Network Slice Traffic Demand Prediction for Slice Mobility Management.
International Conference on Artificial Intelligence in Information and Communication(2024)
摘要
5G revolutionizes connectivity with network slicing, but non-uniform slice deployment poses challenges. Efficient slice handover mechanisms and predictive learning offer solutions. We propose Long Short-Term Memory (LSTM) based prediction of network slice traffic demands for proactive resource management to resolve challenges of mobility management and non-uniform slice deployment within a registration area. Thereby, allowing seamless service provision throughout the registration area. Our proposed model shows excellent results in predicting the number of received requests for all available slices. These results are then utilized to deduce the average slice demand for each service in the network. The obtained results are beneficial for configuring the slice resources proactively in order to provide seamless service to mobile users throughout the registration area, regardless of the non-uniformity in slice deployment among different tracking areas.
更多查看译文
AI 理解论文
溯源树
样例
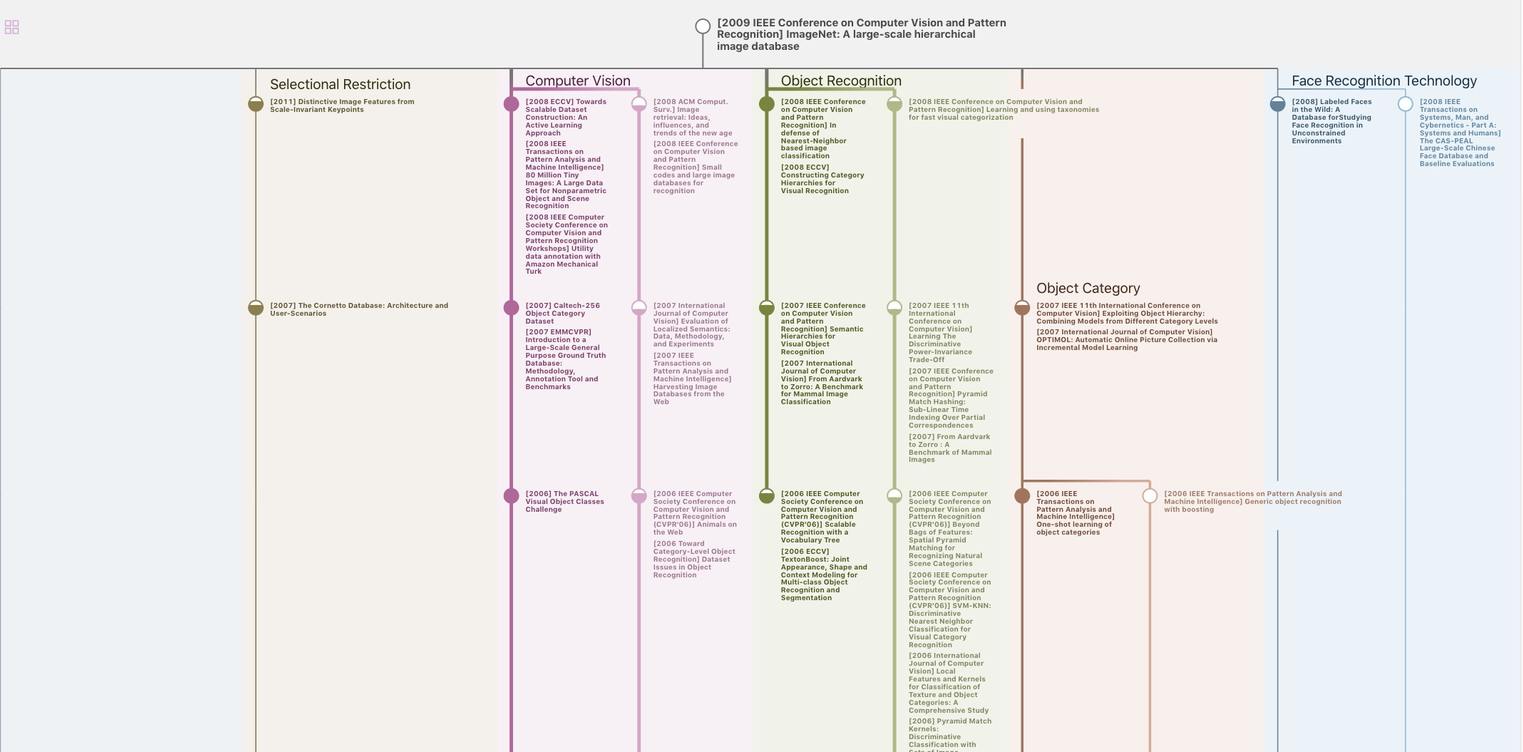
生成溯源树,研究论文发展脉络
Chat Paper
正在生成论文摘要