Joint Dual Learning with Mutual Information Maximization for Natural Language Understanding and Generation in Dialogues
IEEE/ACM Transactions on Audio, Speech, and Language Processing(2024)
摘要
Modular conversational systems heavily rely on the performance of their natural language understanding (NLU) and natural language generation (NLG) components. NLU focuses on extracting core semantic concepts from input texts, while NLG constructs coherent sentences based on these extracted semantics. Inspired by information theory in digital communication, we introduce a one-way communication model that mirrors human conversations, comprising two distinct phases: (1) the conversion of thoughts into messages, similar to NLG, and (2) the comprehension of received messages, similar to NLU. This paper presents a novel algorithm that trains NLU and NLG collaboratively by concatenating their models and maximizing mutual information between inputs and outputs. This approach efficiently facilitates the transmission of semantics, leading to enhanced learning performance for both components. Our experimental results, based on three benchmark datasets, consistently demonstrate significant improvements for both NLU and NLG tasks, highlighting the practical promise of our proposed method.
更多查看译文
关键词
Dual learning,natural language understanding,natural language generation,mutual information
AI 理解论文
溯源树
样例
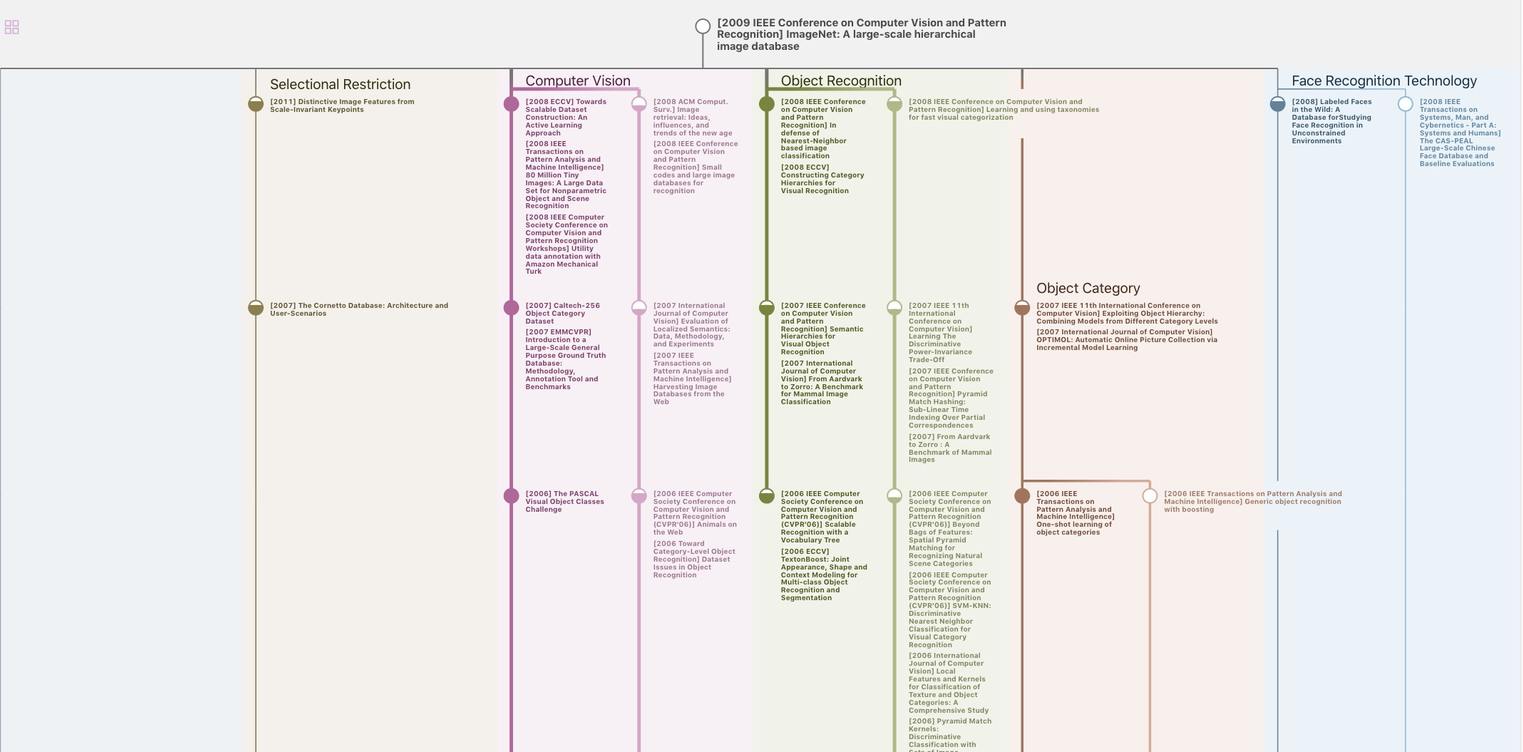
生成溯源树,研究论文发展脉络
Chat Paper
正在生成论文摘要