A Survey of Factual Consistency in Summarization from 2021 to 2023
2023 4th International Conference on Computers and Artificial Intelligence Technology (CAIT)(2023)
摘要
Factual consistency problem has become an increasingly important issue in summarization in recent years. Since 2019, researchers have continuously pointed out that there are many factual inconsistencies in the model-generated summaries. Several pieces of information may appear in the summaries that are factually inconsistent with the documents, thus hindering the further development and application of existing summarization models. With the efforts of researchers in recent years, solutions to the factual consistency problem in summarization have been greatly enriched and involved a variety of improvement schemes. Considering this rapid technical progress from 2021 to 2023, in this survey, we review the recent developments by systematically sorting out the background, main findings and major improvement methods for factual consistency in summarization. The recent works can be divided into five categories, namely factual error types, faithfulness of summarization datasets, factual consistency metrics, post-editing methods and end-to-end summarization models.
更多查看译文
关键词
natural language processing,summarization,factual consistency
AI 理解论文
溯源树
样例
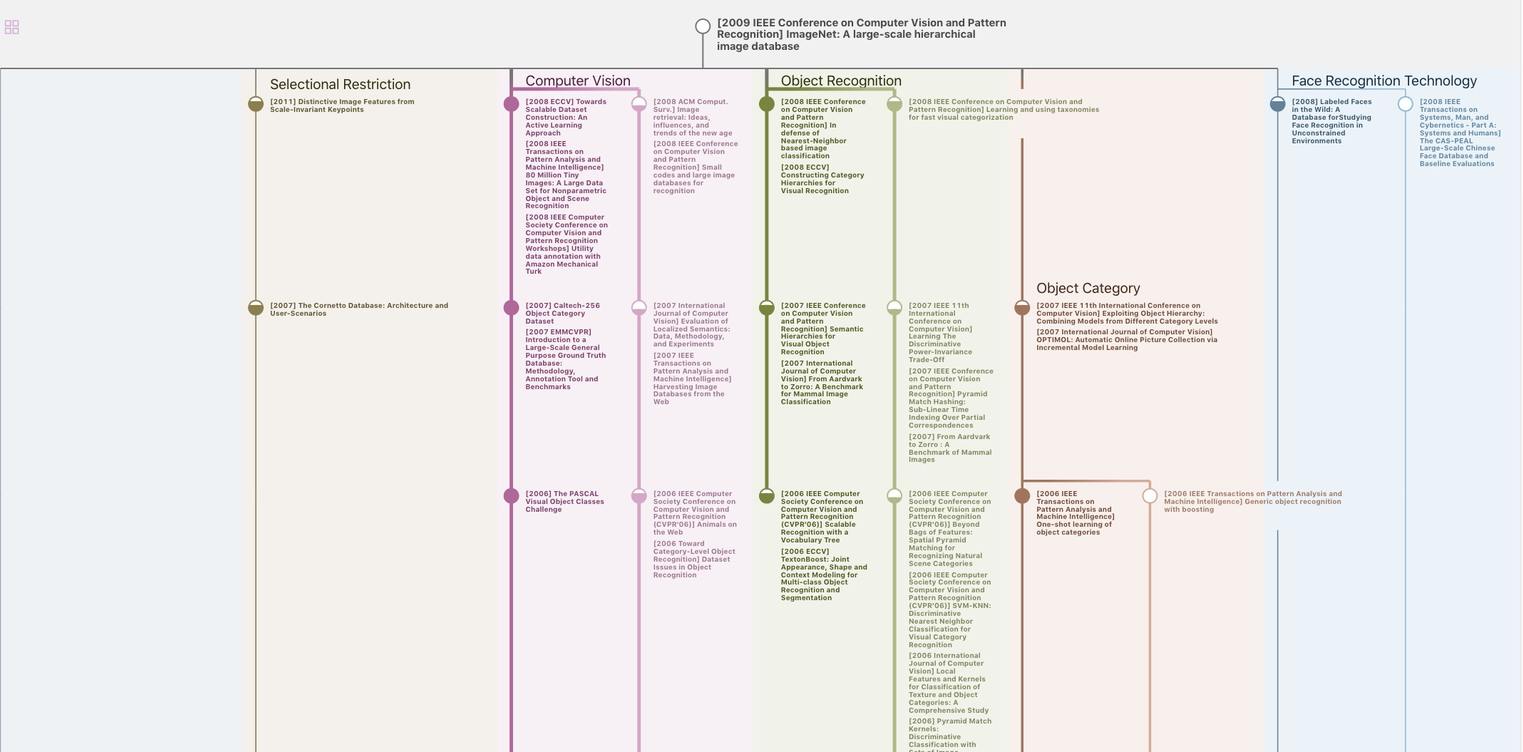
生成溯源树,研究论文发展脉络
Chat Paper
正在生成论文摘要