Learning-Aided Evolutionary Search and Selection for Scaling-up Constrained Multiobjective Optimization
IEEE Transactions on Evolutionary Computation(2024)
摘要
The existing constrained multiobjective evolutionary algorithms (CMOEAs) still have great room for improvement in balancing populations convergence, diversity and feasibility on complex constrained multiobjective optimization problems (CMOPs). Besides, their effectiveness deteriorates dramatically when facing the CMOPs with scaling-up objective space or search space. We are thus motivated to design a learning-aided CMOEA with promising problem-solving ability and scalability for various CMOPs. In the proposed solver, two learning models are respectively trained online on constrained-ignored task and feasibility-first task, which are then used to learn the two improvement-based vectors for enhancing the search by differential evolution. In addition, the union population of parent and child solutions is divided into multiple subsets with a hierarchical clustering based on cosine similarity. A comprehensive indicator, considering objective-based performance and constraint violation degree of a solution, is developed to select the representative solution from each cluster. The effectiveness of the proposed optimizer is verified by solving the CMOPs with various irregular Pareto fronts, the number of objectives ranging from 2 to 15, and the dimensionality of search space scaling up to 1000.
更多查看译文
关键词
Evolutionary algorithm,Constrained Multiobjective Optimization,Learning-aided Search and Selection
AI 理解论文
溯源树
样例
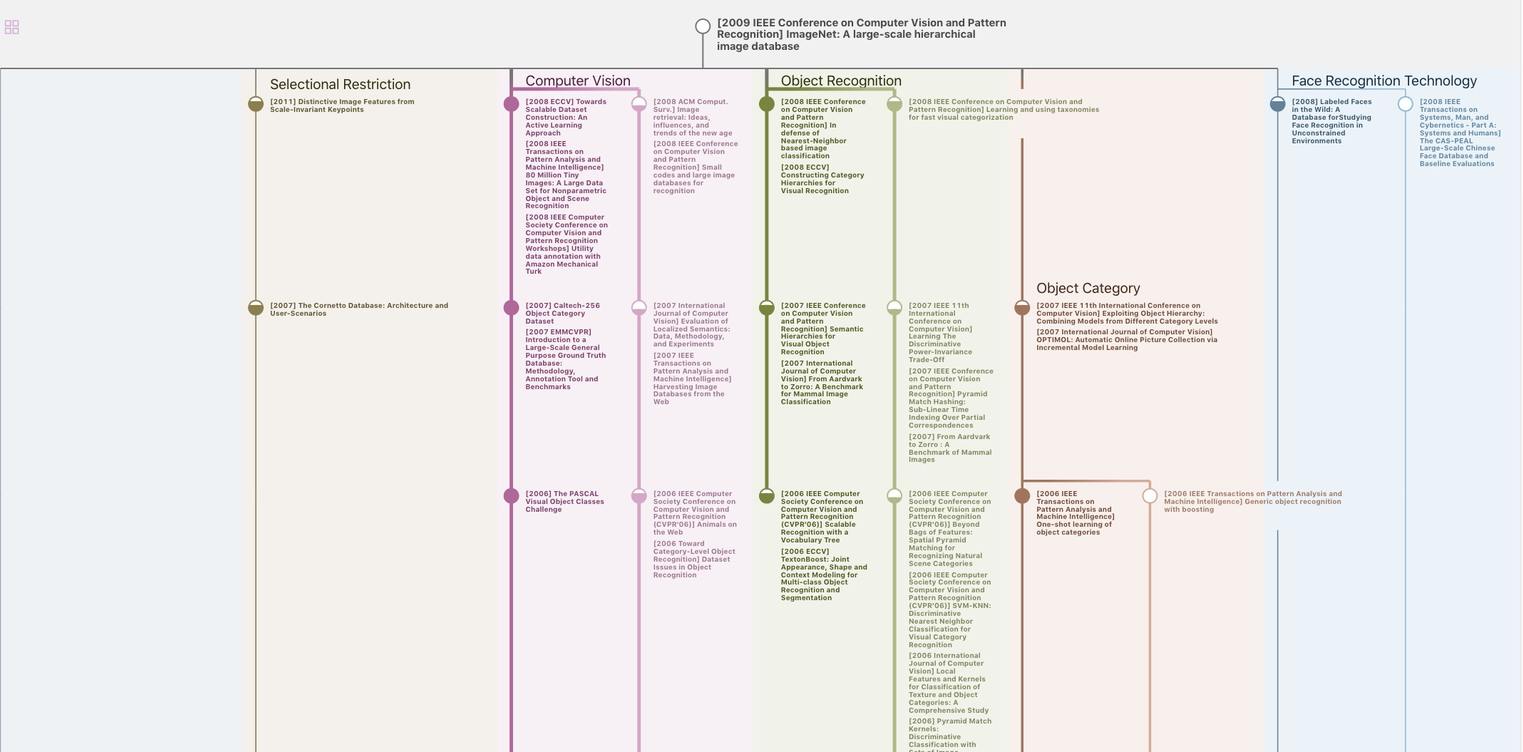
生成溯源树,研究论文发展脉络
Chat Paper
正在生成论文摘要