A graph-optimized deep learning framework for recognition of Barrett’s esophagus and reflux esophagitis
Multimedia Tools and Applications(2024)
摘要
Barrett’s esophagus (BE) recognition is essential for the diagnosis of esophageal adenocarcinoma (EAC), a highly fatal disease when left untreated at earlier stages of cancer development. General endoscopists often face difficulty in distinguishing BE from reflux esophagitis (RE) due to their resemblance in endoscopic images. This paper proposes a novel framework for the diagnosis of BE and RE in endoscopic images, leveraging deep learning techniques. The proposed framework begins with a localization network that removes interference information and enhances image contrast using histogram equalization. Subsequently, a graph-optimized deep learning (GODL) model is designed for the few-shot classification task. This model consists of two branches: a convolutional neural network (CNN) classification part, incorporating a CNN module for feature representation, and an SVM classifier for classification. Additionally, a graph neural network (GNN) branch is included to capture sample relations and utilize them effectively. The proposed model is trained using a meta-learning strategy and evaluated on endoscopic images obtained from both the Xiangya Hospital private dataset and the Hyper-Kvasir dataset. Experimental results demonstrate that the proposed model achieves a 4-classification recognition accuracy of 93.0%, with macro-precision, macro-recall, and macro-F scores of 93.5%, 92.9%, and 93.2%, respectively. Importantly, our model’s performance is comparable to that of experienced endoscopists. These promising results suggest that the proposed GODL model can serve as a valuable supportive tool for detecting esophageal diseases in clinical settings.
更多查看译文
关键词
Deep learning(DL),Graph neural network(GNN),Barrett’s esophagus(BE),Reflux esophagitis(RE)
AI 理解论文
溯源树
样例
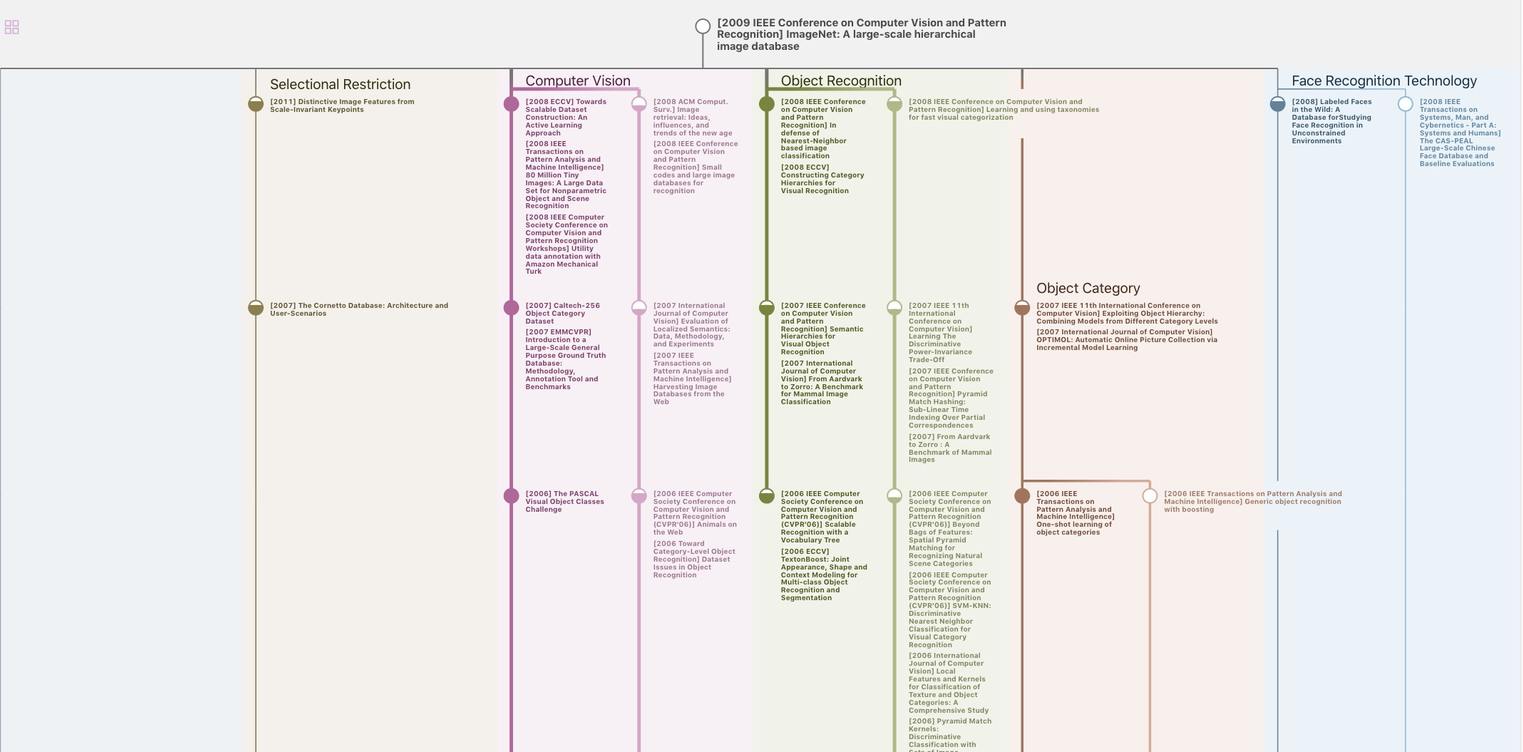
生成溯源树,研究论文发展脉络
Chat Paper
正在生成论文摘要