Toward Optimal Feature Selection: A Framework Harnessing Ensemble Metaheuristics
crossref(2024)
摘要
Abstract The increasing complexity of datasets in various domains necessitates more effective and efficient feature selection techniques for predictive analytics. This study presents an ensemble approach that integrates multiple metaheuristic algorithms to optimize feature selection across different datasets. Utilizing five datasets—NSL KDD, DARWIN, Period Changer, Toxicity, and SMK CAN 187—our experimental evaluation compared the ensemble of metaheuristic algorithms against individual algorithms in terms of both feature selection and predictive accuracy. Our results revealed that the ensemble approach significantly reduced the dimensionality of the feature space without sacrificing much in terms of predictive performance. For instance, on the NSL KDD dataset, the ensemble method averaged just 4.76% selected features with a competitive accuracy rate of 93.9%. Conversely, on the Period Changer dataset, the ensemble not only reduced the feature set but also outperformed individual algorithms with a higher average accuracy rate of 56.7%. These findings affirm the potential of ensembles of metaheuristics to offer a trade-off between computational efficiency and prediction accuracy, making them highly applicable in resource-constrained and real-world scenarios.
更多查看译文
AI 理解论文
溯源树
样例
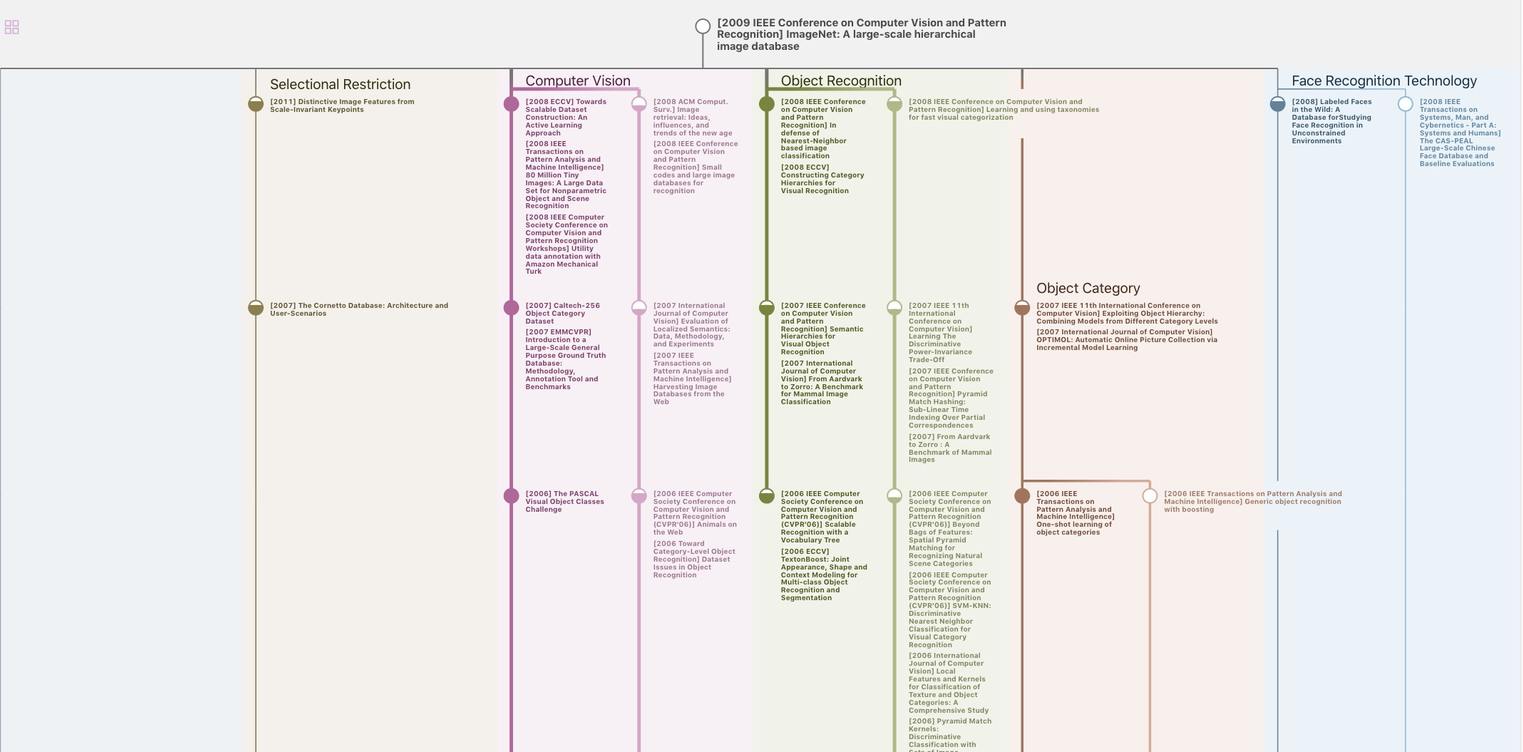
生成溯源树,研究论文发展脉络
Chat Paper
正在生成论文摘要