Machine learning methods applied to classify complex diseases using genomic data
crossref(2024)
摘要
Complex diseases pose challenges in disease prediction due to their multifactorial and polygenic nature. In this work, we explored the prediction of two complex diseases, multiple sclerosis (MS) and Alzheimer's disease (AD), using machine learning (ML) methods and genomic data from UK Biobank. Different ML methods were applied, including logistic regressions (LR), gradient boosting decision trees (GB), extremely randomized trees (ET), random forest (RF), feedforward networks (FFN), and convolutional neural networks (CNN). The primary goal of this research was to investigate the variability of ML models in classifying complex diseases based on genomic risk. LR was the most robust method across folds and diseases, whereas deep learning methods (FFN and CNN) exhibited high variability. When comparing the performance of polygenic risk scores (PRS) with ML methods, PRS consistently performed at an average level. However, PRS still offers several practical advantages over ML methods. Despite implementing feature selection techniques to exclude non-informative and correlated predictors, the performance of ML models did not improve significantly, underscoring the ability of ML methods to achieve optimal performance even in the presence of correlated features due to linkage disequilibrium. Upon applying explainability tools to extract information about the genomic features contributing most to the classification task, the results confirmed the polygenicity of MS. The prevalence of HLA gene annotations among the top genomic features on chromosome 6 aligns with their significance in the context of MS. Overall, the highest-prioritized genomic variants were identified as expression or splicing quantitative trait loci (eQTL or sQTL) located in non-coding regions within or near genes associated with the immune response and MS. In summary, this research offers deeper insights into how ML models discern genomic patterns related to complex diseases.
### Competing Interest Statement
The authors have declared no competing interest.
更多查看译文
AI 理解论文
溯源树
样例
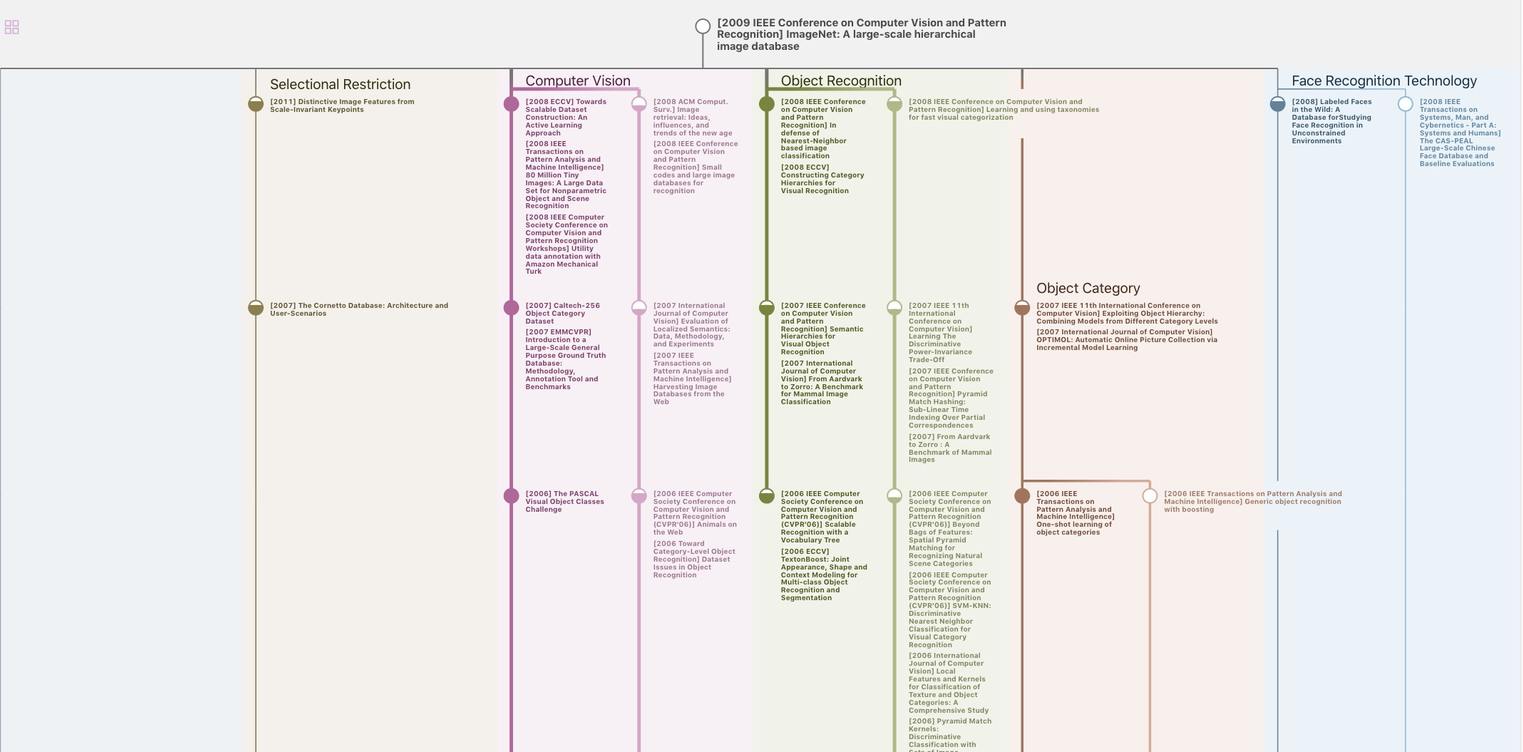
生成溯源树,研究论文发展脉络
Chat Paper
正在生成论文摘要