Diabetes Prediction using Enhanced and Optimized DRL-CNN Approaches
2024 21st Learning and Technology Conference (L&T)(2024)
摘要
This study develops an advanced automated prediction system using Machine Learning (ML) techniques to identify diabetes early. The research employs the WBSMOTE method for data preprocessing, addresses class imbalances with the WB-SMOTE approach, and introduces two novel classification methods, DRL-CNN and DRL-OCNN. Reliable datasets from sources like The Data World repository, PIMA Indian Diabetes (PIDD) dataset, and Real Time (RT) Diabetes Dataset are used, with an emphasis on data consistency and feature relevance. If we examine these datasets, it contains general attributes and no internal specific attributes related to diabetes is used. So, prediction results will be what type of diabetes we won’t get any specific characteristics and risk factors. Evaluation is based on Accuracy, Precision, and Recall criteria. The DRL-OCNN model, integrating SGD optimization and Delta Rule Learning, outperforms other ML models with a accuracy of 96%, precision of 0.93 and recall of 0.91, demonstrating its potential for accurate early diabetes identification and classification.
更多查看译文
关键词
Diabetes,Machine learning,DRL-CNN,DRL-OCNN,WB-SMOTE
AI 理解论文
溯源树
样例
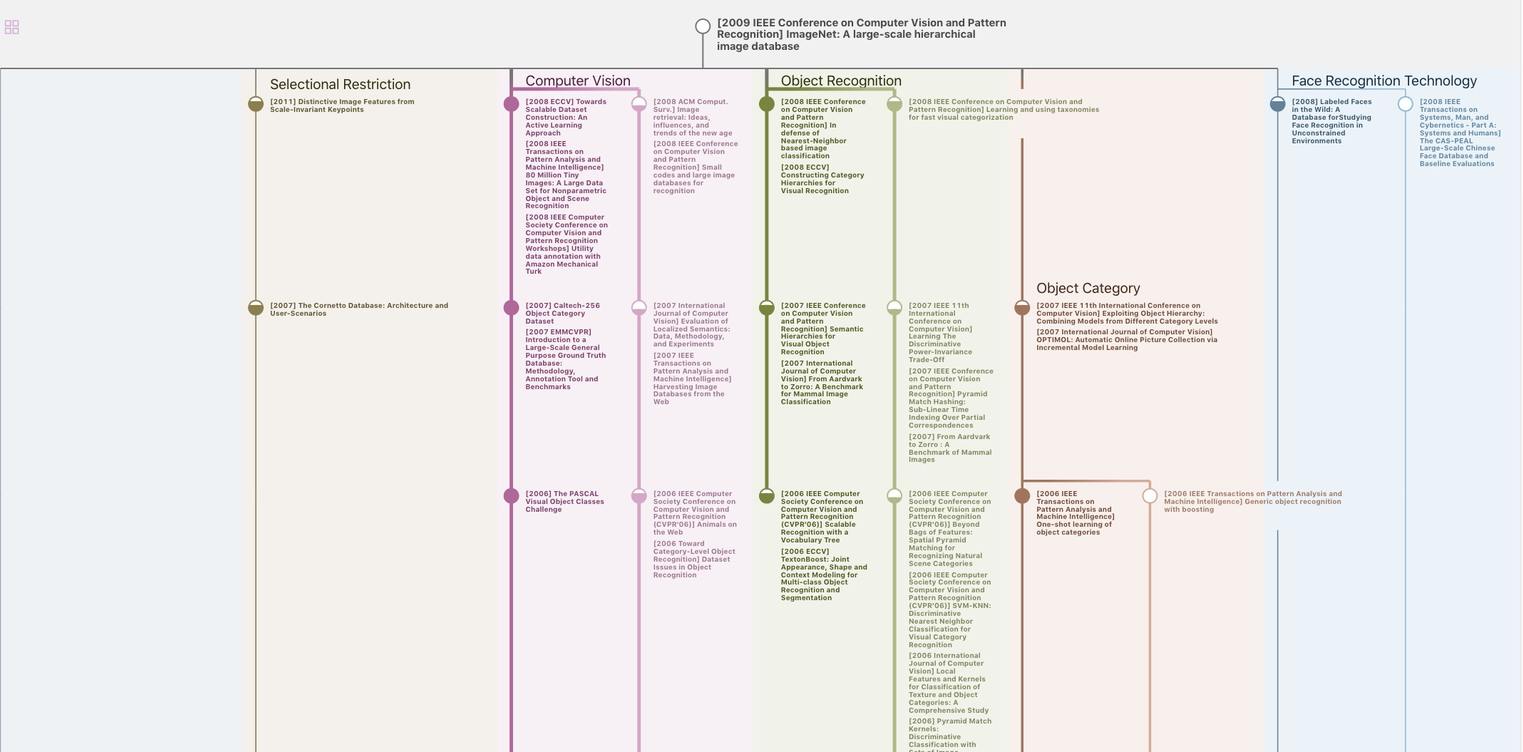
生成溯源树,研究论文发展脉络
Chat Paper
正在生成论文摘要