven models through learning from multiple data sets at once. In SDSS-V, a number of spectra of stars will be observed not just with APOGEE in the near-IR, but also with BOSS in the optical regime. Here, we aim to develop a complementary model, BOSS Net, that will replicate the performance of APOGEE Net in these optical data through label transfer. We further improve the model by extending it to brown dwarfs, as well as white dwarfs, resulting in a comprehensive coverage between 1700 < T _eff < 100,000 K and 0 < $\mathrm{log}g$ < 10, to ensure BOSS Net can reliably measure parameters of most of the commonly observed objects within this parameter space. We also update APOGEE Net to achieve a comparable performance in the near-IR regime. The resulting models provide a robust tool for measuring stellar evolutionary states, and, in turn, enable characterization of the star-forming history of the Galaxy.
更多查看译文
关键词
Spectroscopy,Fundamental parameters of stars,Surveys
AI 理解论文
溯源树
样例
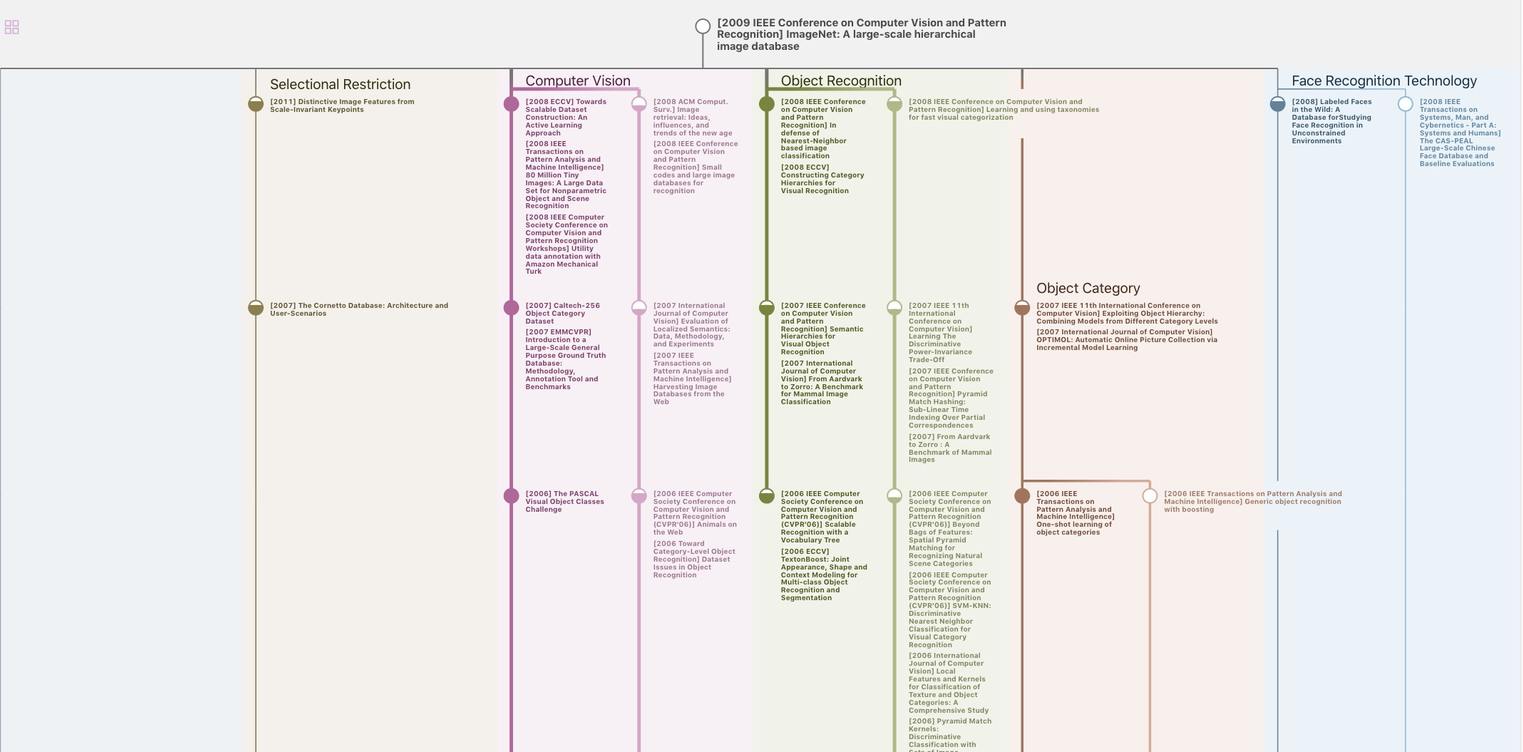
生成溯源树,研究论文发展脉络
Chat Paper
正在生成论文摘要