Feature Generation-Aided Zero-Shot Fast SAR Target Recognition With Semantic Attributes
IEEE GEOSCIENCE AND REMOTE SENSING LETTERS(2024)
摘要
A novel semantic-aided feature generative adversarial network (SFGAN) is proposed in this letter and utilized in zero-shot learning (ZSL) for fast synthetic aperture radar (SAR) automatic target recognition (ATR). By exploiting shared semantic attributes of all classes, the SFGAN is capable of directly generating and recognizing samples with sufficient discriminability and reality from feature dimension and ultimately achieves the accurate and fast recognition of unseen targets. To enhance the quality and stability of feature space, the Wasserstein distance measurement based on the semantic attributes is applied in generative adversarial network (GAN). Meanwhile, in order to improve the discriminability of generated features, a classification subnet is integrated into the network. Additionally, Gaussian mixture model (GMM) and reconstruction loss are introduced in feature generation to align the unseen features more consistent with distribution of real data. In experiments on a new self-built SAR aircraft dataset, SFGAN achieves 70.14% accuracy on unseen targets and improves the training speed by more than two times, fully demonstrating the advancement and effectiveness of the proposed method.
更多查看译文
关键词
Fast recognition,feature generation,synthetic aperture radar (SAR),zero-shot learning (ZSL)
AI 理解论文
溯源树
样例
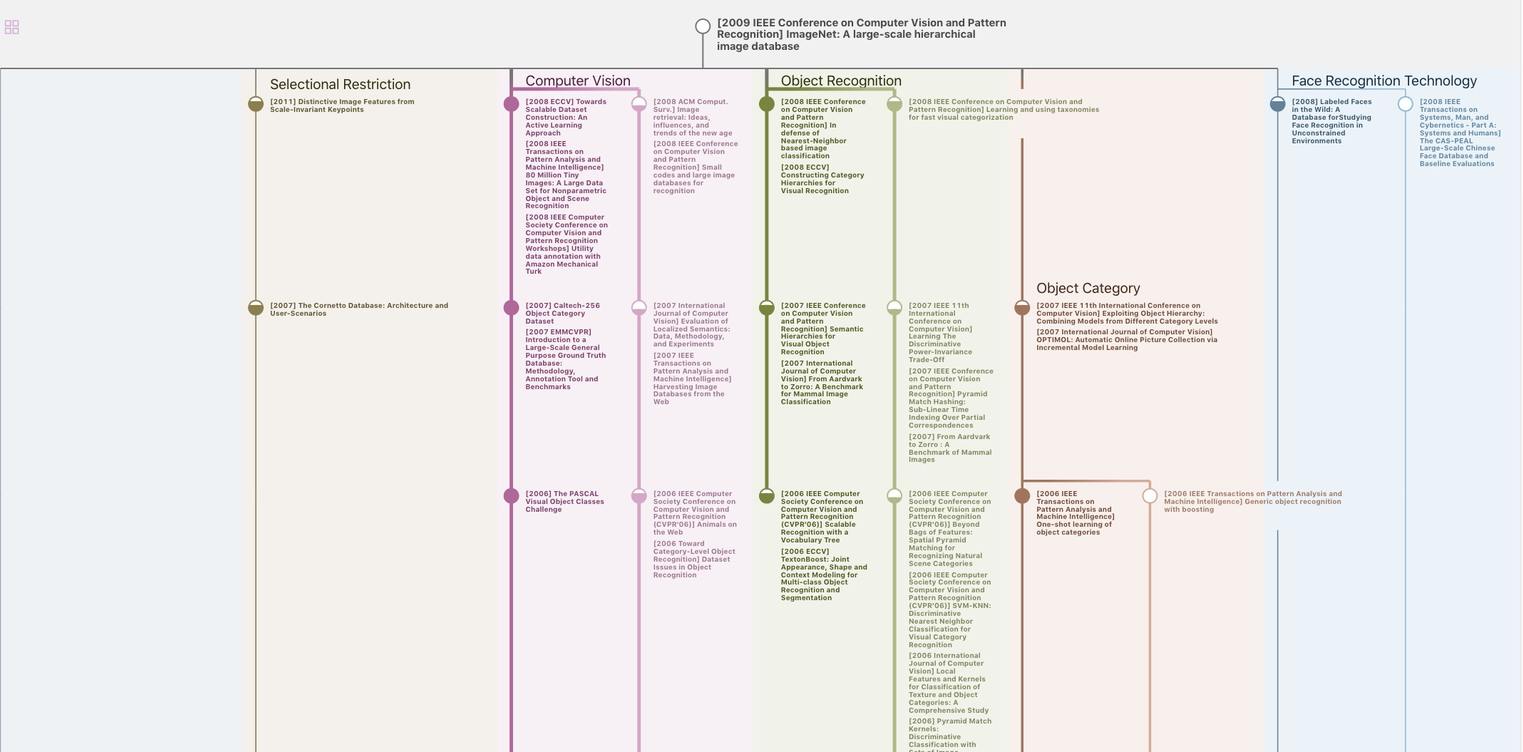
生成溯源树,研究论文发展脉络
Chat Paper
正在生成论文摘要