Group Based Privacy Preserving Incentive Mechanism for Federated Learning
2024 IEEE 3rd International Conference on Control, Instrumentation, Energy & Communication (CIEC)(2024)
摘要
Federated Learning (FL) ensures the privacy of clients’ data by allowing them to train their data by themselves and sharing only updated gradients to the server instead of the raw data. Various incentive mechanisms are proposed to boost clients’ participation in the FL process, which awards the clients based on their contribution. However, clients may selfishly minimize their computation resources to maximize profit and incentives. Some malicious clients may abort their local training process in the middle or reduce the size of training data and declare that they have trained their data completely so that they may claim maximum incentives. As a result, the quality of the trained model is reduced. Therefore, we propose a group signature-based privacy-preserving incentive mechanism in which the admin in charge of the group signing process can efficiently identify any fraudulent activity the client does. Moreover, our scheme ensures that the server can easily detect the clients that abort their process. The performance analysis shows how our algorithm is more efficient than most existing incentive algorithms in FL. Security analysis discusses that our algorithm is resistant to most of the attacks in FL and more secure than existing methods.
更多查看译文
关键词
Privacy preservation,Incentive mechanism,Federated learning,security
AI 理解论文
溯源树
样例
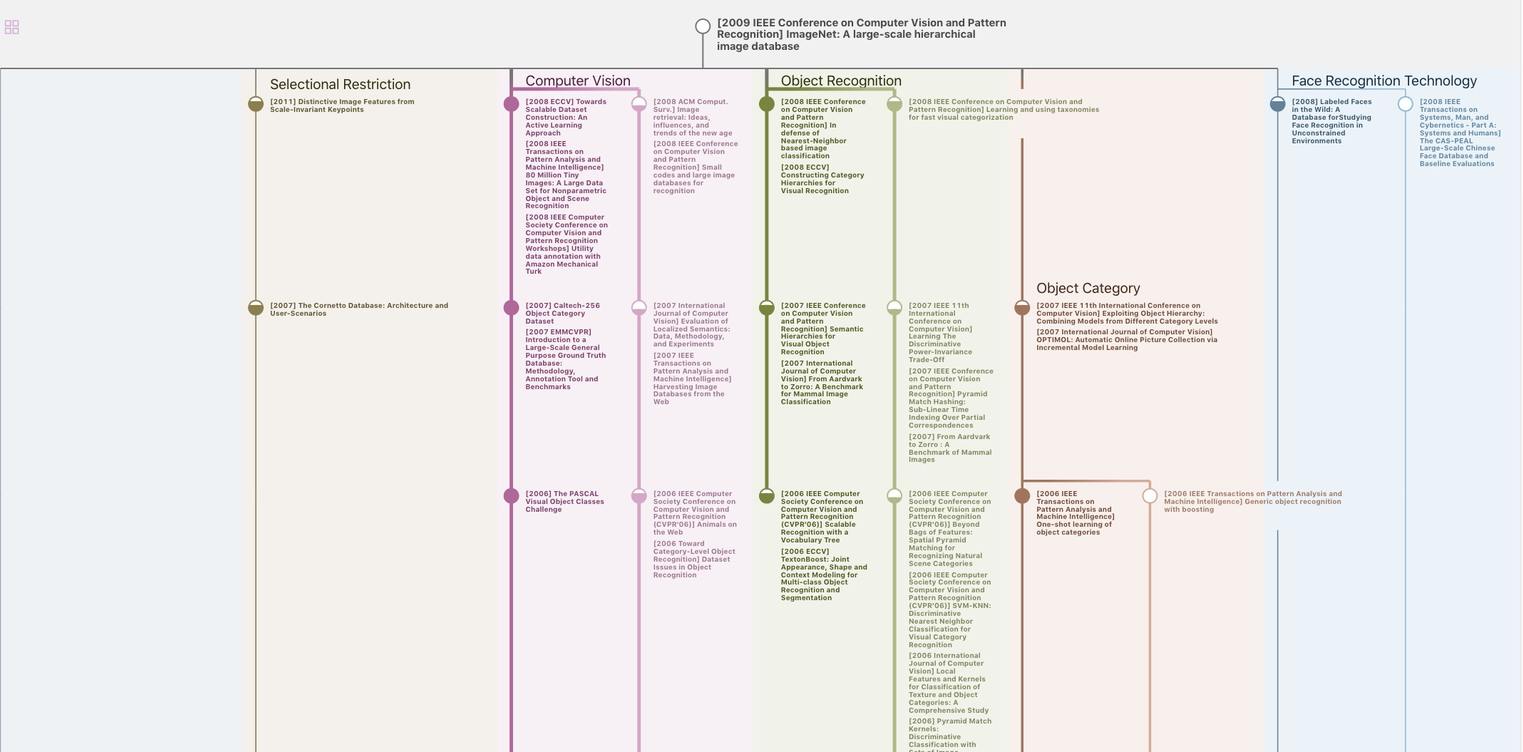
生成溯源树,研究论文发展脉络
Chat Paper
正在生成论文摘要