Intelligent Joint Actuator Fault Diagnosis for Heavy-duty Industrial Robots
IEEE Sensors Journal(2024)
摘要
A data-driven intelligent fault diagnosis algorithm is designed in this paper for heavy-duty industrial-robots (I-Rs), which aims accurately detecting and identifying joint actuator faults that may occur during the operation of industrial heavy-duty robot arms. Considering the fact that faulty samples of industrial robots are difficult to access, a simulation model-based strategy is adopted in this paper. With the help of Euler-Lagrange method, dynamics of heavy-duty industrial robots are established with considering the joint flexibility. By injecting fault in different joint actuators, unbalanced normal and faulty samples are obtained, based on which intelligent diagnosis model is constructed. Subsequently, a composite neural network model, LSTM-CNN, is proposed, which combines the merits of long short-term memory network (LSTM) and convolutional neural network (CNN). The constructed LSTM-CNN model is then trained and validated using generated data to achieve fault diagnosis and identification of actuators of heavy industrial robots. Finally, the constructed intelligent fault diagnosis model is experimentally validated, and result analysis demonstrates that the proposed algorithm shows superior accuracy and precision in diagnosing single joint and multiple joints faults.
更多查看译文
关键词
Intelligent fault diagnosis,Heavy-duty industrial robots,Actuator failures
AI 理解论文
溯源树
样例
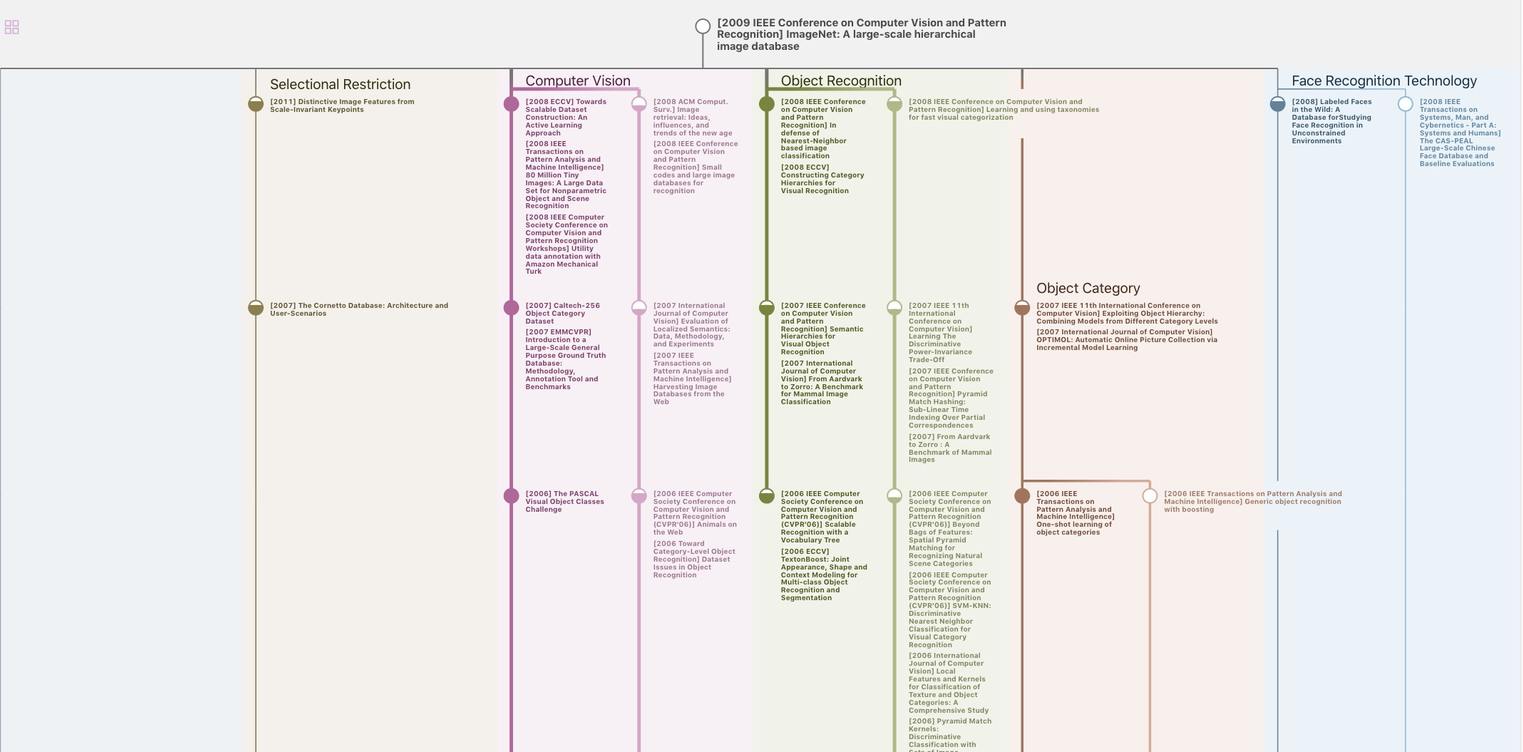
生成溯源树,研究论文发展脉络
Chat Paper
正在生成论文摘要