A Forward-Backward Simheuristic for the Stochastic Capacitated Dispersion Problem
MATHEMATICS(2024)
摘要
In an effort to balance the distribution of services across a given territory, dispersion and diversity models typically aim to maximize the minimum distance between any pair of facilities. Specifically, in the capacitated dispersion problem (CDP), each facility has an associated capacity or level of service, and the objective is to select a set of facilities so that the minimum distance between any pair of them (dispersion) is maximized, while ensuring a user-defined level of service. This problem can be formulated as a linear integer model, where the sum of the capacities of the selected facilities must match or exceed the total demand in the network. Real-life applications often necessitate considering the levels of uncertainty affecting the capacity of the nodes. Failure to account for this uncertainty could lead to low-quality or infeasible solutions in practical scenarios. However, research addressing the stochastic version of the CDP is scarce. This paper introduces two models for the CDP with stochastic capacities, incorporating soft constraints and penalty costs for violating the total capacity constraint. The first model includes a probabilistic constraint to ensure the required level of service with a certain probability, while the second model introduces a soft constraint with penalty costs for violations. To solve both variants of the model, a forward-backward simheuristic algorithm is proposed. Our approach combines a metaheuristic algorithm with Monte Carlo simulation, enabling the efficient handling of the random behavior of node capacities and obtaining reliable solutions regardless of their probability distribution.
更多查看译文
关键词
dispersion problem,stochastic optimization problems,metaheuristics,simulation,90-10
AI 理解论文
溯源树
样例
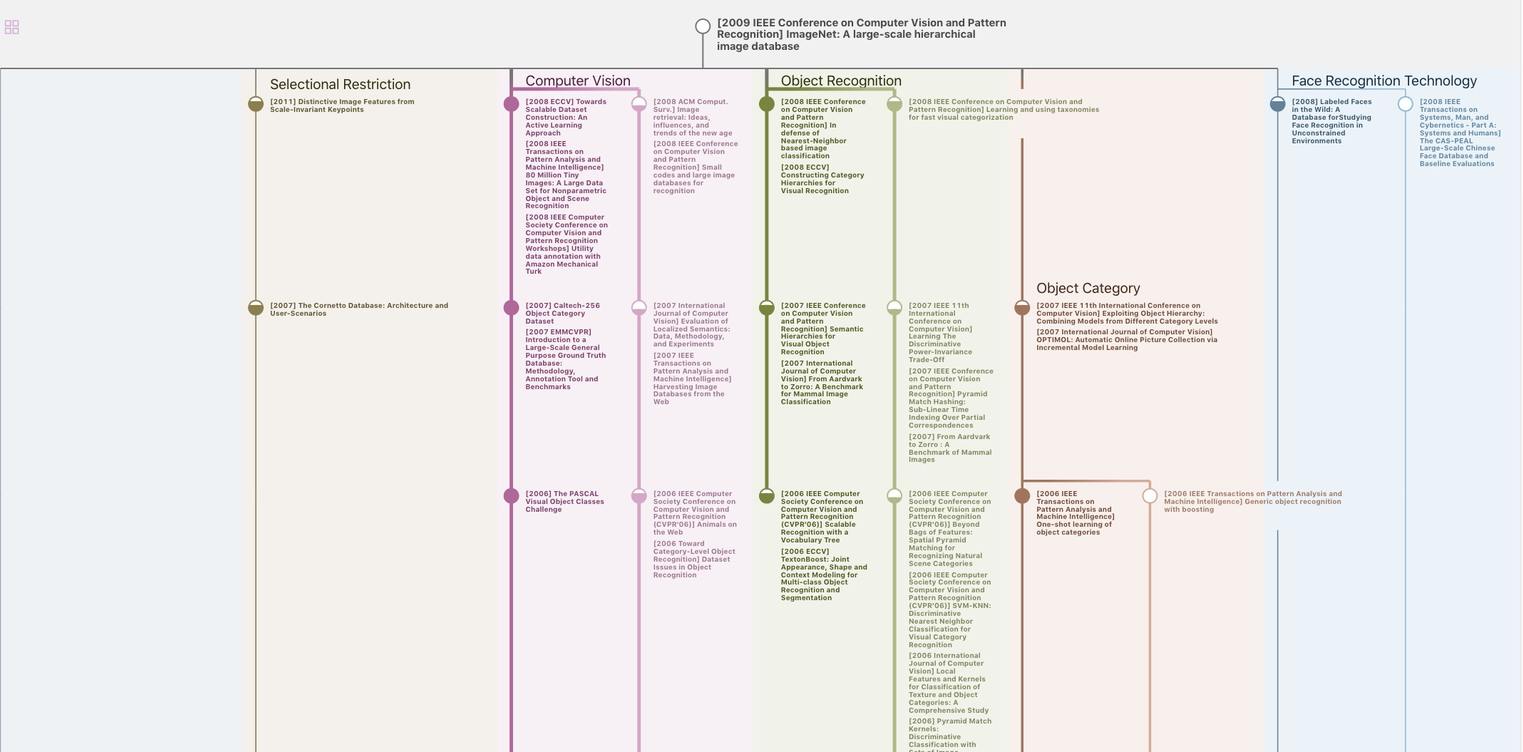
生成溯源树,研究论文发展脉络
Chat Paper
正在生成论文摘要