Robust Asymmetric Cross-Modal Hashing Retrieval With Dual Semantic Enhancement
IEEE TRANSACTIONS ON COMPUTATIONAL SOCIAL SYSTEMS(2024)
摘要
As social media faces with large amounts of data and multimodal properties, cross-modal hashing (CMH) retrieval gains extensive applications with its high efficiency and low storage consumption. However, there are two issues that hinder the performance of the existing semantics-learning-based CMH methods: 1) there exist some nonlinear relationships, noises, and outliers in the data, which may degrade the learning effectiveness of a model; and 2) the complementary relationships between the label semantics and sample semantics may be inadequately explored. To address the above two problems, a method called robust asymmetric cross-modal hashing retrieval with dual semantic enhancement (RADSE) is proposed. RADSE consists of three parts: 1) cross-modal data alignment (CDA) that applies kernel mapping and establishes a unified linear representation in the neighborhood to capture the nonlinear relationships between cross-modal data; 2) relaxed label semantic learning for robustness (RLSLR) that uses a relaxation strategy to expand label distinctiveness, and leverages & ell;(2,1) norm to enhance the robustness of the model against noise and outliers; and 3) dual semantic enhancement learning (DSEL) that learns more interrelationships between samples under the label semantic guidance to ensure the mutual enhancement of semantic information. Extensive experiments and analyses on three popular datasets demonstrate that RADSE outperforms the most existing methods in terms of mean average precision (MAP), precision recall (P-R) curves, and top-N precision curves. In the comparisons of MAP, RADSE improves by an average of 2%-3% in two retrieval tasks.
更多查看译文
关键词
Semantics,Codes,Feature extraction,Robustness,Matrix decomposition,Kernel,Training,Cross-modal hashing,dual semantic learning,label relaxation strategy,robustness
AI 理解论文
溯源树
样例
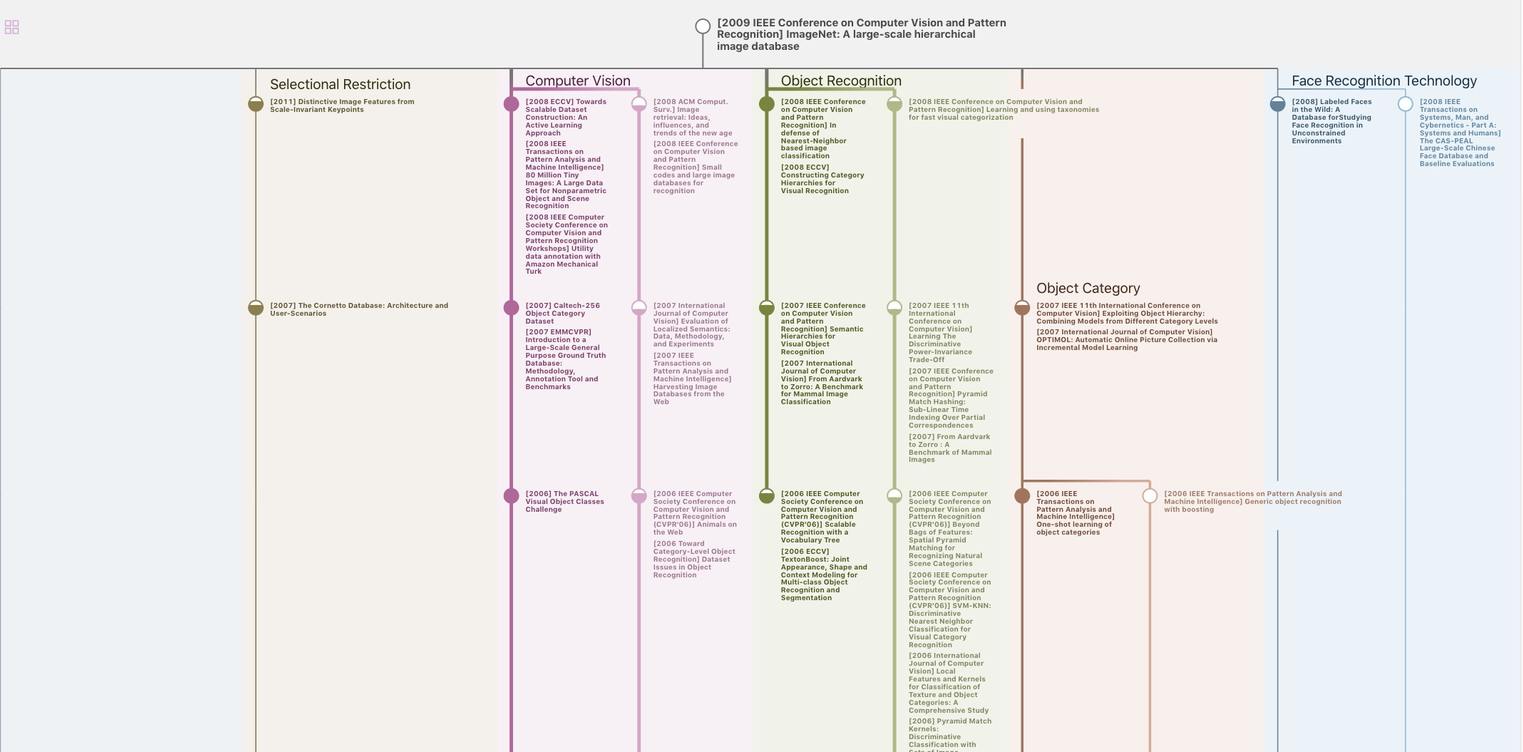
生成溯源树,研究论文发展脉络
Chat Paper
正在生成论文摘要