Hierarchical Multi-head Self-attention for Time-series-based Fault Diagnosis
Chinese Journal of Chemical Engineering(2024)
摘要
Fault diagnosis is important for maintaining the safety and effectiveness of chemical process. Considering the multivariate, nonlinear, and dynamic characteristic of chemical process, many time-series-based data-driven fault diagnosis methods have been developed in recent years. However, the existing methods have the problem of long-term dependency and is difficult to train due to the sequential way of training. To overcome these problems, a novel fault diagnosis method based on time-series and the hierarchical multi-head self-attention (HMSAN) is proposed for chemical process. First, a sliding window strategy is adopted to construct the normalized time-series dataset. Second, the HMSAN is developed to extract the time-relevant features from the time-series process data. It improves the basic self-attention model in both width and depth. With the multi-head structure, the HMSAN can pay attention to different aspects of the complicated chemical process and obtain the global dynamic features. However, the multiple heads in parallel lead to redundant information, which cannot improve the diagnosis performance. With the hierarchical structure, the redundant information is reduced and the deep local time-related features are further extracted. Besides, a novel many-to-one training strategy is introduced for HMSAN to simplify the training procedure and capture the long-term dependency. Finally, the effectiveness of the proposed method is demonstrated by two chemical cases. The experimental results show that the proposed method achieves a great performance on time-series industrial data and outperforms the state-of-art approaches.
更多查看译文
关键词
Self-attention mechanism,Deep learning,Chemical process,Time-series,Fault diagnosis
AI 理解论文
溯源树
样例
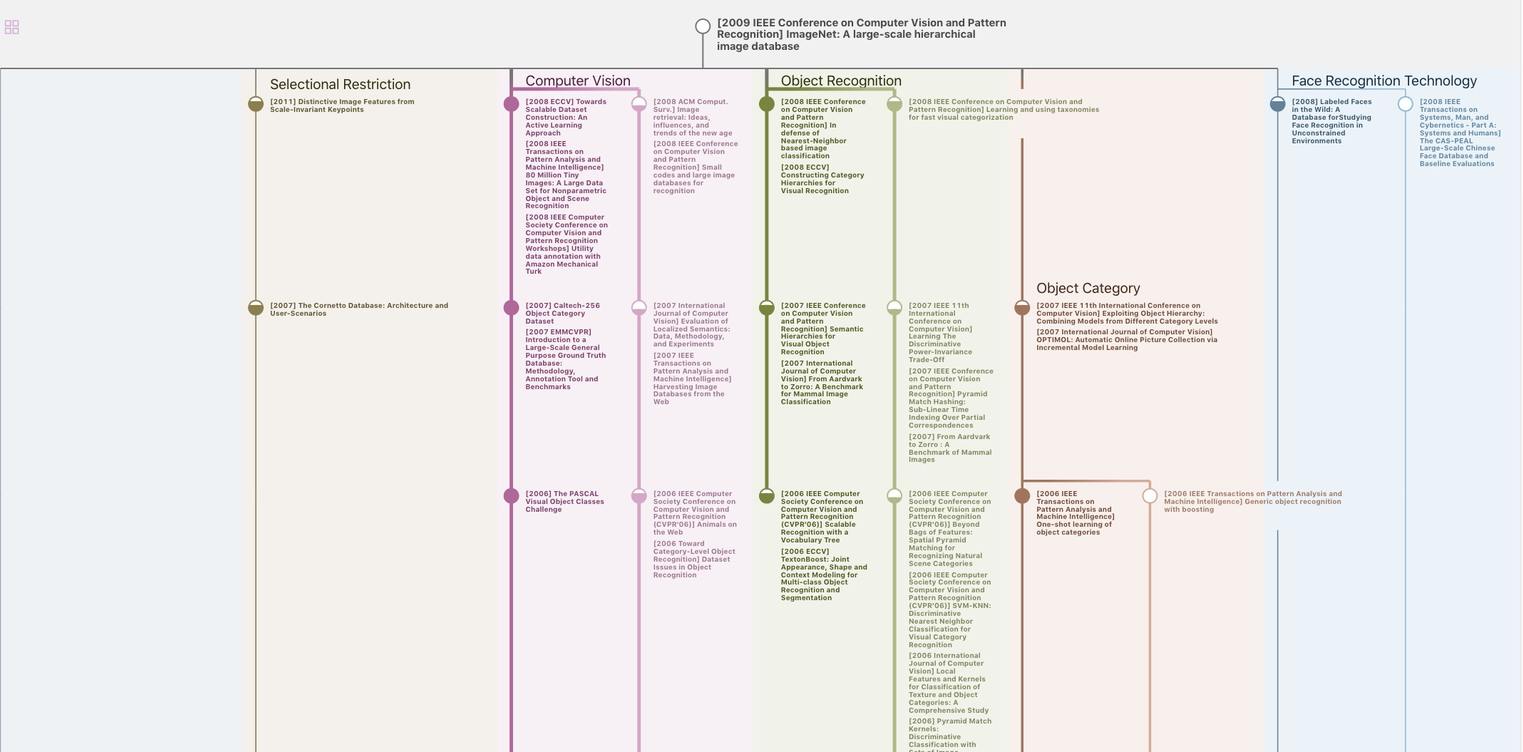
生成溯源树,研究论文发展脉络
Chat Paper
正在生成论文摘要