Mitigating Bias in Aesthetic Quality Control Tasks: An Adversarial Learning Approach
Procedia Computer Science(2024)
摘要
Aesthetic quality control (AQC) is an essential step in smart factories to ensure that product quality meets the desired standards. This operation includes assessing factors such as color, texture, and shape. In the context of AQC, bias can arise when the criteria used to evaluate the aesthetics of a product are subjective and influenced by personal preferences. Bias can also occur due to the background or other objective factors like the geometry of the material. This work will focus on applying an adversarial learning strategy to a pre-trained DL architecture for improving the generalization performance of a predictive model tailored explicitly for solving AQC task classification. Experimental results on a benchmark AQC dataset highlighted the robustness of the proposed methodology for learning only relevant components related to quality classes rather than other confusing traits, enabling the mitigation of the identified bias.
更多查看译文
关键词
Adversarial learning,Bias mitigation,Aesthetic quality control,Industry 4.0
AI 理解论文
溯源树
样例
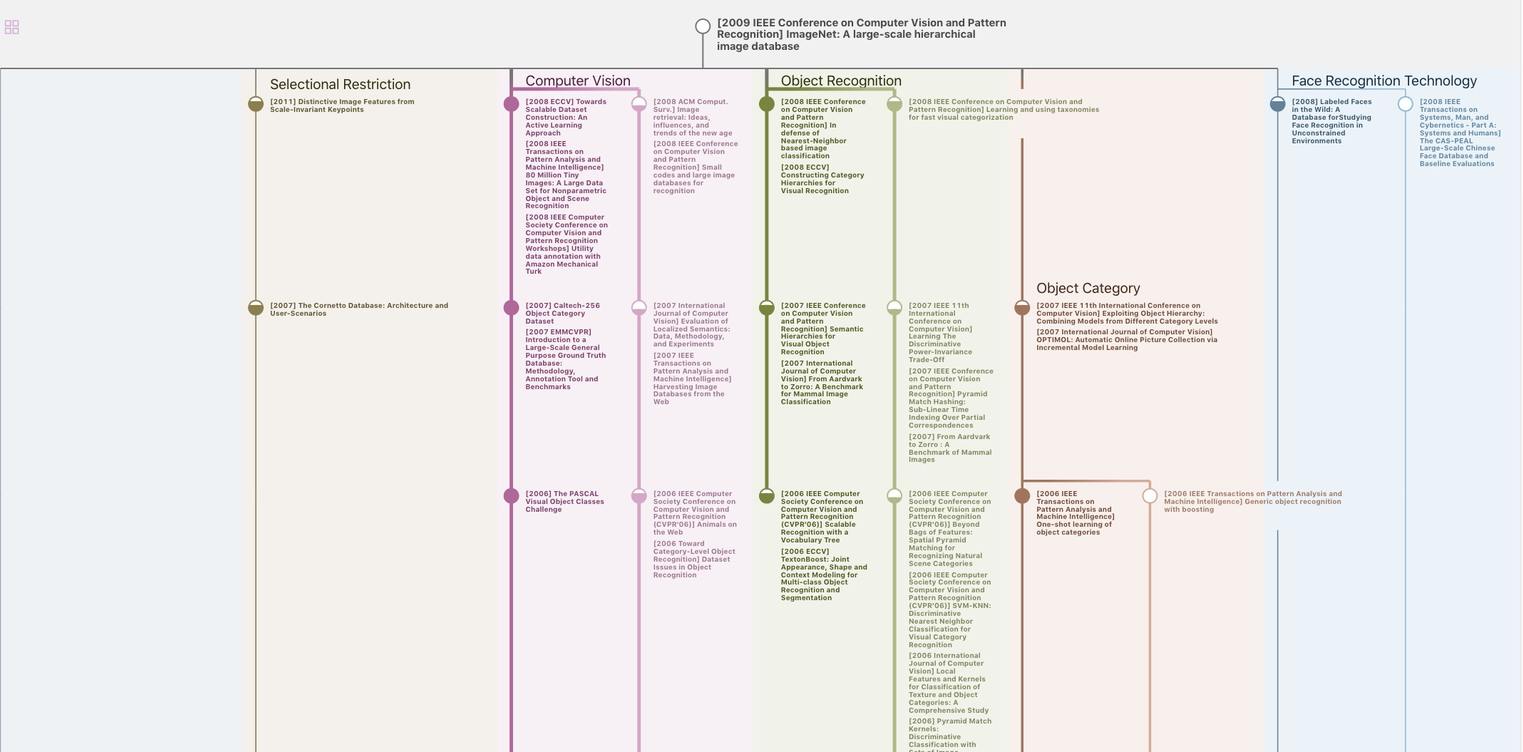
生成溯源树,研究论文发展脉络
Chat Paper
正在生成论文摘要