PPTtrack: Pyramid Pooling based Transformer backbone for visual tracking
Expert Systems with Applications(2024)
摘要
In visual tracking, Convolutional Neural Network (CNN) is usually used as feature extractor, and can fully explore local dependencies of image blocks, which is help for improving tracking performance. However, CNN ignores global dependencies in image blocks. The global modeling is crucial in visual tracking. Recently, Transformer has gained attention to fully explore global dependencies on sequential data. However, Transformer’s unique multi-head self-attention mechanism results in high computational complexity. In this paper, we design a pyramid pooling based Transformer backbone network for visual tracking. Pyramid pooling refers to multiple pooling operations for feature map with different receptive fields and strides. The output data of each pooling layer is concatenated to form the final pooled feature map. On the one hand, after flattening the feature map with pyramid pooling, its sequence length will be greatly reduced. This will effectively reduce the computational complexity of the multi-head self-attention. On the other hand, pyramid pooling can extract multi-scale features, makes the feature maps contain more global context information. Finally, we propose a novel tracker with the designed pyramid pooling based Transformer backbone network and the Transformer based model predictor. We train the proposed tracker by end-to-end, and evaluate it on seven tracking benchmarks including UAV123, NFS, Trackingnet, LaSOT, GOT-10K, VOT2020 and RGBT2019. The proposed tracker achieves 79.8% robustness and 35 FPS on the VOT2020 dataset. The experiment demonstrates that proposed tracker achieves superior tracking performance with state-of-the-art trackers.
更多查看译文
关键词
Visual tracking,Transformer,Convolutional neural network,Pyramid pooling
AI 理解论文
溯源树
样例
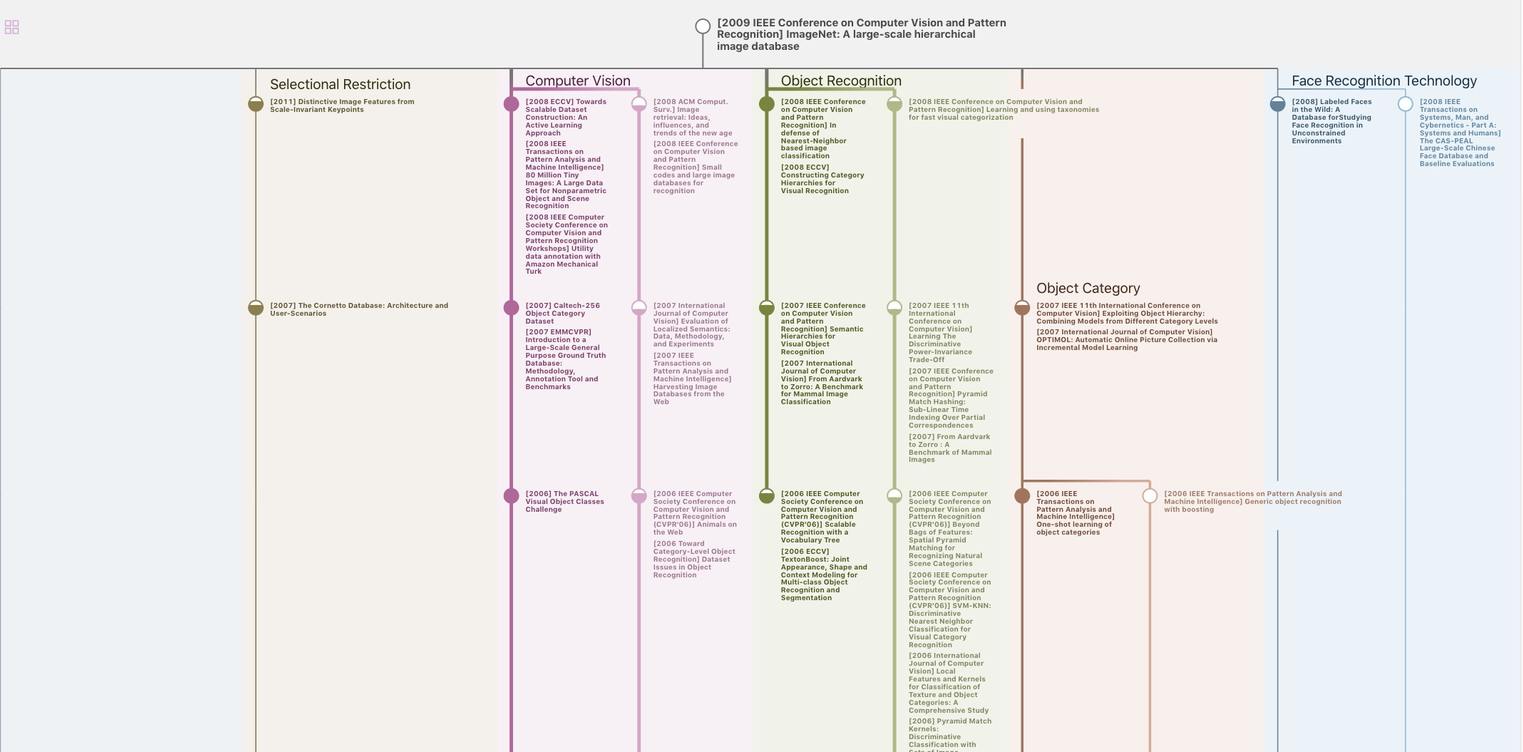
生成溯源树,研究论文发展脉络
Chat Paper
正在生成论文摘要