Design and Implementation of a High-Speed Low-Power K -Nearest-Neighbors-based Algorithm for Detecting Micro-Single-Event-Latchups
IEEE Transactions on Nuclear Science(2024)
摘要
In an irradiation environment such as space, Single-Event-Latchup (SEL) is a radiation effect that may cause an abnormal high-current state in semiconductor devices, particularly in commercial-off-the-shelf (COTS) integrated circuits (ICs). On the other hand, the micro-SEL (μ-SEL) is lower-current and more localized, and therefore more difficult to detect. Recent reported work embodying machine learning to detect μ-SELs is only partially successful (70% accuracy), yet non-real-time detection (50 s) and with high power dissipation (82-132 mW). In this paper, we propose a novel software-hardware co-optimized high-speed low-power
k
-nearest-neighbors-based (KNN-based) algorithm to protect a COTS IC against μ-SELs. Further, to obtain the highest μ-SEL detection accuracy, yet with low hardware and power overhead, we propose two features of μ-SEL characteristics in addition to the reported seven. The two proposed features are very worthy – the optimum requires five features (of which two are the proposed). When implemented in a Field-Programmable Gate Array (FPGA) clocked at 10 MHz, our prototype achieves high (95%) μ-SEL detection accuracy, yet real-time (110 μs) detection and low (47 mW) power dissipation – equivalent to 36% improved accuracy, yet ~450,000x faster detection and 43%-64% lower power dissipation over the reported work. This renders our proposed approach appropriate for power-constrained space applications.
更多查看译文
关键词
Anomaly Detection,Field-Programmable Gate Array (FPGA),K-Nearest Neighbors (KNN),Micro-Single-Event-Latchup (μ-SEL),Machine Learning (ML),Space Application
AI 理解论文
溯源树
样例
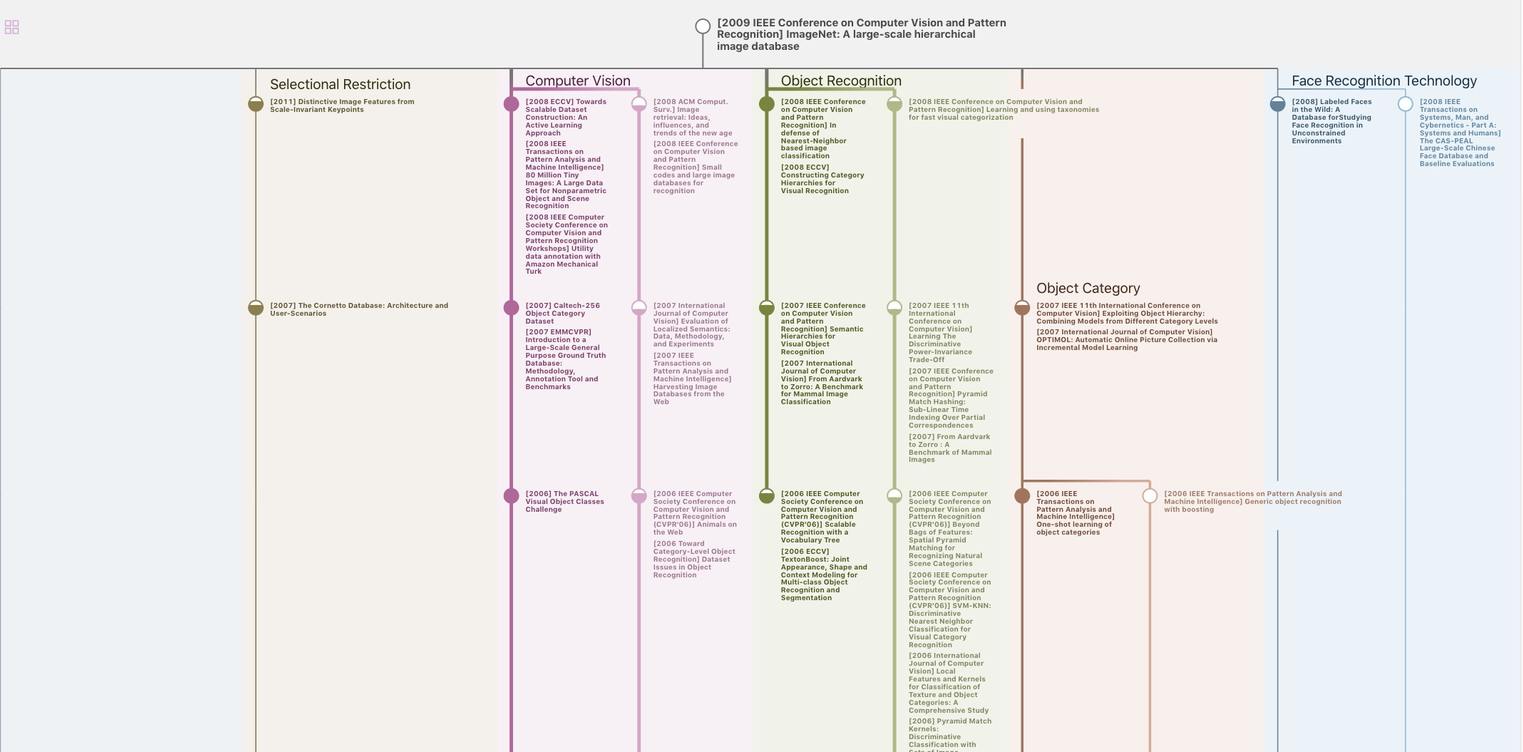
生成溯源树,研究论文发展脉络
Chat Paper
正在生成论文摘要