Speech-Based Alzheimer's Disease Classification System with Noise-Resilient Features Optimization
2023 31ST IRISH CONFERENCE ON ARTIFICIAL INTELLIGENCE AND COGNITIVE SCIENCE, AICS(2023)
摘要
Alzheimer's disease is a severe neurological disorder having a major influence on a substantial portion of the population. The prompt detection of this condition is crucial, and speech analysis may play a crucial role in facilitating efficient treatment and care. The main aim of this research has been to investigate the significance of timely identification of speech signal abnormalities associated with Alzheimer's disease in order to provide effective therapy interventions and improve disease management. The study used the Mel Frequency Cepstral Coefficients (MFCC) framework, a well recognized technique for feature extraction known for its versatility across several domains. This research introduces an innovative approach that utilizes both individuals diagnosed with dementia and control participants to detect two unique types of cognitive impairment via the analysis of speech signals. The approach used in this work involves the extraction of acoustic properties from pre-processed speech data obtained from the Pitt Corpus of Dementia Bank. This is achieved by using several feature sets, which include a combination of MFCC, prosodic features, and statistical features. This study examines the attributes of optimum feature optimization in actual and noise-enhanced speech environments using machine learning techniques. The integration of MFCC,Statistical and prosodic features has shown remarkable outcomes, exhibiting a superior accuracy rate of 98.3%. This surpasses the performance of other feature combinations when using the Random Forest classifier.
更多查看译文
关键词
Alzheimer's Disease,MFCC features,Prosodic Feature,Statistical Feature,Machine Learning,Classification
AI 理解论文
溯源树
样例
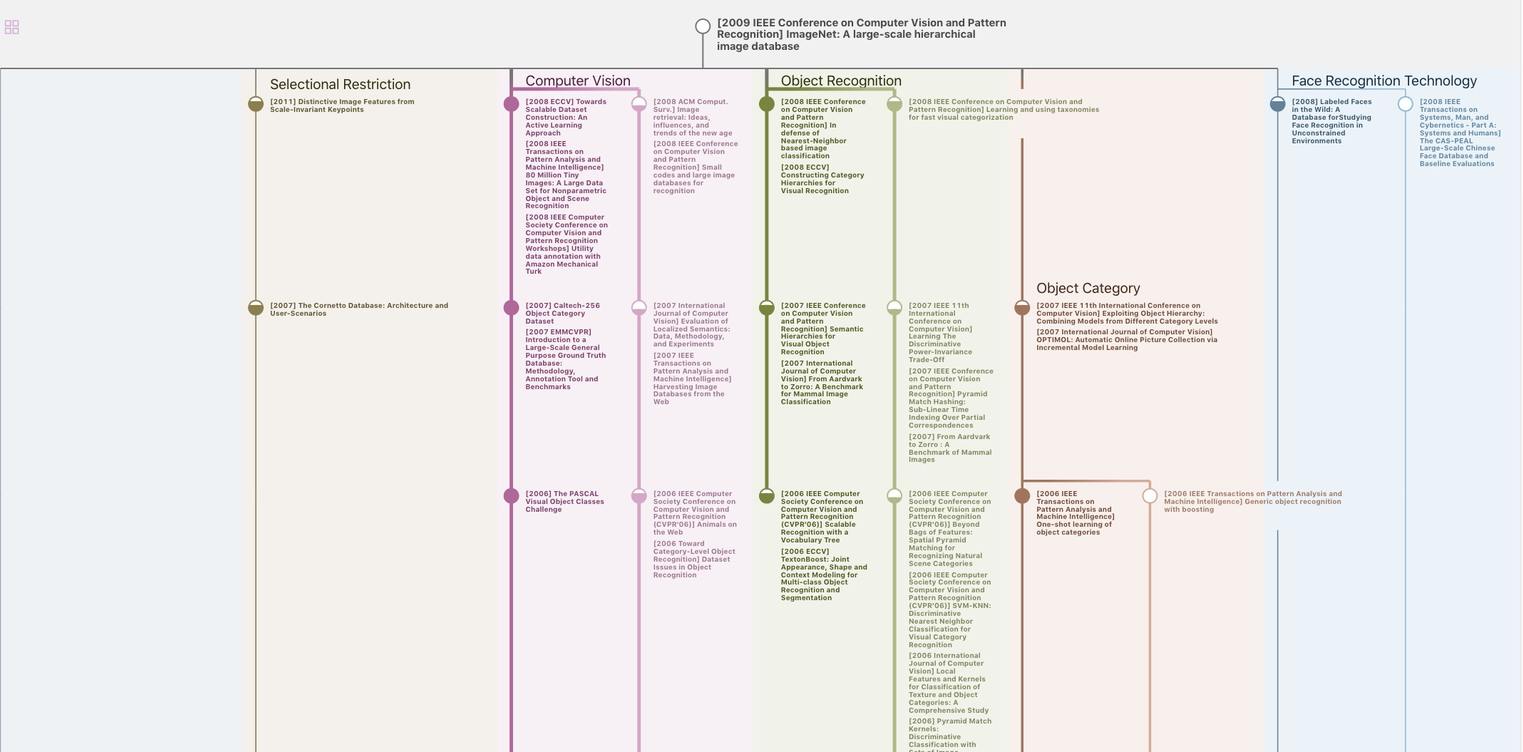
生成溯源树,研究论文发展脉络
Chat Paper
正在生成论文摘要