A Novel Energy-Efficient Approach Based On Clustering Using Grey Prediction in WSNs for IoT Infrastructures
IEEE Internet of Things Journal(2024)
摘要
Wireless Sensor Networks (WSNs), which provide perception services for the Internet of Things (IoT) infrastructure, usually suffer from constrained energy resources. However, the fact that the data collected by WSNs often exhibit spatial-temporal correlation leads to the waste of energy. In addition, load imbalance among sensor nodes also makes energy efficiency low. To this end, a novel Energy-efficient approach based on Clustering using Grey Prediction (ECGP) is proposed in this paper. To be specific, a novel Dual-end Data Prediction Mechanism (DDPM) is presented based on the grey prediction model to cut down data redundancy. Furthermore, the prediction process of the grey model, namely a dynamic and fixed size prediction queue scheme, is optimized to enhance the prediction accuracy. A novel Energy-Distance Factor (EDF) and a novel Dual-Threshold-based Critical Condition (DTCC) are proposed with the aim of realizing load balance and alleviating the challenge resulted from random events occurrence. Finally, extensive experimental simulations have been carried out to demonstrate the energy efficiency of ECGP. It is compared with the classic and several latest clustering algorithms, namely, LEACH, R-LEACH, EAHA and ECPSO. The experimental results indicate that ECGP outperforms the others in terms of the network lifetime, the throughput, and the energy efficiency.
更多查看译文
关键词
Energy efficiency,data prediction,optimal cluster head selection,grey prediction model
AI 理解论文
溯源树
样例
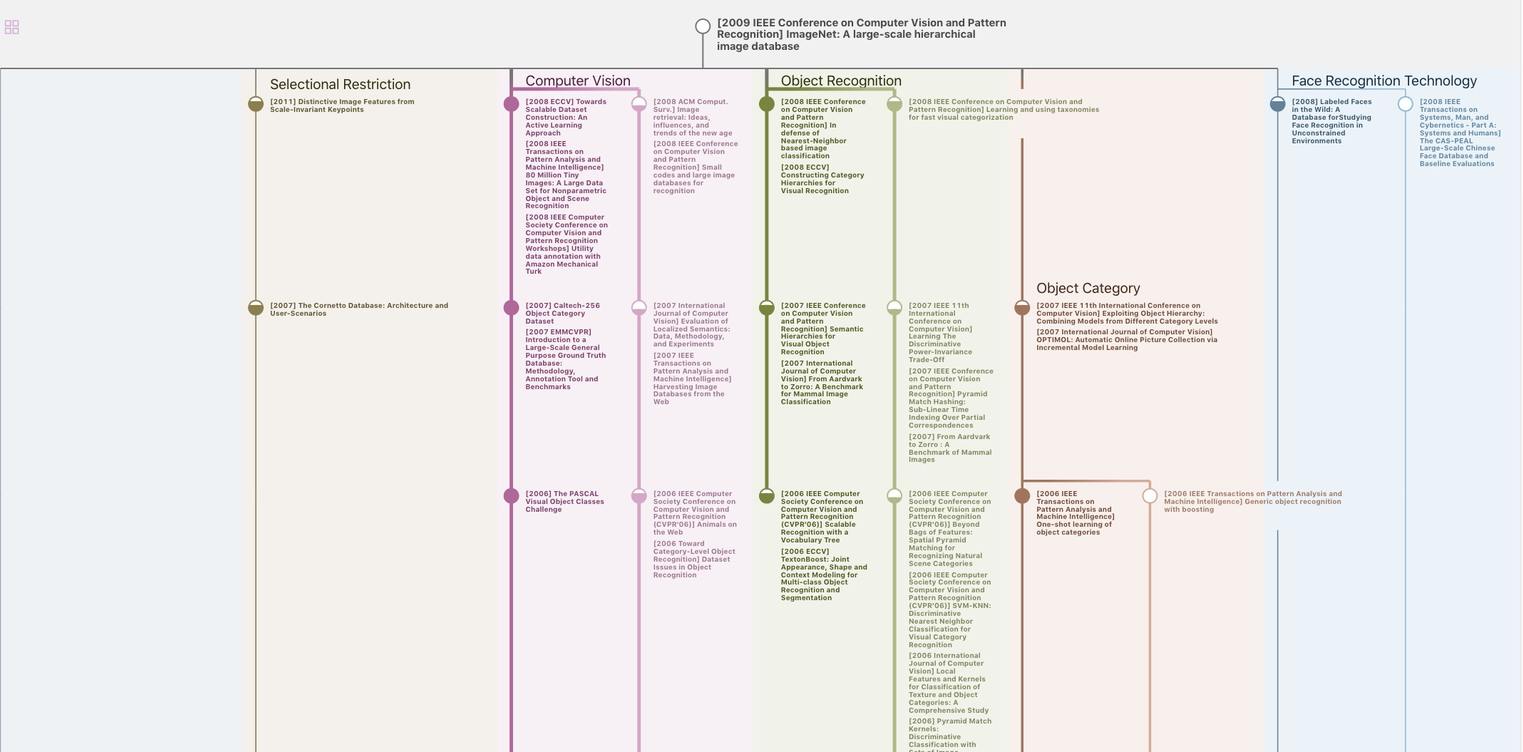
生成溯源树,研究论文发展脉络
Chat Paper
正在生成论文摘要