Evaluation of Multiclass Extraction of Representative Road Lines Found on Highway Pavement Using Supervised Semantic Segmentation Techniques and Aerial Imagery
2024 International Conference on Artificial Intelligence, Computer, Data Sciences and Applications (ACDSA)(2024)
摘要
Representative road lines (e.g., continuous and discontinuous lane markings, or lines defining highway entrances or exits) found on highway pavement represent valuable information for the generation of high-definition road cartography. A correct extraction of these road markings is becoming an increasingly important aspect in the context of accurate road decision support systems creation (used by autonomous driving solutions). In this work, the extraction of the mentioned representative road lines is tackled as a multiclass, supervised semantic segmentation task with deep learning methods. In this regard, thirteen semantic segmentation models (based on the state-of-the-art LinkNet, PSPNet (Pyramid Scene Parsing Network), FPN (Feature Pyramid Network), U-Net and U-Net++, and MA-Net (Multi-Attention-Network) architectures) were trained on a novel dataset (named "WhiteRoadLines") containing 27,025 images with a spatial resolution of 15 cm, labelled at pixel level. Post-training, the performance was evaluated using appropriate performance metrics; the best performing model being a version of U-Net coupled with Inception-ResNet-v2 that achieved a mean Intersection-over-Union score of 0.6559, while delivering high quality predictions on unseen, test data. The results proved the appropriateness of using DL techniques for completing high-definition road cartography with information related to road markings found on highways.
更多查看译文
关键词
road marking,semantic segmentation extraction,model evaluation
AI 理解论文
溯源树
样例
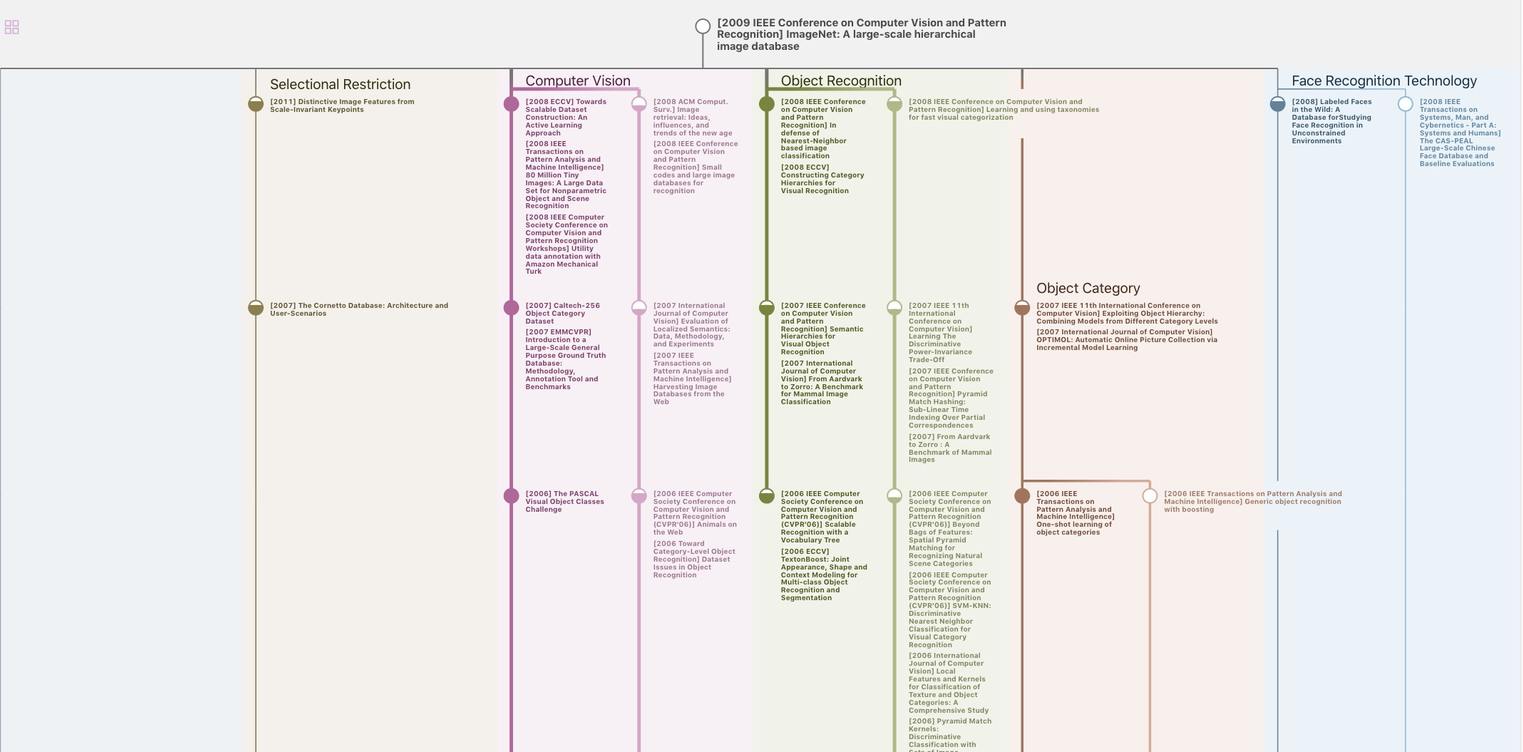
生成溯源树,研究论文发展脉络
Chat Paper
正在生成论文摘要