A Hot Spot Identification Approach for Photovoltaic Module Based on Enhanced U-Net With Squeeze-and-Excitation and VGG19
IEEE TRANSACTIONS ON INSTRUMENTATION AND MEASUREMENT(2024)
摘要
At present, hot spot identification methods for photovoltaic (PV) modules are difficult to accurately characterize the size and location of the hot spots, which brings a challenge to timely handling of the fault. To solve the problem, an enhanced U-Net with visual geometry group-19 (VGG19) and squeeze-and-excitation (SVU-Net) is proposed to achieve accurate identification at the granularity level of pixels. Based on the U-Net architecture, the convolutional layer of VGG19 is used as an encoder to extract feature information of PV module hot spot images. Based on skip connections, detailed information are gradually recovered through the upsampling of the decoder. Specifically, the thermal infrared image of the PV module is processed by the Gaussian blur and image sharpening method to improve the quality of the hot spot image. Then, based on the U-Net architecture, the convolutional layer of VGG19 is used as the encoder to extract low-level and high-level features through a series of convolutional layers and pooling layers. At the same time, a squeeze-and-excitation block is added to the $224 \times 224 \times 64$ convolution in the VGG19 encoder for extracting global features. In addition, to better distinguish the differences between PV hot spots and normal regions, dilated convolutions are used to increase the receptive field and enhance the understanding of the structure and semantics in the image, thereby capturing the surrounding context information of the hot spots. The experimental results show that the accuracy and mean intersection over union (MIoU) of PV hot spots are 98.37% and 91.93%, respectively, by using the proposed method.
更多查看译文
关键词
Hot spot identification,improved U-Net method,photovoltaic (PV) modules
AI 理解论文
溯源树
样例
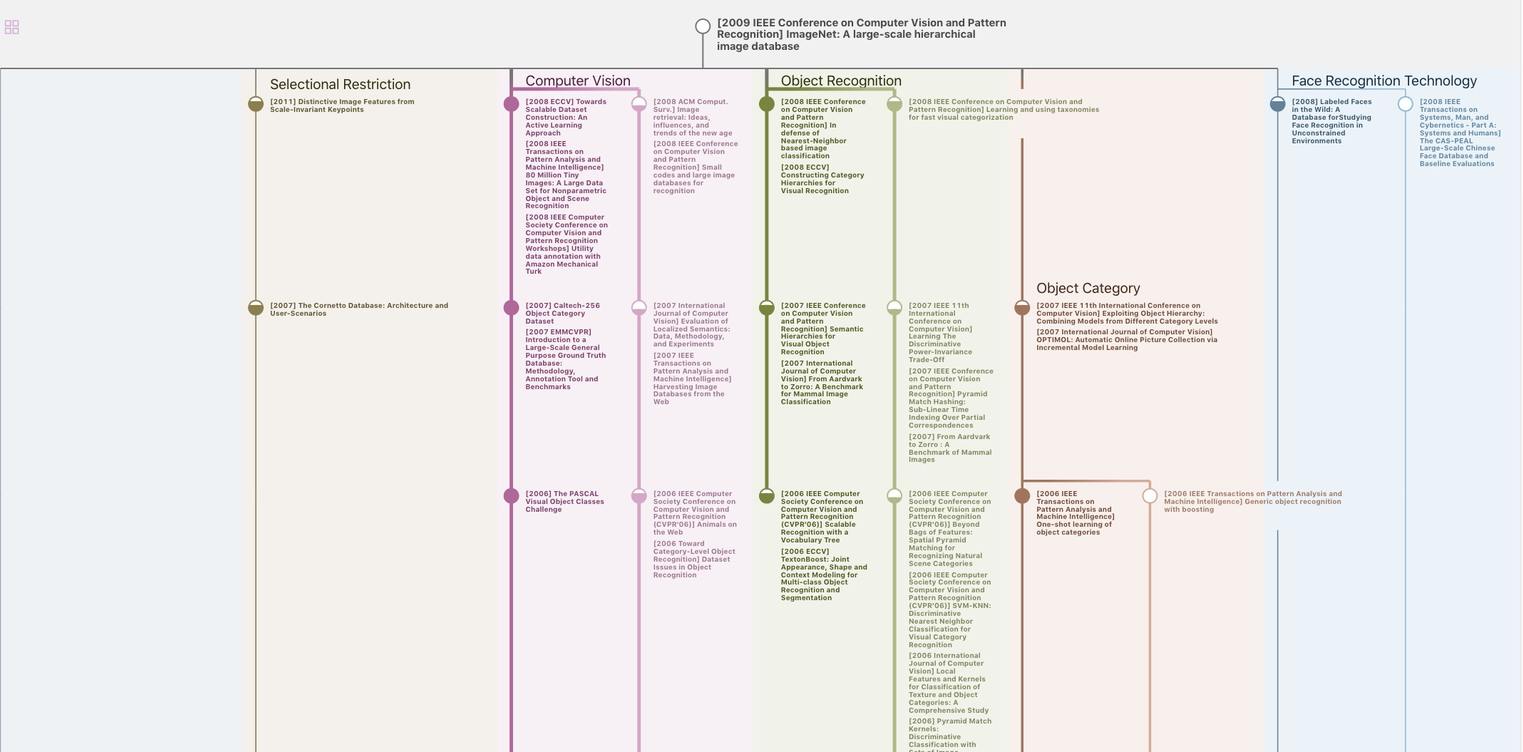
生成溯源树,研究论文发展脉络
Chat Paper
正在生成论文摘要