Causal knowledge engineering: A case study from COVID-19
arxiv(2024)
摘要
COVID-19 appeared abruptly in early 2020, requiring a rapid response amid a
context of great uncertainty. Good quality data and knowledge was initially
lacking, and many early models had to be developed with causal assumptions and
estimations built in to supplement limited data, often with no reliable
approach for identifying, validating and documenting these causal assumptions.
Our team embarked on a knowledge engineering process to develop a causal
knowledge base consisting of several causal BNs for diverse aspects of
COVID-19. The unique challenges of the setting lead to experiments with the
elicitation approach, and what emerged was a knowledge engineering method we
call Causal Knowledge Engineering (CKE). The CKE provides a structured approach
for building a causal knowledge base that can support the development of a
variety of application-specific models. Here we describe the CKE method, and
use our COVID-19 work as a case study to provide a detailed discussion and
analysis of the method.
更多查看译文
AI 理解论文
溯源树
样例
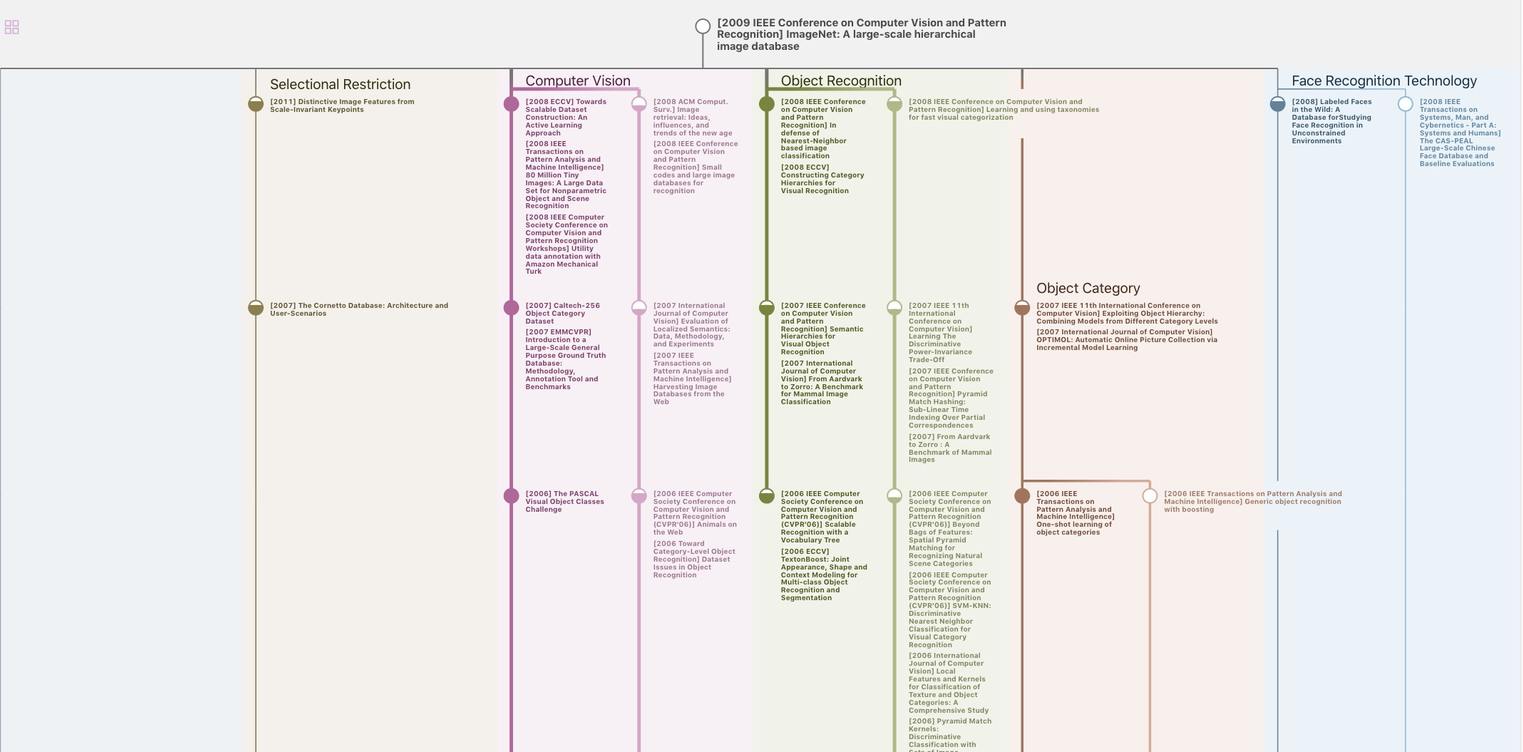
生成溯源树,研究论文发展脉络
Chat Paper
正在生成论文摘要