Contribution of low support association rules in understanding the mined knowledge
2024 International Conference on Artificial Intelligence, Computer, Data Sciences and Applications (ACDSA)(2024)
摘要
In the realm of numerical association rule mining using population-based nature-inspired algorithms, the evaluation of results usually depends on a crucial metric – a fitness value crafted from weighted support and confidence measures. Traditionally, this metric separates the mined rules into a spectrum, ranging from the low-quality, low-fitness rules to the peaks of their high-quality, high-fitness counterparts. Until recently, little attention has been directed towards low-fitness rules, as users engage predominantly with high-quality rules. However, in our pioneering research, we go into the enigmatic realm of low-support, more precisely, the often-overlooked association rules. Through meticulous analysis of our rule repository, we seek to uncover the profound insights concealed within these seemingly unremarkable rules. Our method was applied to the Abalone UCI ML dataset, where three association rules, mined using the universal association rule miner based on evolutionary algorithms, were taken into consideration, i.e., the AR-1 was mined in the first generation, the AR-2 mined in the last generation, and the AR-3 mined at the moment before the phenomenon of the disappearing features has arisen. Significantly, the analysis of the AR-3 revealed that the association rules of the lower support can contribute to understanding the mined knowledge.
更多查看译文
关键词
association rule mining,data mining,evolutionary algorithms,numerical association rule mining,support
AI 理解论文
溯源树
样例
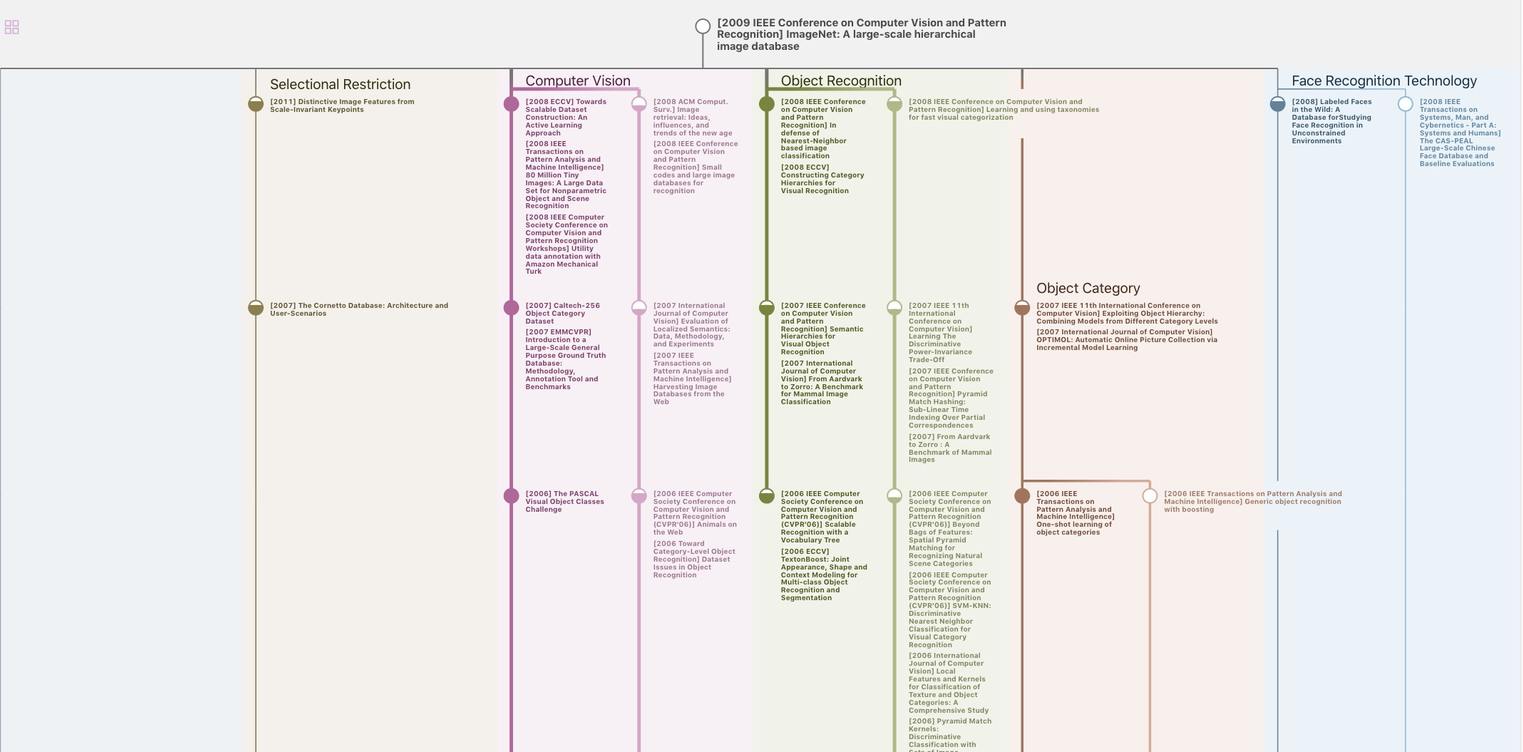
生成溯源树,研究论文发展脉络
Chat Paper
正在生成论文摘要