Deep Learning-based Point Cloud Geometry Coding with Attention Models.
ISM(2023)
摘要
Recent advancements in Deep Learning (DL)-based architectures have demonstrated that integrating attention models can substantially enhance the performance across various tasks, including computer vision and visual coding. In accordance with this trend, this paper proposes a framework for incorporating attention models into DL-based Point Cloud (PC) geometry coding, namely JPEG Pleno PC Coding (JPEG PCC) and a definition of its key architectural design options. Experimental results show that the integration of attention models in JPEG PCC can provide a trade-off between compression and complexity, notably compression gains at the cost of an increase in model complexity and number of parameters. If the priority is on compression gains, the use of attention models can lead to a rate reduction up to 5.7%, for both the PSNR DI and PSNR D2 geometry quality metrics, at the cost of a 47% increase on the number of parameters and a 15.1% increase on the Floating-Point Operations (FLOPs) complexity. This obtained trade-off depends on the attention model integration configuration which can be defined depending on the application requirements.
更多查看译文
关键词
point cloud coding,learning-based coding,attention models,JPEG PCC standard
AI 理解论文
溯源树
样例
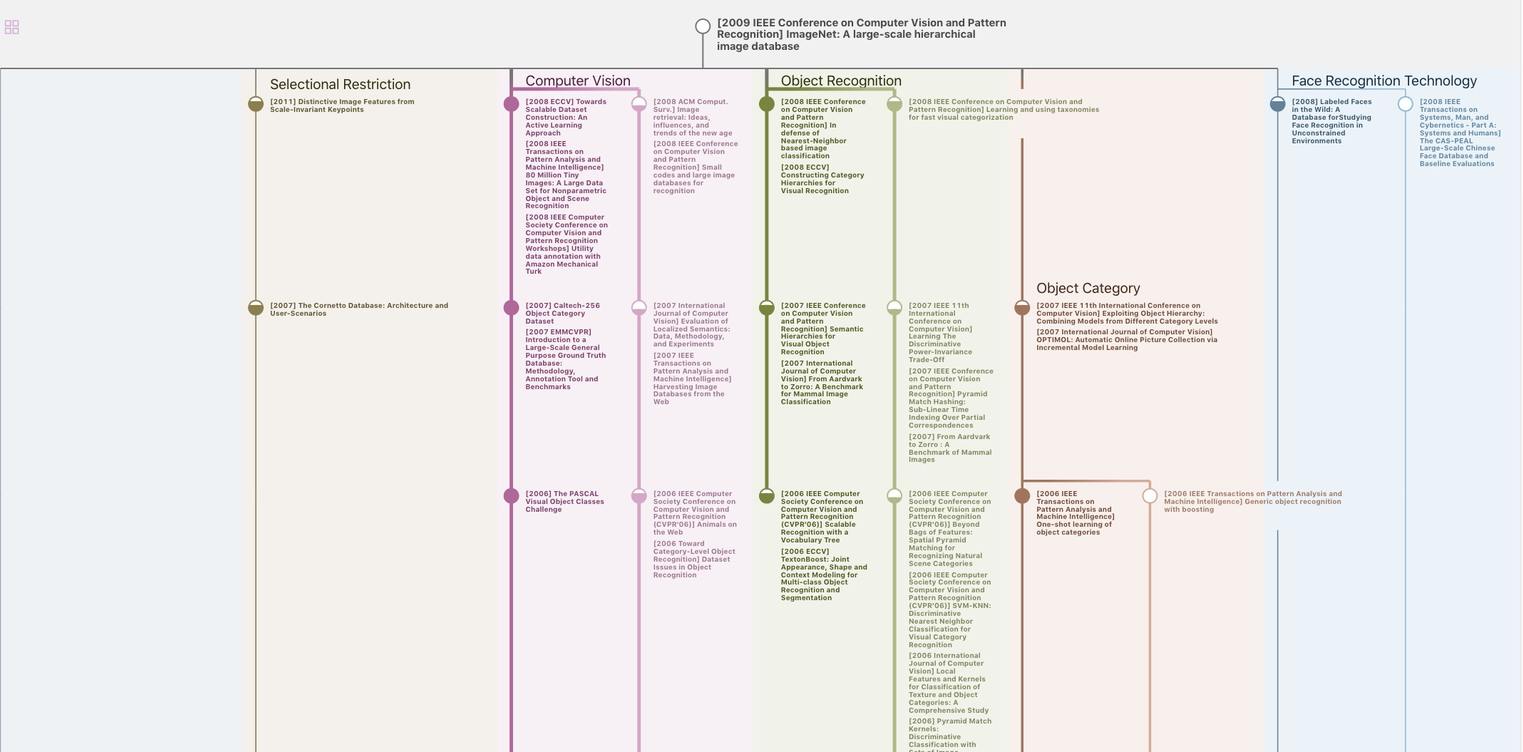
生成溯源树,研究论文发展脉络
Chat Paper
正在生成论文摘要